Towards Better Data Exploitation in Self-Supervised Monocular Depth Estimation
IEEE ROBOTICS AND AUTOMATION LETTERS(2024)
摘要
Depth estimation plays an important role in robotic perception systems. The self-supervised monocular paradigm has gained significant attention since it can free training from the reliance on depth annotations. Despite recent advancements, existing self-supervised methods still underutilize the available training data, limiting their generalization ability. In this letter, we take two data augmentation techniques, namely Resizing-Cropping and Splitting-Permuting, to fully exploit the potential of training datasets. Specifically, the original image and the generated two augmented images are fed into the training pipeline simultaneously and we leverage them to conduct self-distillation. Additionally, we introduce the detail-enhanced DepthNet with an extra full-scale branch in the encoder and a grid decoder to enhance the restoration of fine details in depth maps. Experimental results demonstrate our method can achieve state-of-the-art performance on the KITTI and Cityscapes datasets. Moreover, our KITTI models also show superior generalization performance when transferring to Make3D, NYUv2 and Cityscapes datasets.
更多查看译文
关键词
Deep learning for visual perception,deep learning methods,visual Learning
AI 理解论文
溯源树
样例
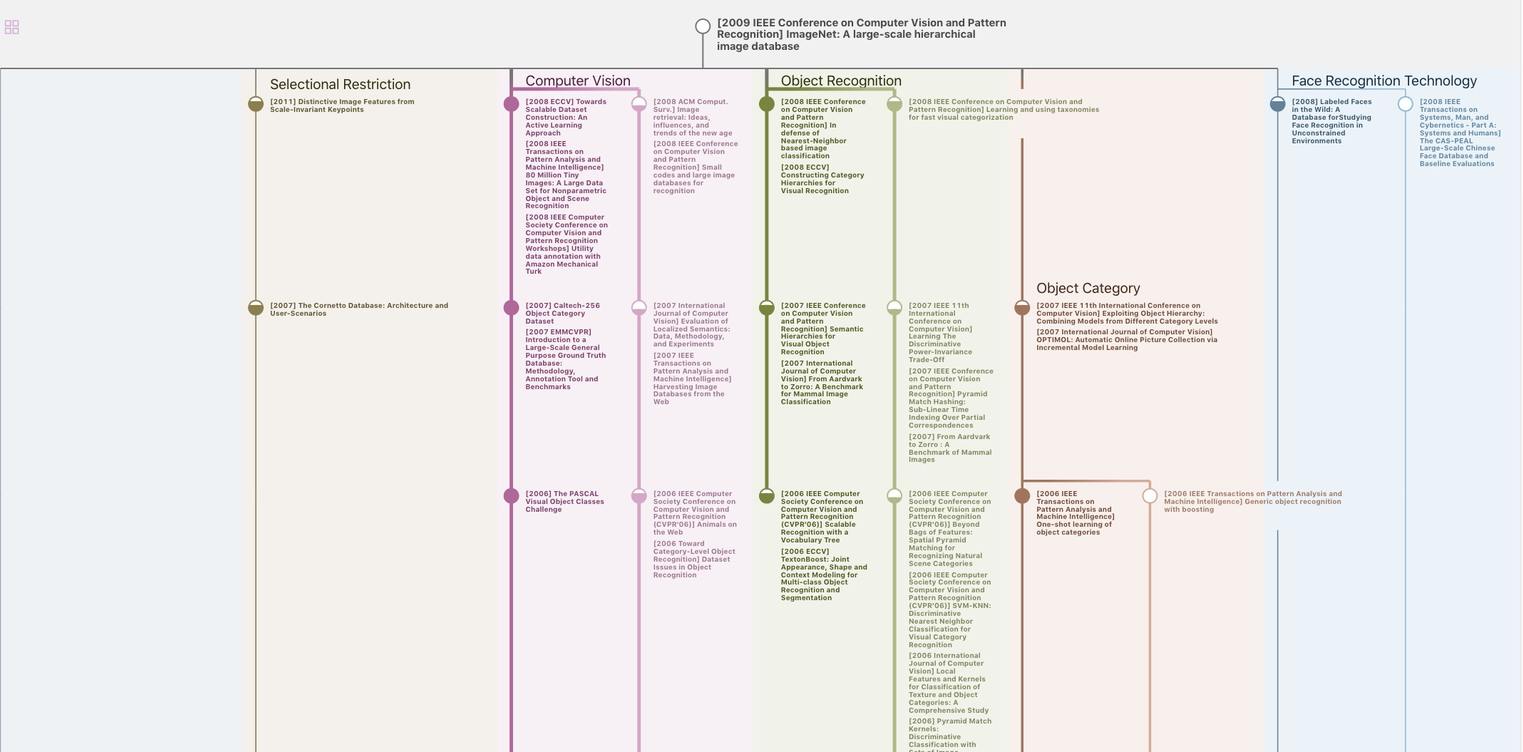
生成溯源树,研究论文发展脉络
Chat Paper
正在生成论文摘要