SSHNN: Semi-Supervised Hybrid NAS Network for Echocardiographic Image Segmentation
ICASSP 2024 - 2024 IEEE International Conference on Acoustics, Speech and Signal Processing (ICASSP)(2023)
Abstract
Accurate medical image segmentation especially for echocardiographic images with unmissable noise requires elaborate network design. Compared with manual design, Neural Architecture Search (NAS) realizes better segmentation results due to larger search space and automatic optimization, but most of the existing methods are weak in layer-wise feature aggregation and adopt a ``strong encoder, weak decoder" structure, insufficient to handle global relationships and local details. To resolve these issues, we propose a novel semi-supervised hybrid NAS network for accurate medical image segmentation termed SSHNN. In SSHNN, we creatively use convolution operation in layer-wise feature fusion instead of normalized scalars to avoid losing details, making NAS a stronger encoder. Moreover, Transformers are introduced for the compensation of global context and U-shaped decoder is designed to efficiently connect global context with local features. Specifically, we implement a semi-supervised algorithm Mean-Teacher to overcome the limited volume problem of labeled medical image dataset. Extensive experiments on CAMUS echocardiography dataset demonstrate that SSHNN outperforms state-of-the-art approaches and realizes accurate segmentation. Code will be made publicly available.
MoreTranslated text
Key words
echocardiographic image,segmentation,network,semi-supervised
AI Read Science
Must-Reading Tree
Example
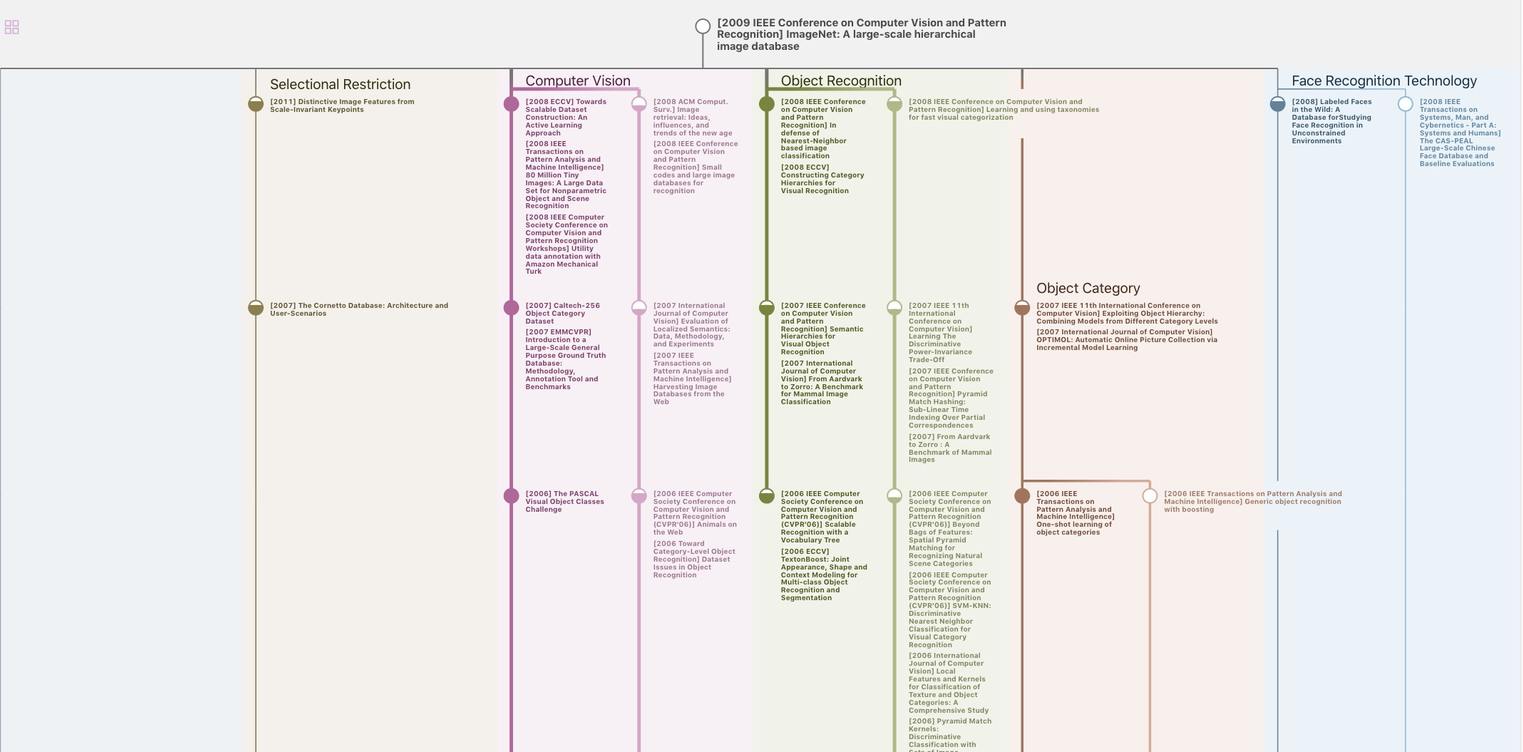
Generate MRT to find the research sequence of this paper
Chat Paper
Summary is being generated by the instructions you defined