Multimodal machine learning for materials science: composition-structure bimodal learning for experimentally measured properties
CoRR(2023)
摘要
The widespread application of multimodal machine learning models like GPT-4 has revolutionized various research fields including computer vision and natural language processing. However, its implementation in materials informatics remains underexplored, despite the presence of materials data across diverse modalities, such as composition and structure. The effectiveness of machine learning models trained on large calculated datasets depends on the accuracy of calculations, while experimental datasets often have limited data availability and incomplete information. This paper introduces a novel approach to multimodal machine learning in materials science via composition-structure bimodal learning. The proposed COmposition-Structure Bimodal Network (COSNet) is designed to enhance learning and predictions of experimentally measured materials properties that have incomplete structure information. Bimodal learning significantly reduces prediction errors across distinct materials properties including Li conductivity in solid electrolyte, band gap, refractive index, dielectric constant, energy, and magnetic moment, surpassing composition-only learning methods. Furthermore, we identified that data augmentation based on modal availability plays a pivotal role in the success of bimodal learning.
更多查看译文
关键词
bimodal learning,multimodal machine,materials science,composition-structure
AI 理解论文
溯源树
样例
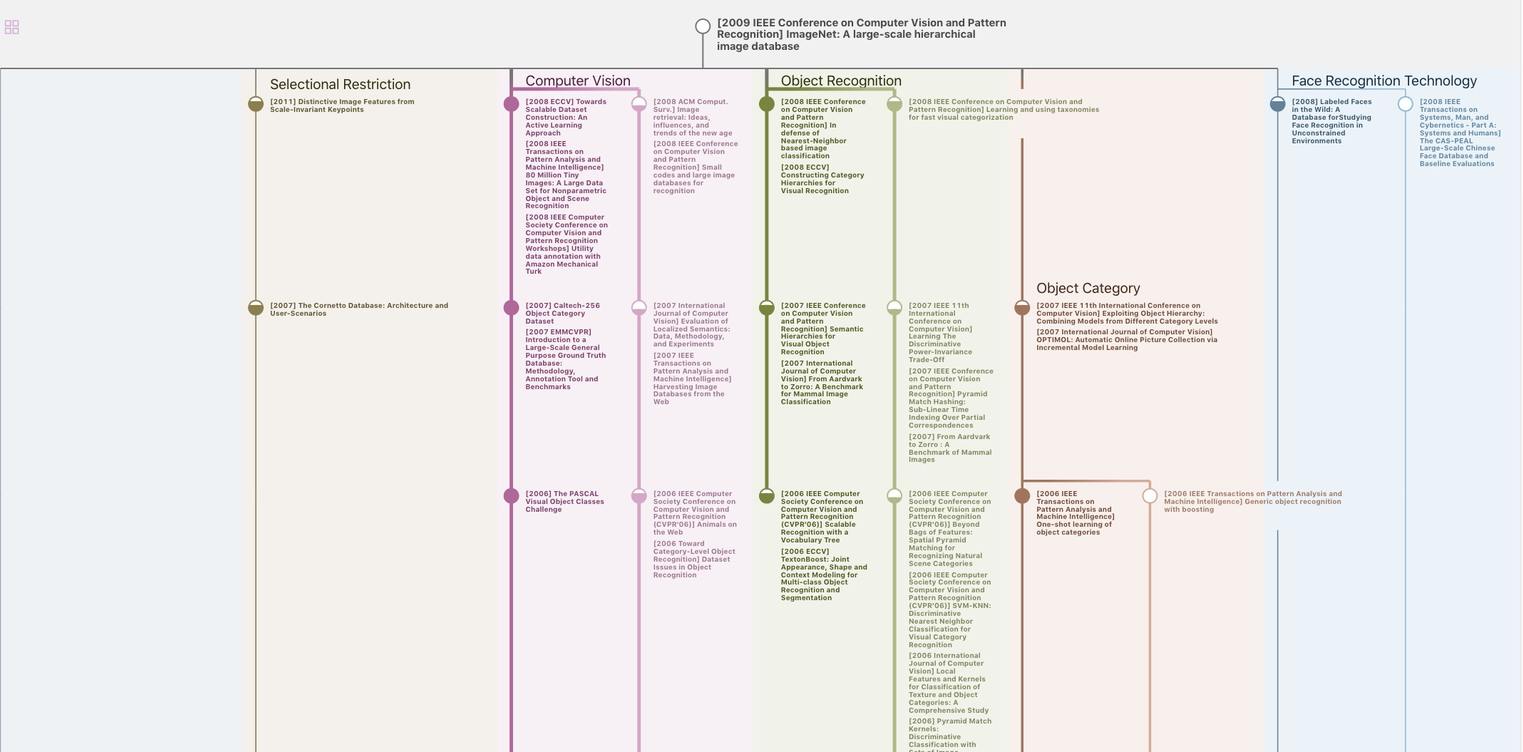
生成溯源树,研究论文发展脉络
Chat Paper
正在生成论文摘要