MFPNet: Multi-scale Feature Propagation Nwtwork For Lightweight Semantic Segmentation
arxiv(2023)
摘要
In contrast to the abundant research focusing on large-scale models, the progress in lightweight semantic segmentation appears to be advancing at a comparatively slower pace. However, existing compact methods often suffer from limited feature representation capability due to the shallowness of their networks. In this paper, we propose a novel lightweight segmentation architecture, called Multi-scale Feature Propagation Network (MFPNet), to address the dilemma. Specifically, we design a robust Encoder-Decoder structure featuring symmetrical residual blocks that consist of flexible bottleneck residual modules (BRMs) to explore deep and rich muti-scale semantic context. Furthermore, taking benefit from their capacity to model latent long-range contextual relationships, we leverage Graph Convolutional Networks (GCNs) to facilitate multi-scale feature propagation between the BRM blocks. When evaluated on benchmark datasets, our proposed approach shows superior segmentation results.
更多查看译文
AI 理解论文
溯源树
样例
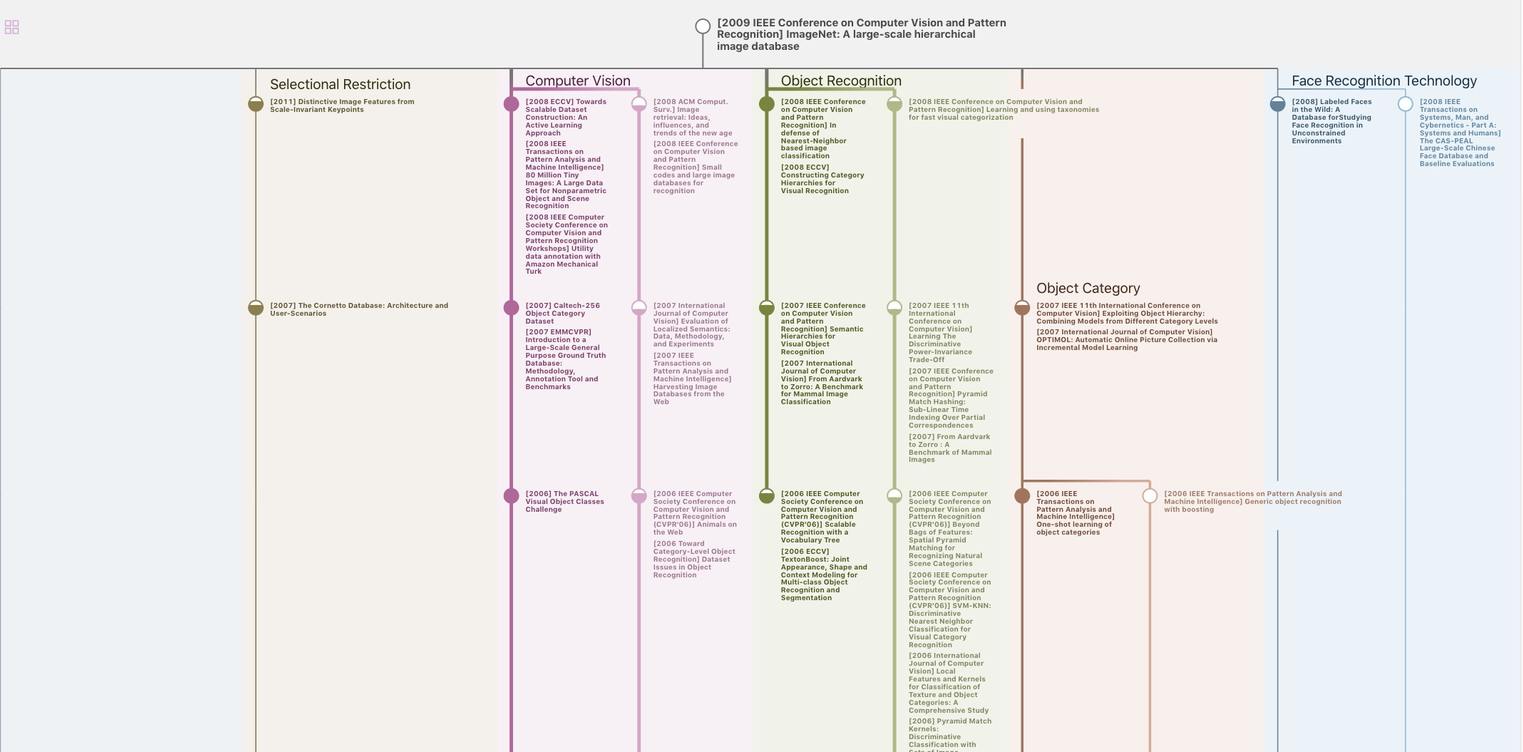
生成溯源树,研究论文发展脉络
Chat Paper
正在生成论文摘要