Tensor based low rank representation of hyperspectral images for wheat seeds varieties identification
Computers and Electrical Engineering(2023)
Abstract
Hyperspectral image (HSI) based methods are widely used in identifying seeds varieties with high accuracy. However, the excessive spectral bands in HSI may contain redundant information and degrade the model performance. To address this challenge, we propose a novel feature extraction method called low rank tensor approximation (LRTA). Unlike traditional methods, LRTA extracts joint discriminative information of all wheat seeds from hyperspectral images in a 3-order tensor form, preserving intrinsic information. Our model has three key steps: extracting the region of interest from hyperspectral images and presenting average spectral information in tensor form, using LRTA to extract jointly discriminative information, and feeding this information into a classifier to identify seeds varieties. Experiments on our proposed dataset show that the proposed method has 4% improved to the conventional methods on average. Our method shows promise for improving the accuracy of seeds identification while reducing the dimensionality of HSI.
MoreTranslated text
Key words
hyperspectral images,low rank representation,wheat
AI Read Science
Must-Reading Tree
Example
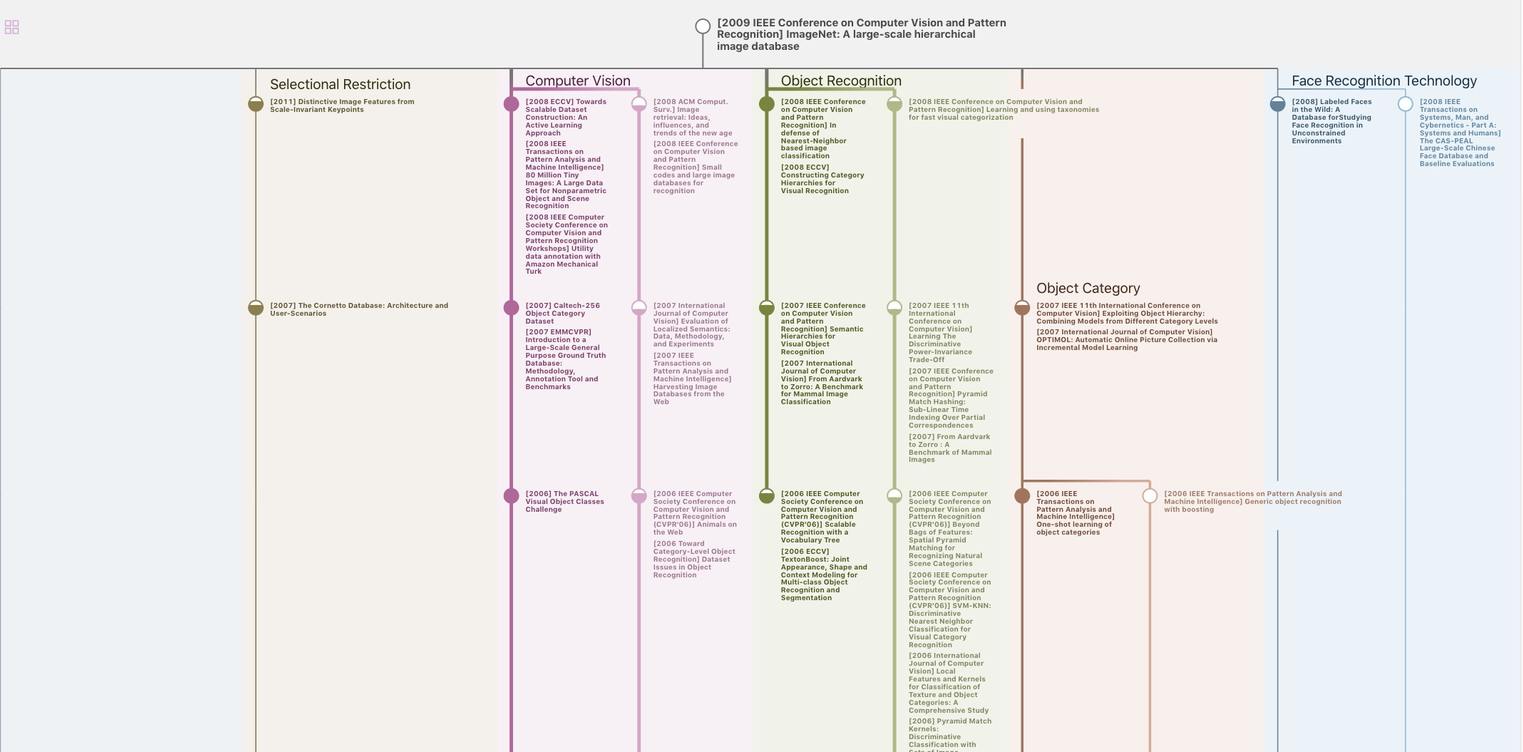
Generate MRT to find the research sequence of this paper
Chat Paper
Summary is being generated by the instructions you defined