AdaBoost-Bagging deep inverse reinforcement learning for autonomous taxi cruising route and speed planning
Transportation Research Part E: Logistics and Transportation Review(2023)
摘要
Taxi cruising route planning has attracted considerable attention, and relevant studies can be broadly categorized into three main streams: recommending one or multiple areas, providing a detailed cruising route, and deriving the optimal routing policy. However, these studies depend on accurate pick-up/drop-off information, and seldom pay attention to cruising speed planning. In view of the rapid development of autonomous taxis, this study proposes AdaBoost-Bagging maximum entropy deep inverse reinforcement learning to learn cruising policy from experienced taxi drivers’ trajectories. Moreover, we develop a trajectory-based self-attention bidirectional LSTM model to adjust cruising speeds on different roads. Numerical experiments using real taxi trajectories in Chengdu, China demonstrate the effectiveness of our approach in learning taxi drivers’ policies and improving taxis’ operational efficiency.
更多查看译文
关键词
deep inverse reinforcement learning,autonomous taxi,adaboost-bagging
AI 理解论文
溯源树
样例
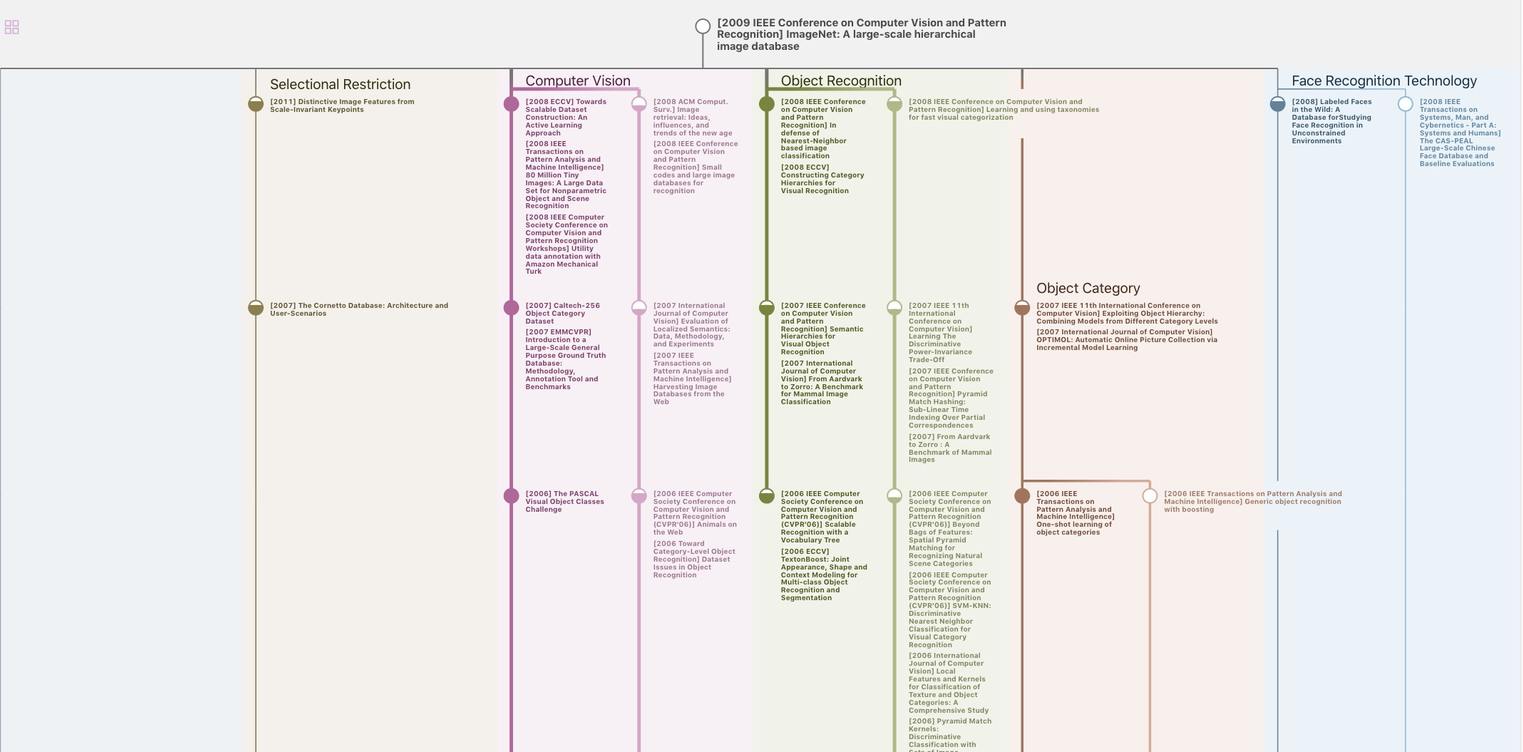
生成溯源树,研究论文发展脉络
Chat Paper
正在生成论文摘要