Riemannian Anderson Mixing Methods for Minimizing $C^2$-Functions on Riemannian Manifolds
arXiv (Cornell University)(2023)
摘要
The Anderson Mixing (AM) method is a popular approach for accelerating fixed-point iterations by leveraging historical information from previous steps. In this paper, we introduce the Riemannian Anderson Mixing (RAM) method, an extension of AM to Riemannian manifolds, and analyze its local linear convergence under reasonable assumptions. Unlike other extrapolation-based algorithms on Riemannian manifolds, RAM does not require computing the inverse retraction or inverse exponential mapping and has a lower per-iteration cost. Furthermore, we propose a variant of RAM called Regularized RAM (RRAM), which establishes global convergence and exhibits similar local convergence properties as RAM. Our proof relies on careful error estimations based on the local geometry of Riemannian manifolds. Finally, we present experimental results on various manifold optimization problems that demonstrate the superior performance of our proposed methods over existing Riemannian gradient descent and LBFGS approaches.
更多查看译文
关键词
riemannian anderson mixing methods,riemannian manifolds
AI 理解论文
溯源树
样例
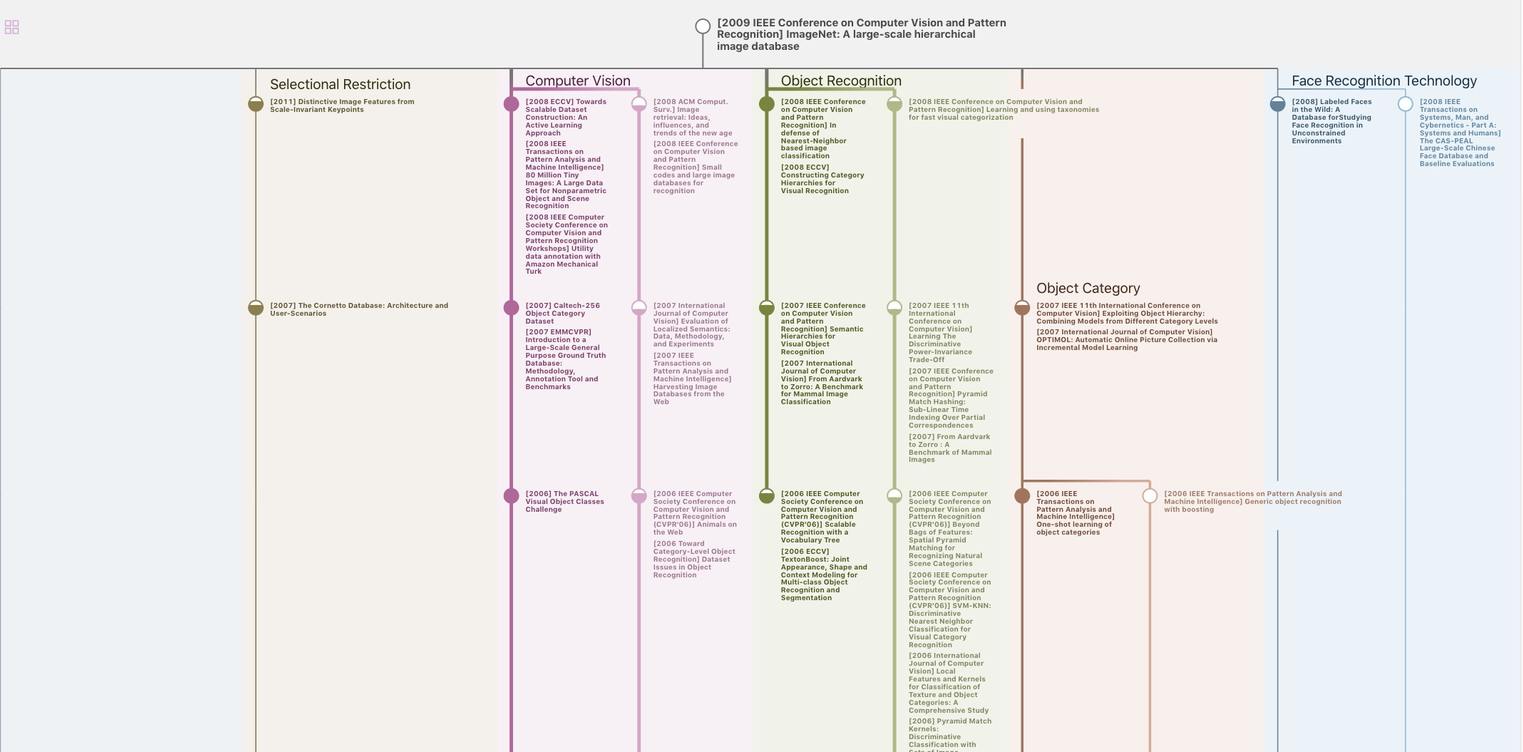
生成溯源树,研究论文发展脉络
Chat Paper
正在生成论文摘要