Task-Oriented Cross-System Design for Timely and Accurate Modeling in the Metaverse
IEEE JOURNAL ON SELECTED AREAS IN COMMUNICATIONS(2024)
摘要
In this paper, we establish a task-oriented cross-system design framework to minimize the required packet rate for timely and accurate modeling of a real-world robotic arm in the Metaverse, where sensing, communication, prediction, control, and rendering are considered. To optimize a scheduling policy and prediction horizons, we design a Constraint Proximal Policy Optimization (C-PPO) algorithm by integrating domain knowledge from relevant systems into the advanced reinforcement learning algorithm, Proximal Policy Optimization (PPO). Specifically, the Jacobian matrix for analyzing the motion of the robotic arm is included in the state of the C-PPO algorithm, and the Conditional Value-at-Risk (CVaR) of the state-value function characterizing the long-term modeling error is adopted in the constraint. Besides, the policy is represented by a two-branch neural network determining the scheduling policy and the prediction horizons, respectively. To evaluate our algorithm, we build a prototype including a real-world robotic arm and its digital model in the Metaverse. The experimental results indicate that domain knowledge helps to reduce the convergence time and the required packet rate by up to 50%, and the cross-system design framework outperforms a baseline framework in terms of the required packet rate and the tail distribution of the modeling error.
更多查看译文
关键词
Metaverse,Task analysis,5G mobile communication,Computational modeling,Servers,Manipulators,Digital twins,Task-oriented cross-system design,scheduling,prediction,constraint deep reinforcement learning
AI 理解论文
溯源树
样例
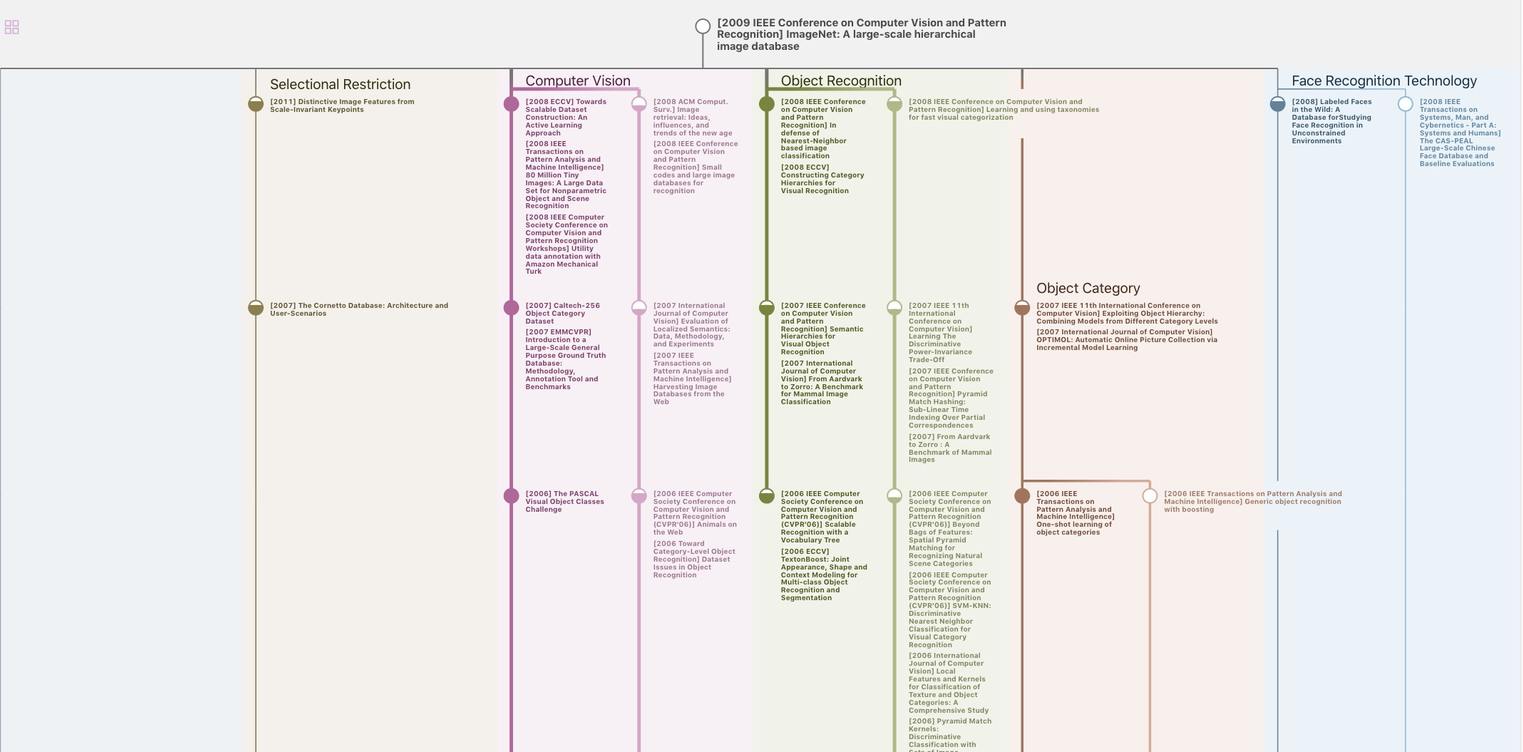
生成溯源树,研究论文发展脉络
Chat Paper
正在生成论文摘要