Retrieving Evidence from EHRs with LLMs: Possibilities and Challenges
CoRR(2023)
摘要
Unstructured Electronic Health Record (EHR) data often contains critical information complementary to imaging data that would inform radiologists' diagnoses. However, time constraints and the large volume of notes frequently associated with individual patients renders manual perusal of such data to identify relevant evidence infeasible in practice. Modern Large Language Models (LLMs) provide a flexible means of interacting with unstructured EHR data, and may provide a mechanism to efficiently retrieve and summarize unstructured evidence relevant to a given query. In this work, we propose and evaluate an LLM (Flan-T5 XXL) for this purpose. Specifically, in a zero-shot setting we task the LLM to infer whether a patient has or is at risk of a particular condition; if so, we prompt the model to summarize the supporting evidence. Enlisting radiologists for manual evaluation, we find that this LLM-based approach provides outputs consistently preferred to a standard information retrieval baseline, but we also highlight the key outstanding challenge: LLMs are prone to hallucinating evidence. However, we provide results indicating that model confidence in outputs might indicate when LLMs are hallucinating, potentially providing a means to address this.
更多查看译文
关键词
llms,ehrs,evidence
AI 理解论文
溯源树
样例
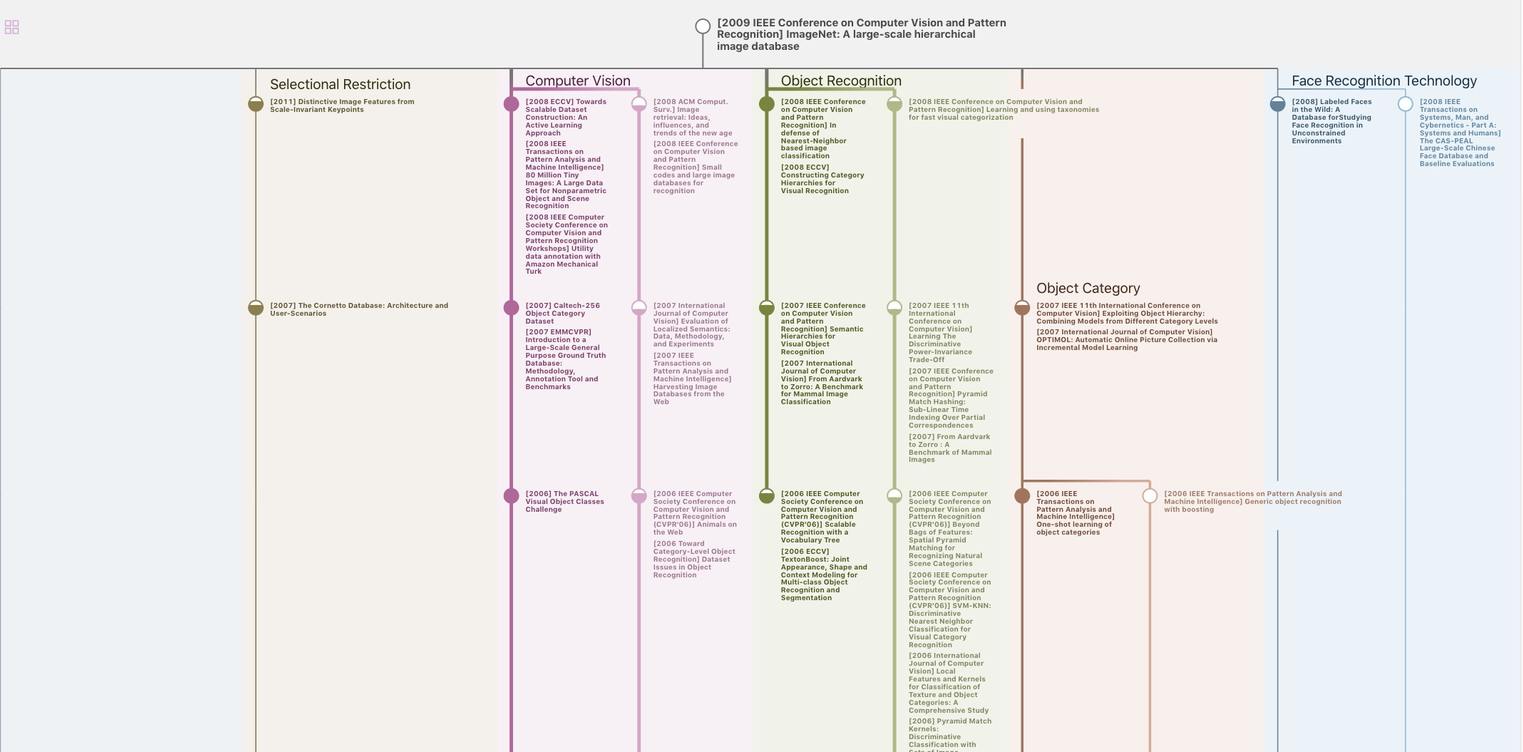
生成溯源树,研究论文发展脉络
Chat Paper
正在生成论文摘要