Compositional Learning of Visually-Grounded Concepts Using Reinforcement
CoRR(2023)
摘要
Deep reinforcement learning agents need to be trained over millions of episodes to decently solve navigation tasks grounded to instructions. Furthermore, their ability to generalize to novel combinations of instructions is unclear. Interestingly however, children can decompose language-based instructions and navigate to the referred object, even if they have not seen the combination of queries prior. Hence, we created three 3D environments to investigate how deep RL agents learn and compose color-shape based combinatorial instructions to solve novel combinations in a spatial navigation task. First, we explore if agents can perform compositional learning, and whether they can leverage on frozen text encoders (e.g. CLIP, BERT) to learn word combinations in fewer episodes. Next, we demonstrate that when agents are pretrained on the shape or color concepts separately, they show a 20 times decrease in training episodes needed to solve unseen combinations of instructions. Lastly, we show that agents pretrained on concept and compositional learning achieve significantly higher reward when evaluated zero-shot on novel color-shape1-shape2 visual object combinations. Overall, our results highlight the foundations needed to increase an agent's proficiency in composing word groups through reinforcement learning and its ability for zero-shot generalization to new combinations.
更多查看译文
关键词
learning,concepts,visually-grounded
AI 理解论文
溯源树
样例
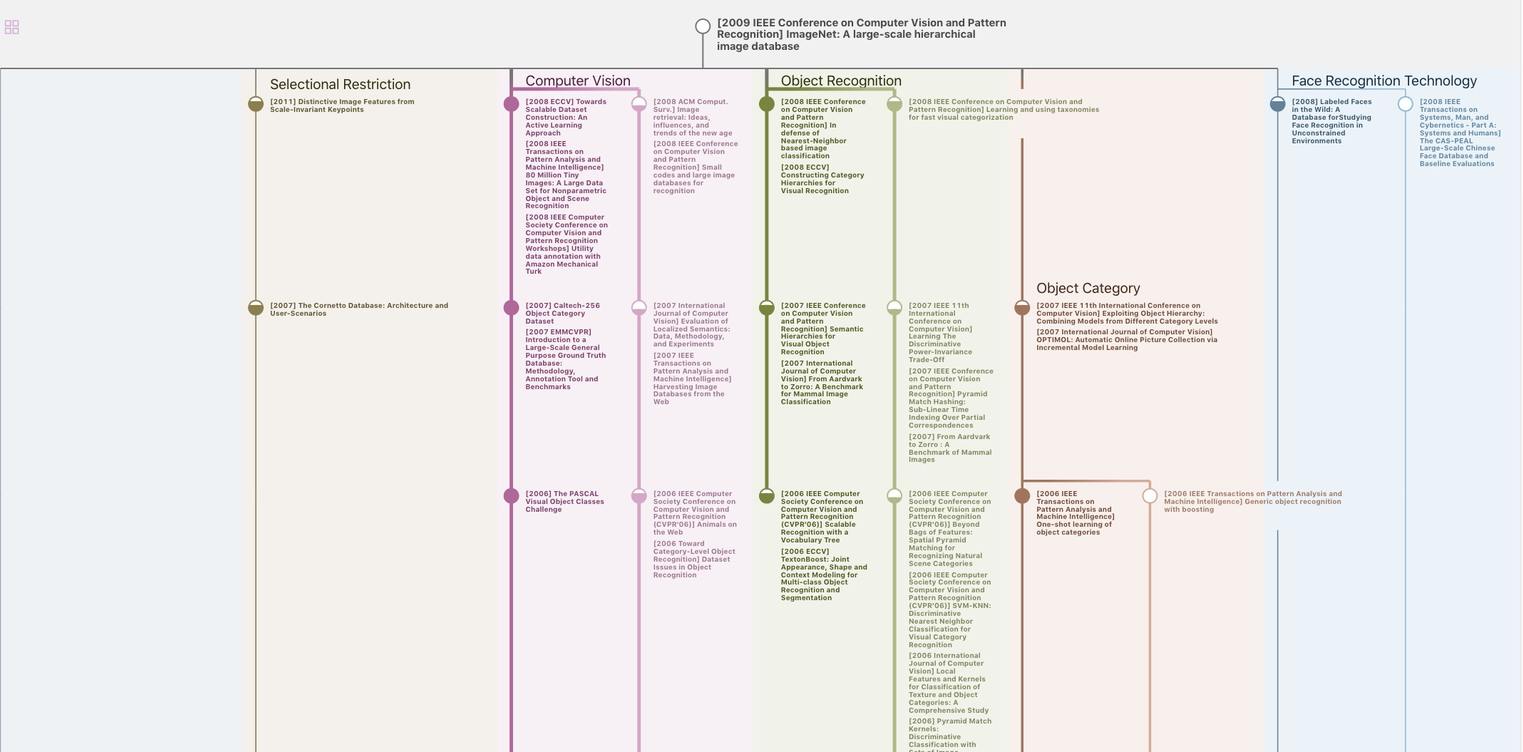
生成溯源树,研究论文发展脉络
Chat Paper
正在生成论文摘要