Machine Learning Study of SNPs in Noncoding Regions to Predict Non-small Cell Lung Cancer Susceptibility.
Clinical oncology (Royal College of Radiologists (Great Britain))(2023)
Abstract
Non-small cell lung cancer (NSCLC) is the most common pathological subtype of lung cancer. Both environmental and genetic factors have been reported to impact the lung cancer susceptibility. We conducted a genome-wide association study (GWAS) of 287 NSCLC patients and 467 healthy controls in a Chinese population using the Illumina Genome-Wide Asian Screening Array Chip on 712,095 SNPs (single nucleotide polymorphisms). Using logistic regression modeling, GWAS identified 17 new noncoding region SNP loci associated with the NSCLC risk, and the top three (rs80040741, rs9568547, rs6010259) were under a stringent p-value (<3.02e-6). Notably, rs80040741 and rs6010259 were annotated from the intron regions of MUC3A and MLC1, respectively. Together with another five SNPs previously reported in Chinese NSCLC patients and another four covariates (e.g., smoking status, age, low dose CT screening, sex), a predictive model by machine learning methods can separate the NSCLC from healthy controls with an accuracy of 86%. This is the first time to apply machine learning method in predicting the NSCLC susceptibility using both genetic and clinical characteristics. Our findings will provide a promising method in NSCLC early diagnosis and improve our understanding of applying machine learning methods in precision medicine.
MoreTranslated text
AI Read Science
Must-Reading Tree
Example
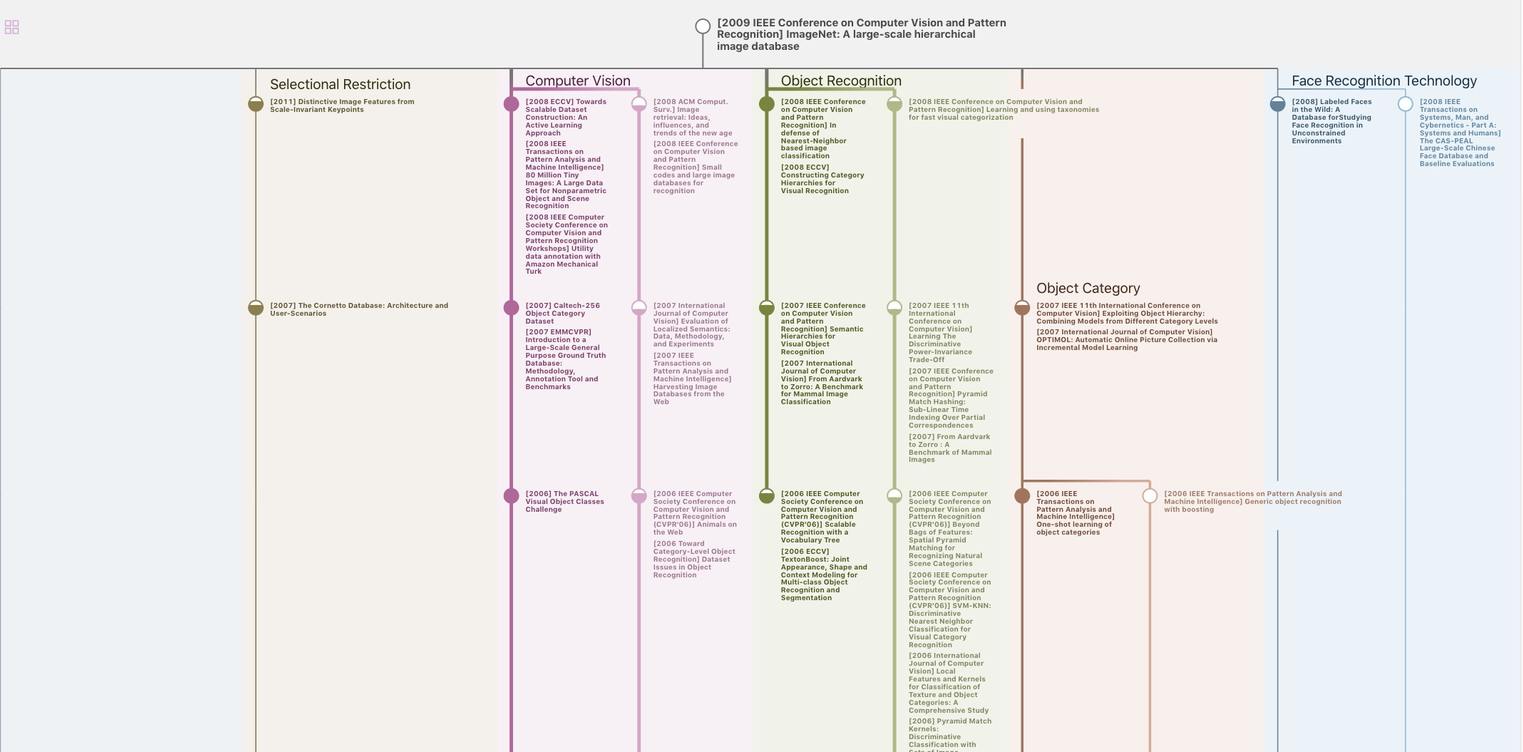
Generate MRT to find the research sequence of this paper
Chat Paper
Summary is being generated by the instructions you defined