Flexible and Robust Counterfactual Explanations with Minimal Satisfiable Perturbations
PROCEEDINGS OF THE 32ND ACM INTERNATIONAL CONFERENCE ON INFORMATION AND KNOWLEDGE MANAGEMENT, CIKM 2023(2023)
摘要
Counterfactual explanations (CFEs) exemplify how to minimally modify a feature vector to achieve a different prediction for an instance. CFEs can enhance informational fairness and trustworthiness, and provide suggestions for users who receive adverse predictions. However, recent research has shown that multiple CFEs can be offered for the same instance or instances with slight differences. Multiple CFEs provide flexible choices and cover diverse desiderata for user selection. However, individual fairness and model reliability will be damaged if unstable CFEs with different costs are returned. Existing methods fail to exploit flexibility and address the concerns of non-robustness simultaneously. To address these issues, we propose a conceptually simple yet effective solution named Counterfactual Explanations with Minimal Satisfiable Perturbations (CEMSP). Specifically, CEMSP constrains changing values of abnormal features with the help of their semantically meaningful normal ranges. For efficiency, we model the problem as a Boolean satisfiability problem to modify as few features as possible. Additionally, CEMSP is a general framework and can easily accommodate more practical requirements, e.g., casualty and actionability. Compared to existing methods, we conduct comprehensive experiments on both synthetic and real-world datasets to demonstrate that our method provides more robust explanations while preserving flexibility.
更多查看译文
关键词
Counterfactual explanations,multiplicity,normal ranges,flexibility,robustness
AI 理解论文
溯源树
样例
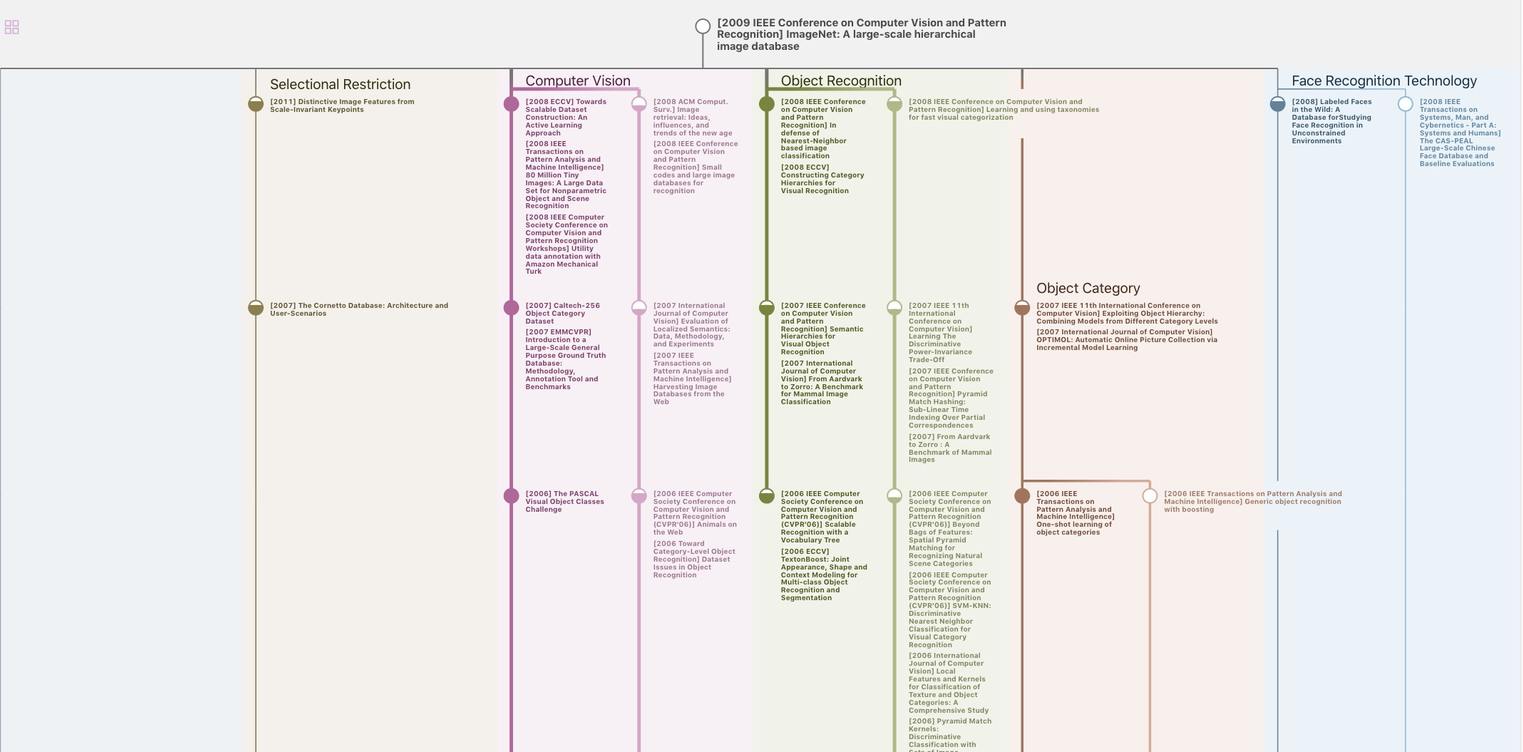
生成溯源树,研究论文发展脉络
Chat Paper
正在生成论文摘要