A novel wind power prediction model improved with feature enhancement and autoregressive error compensation
JOURNAL OF CLEANER PRODUCTION(2023)
Abstract
Wind energy is a widely utilized form of clean energy with significant implications for maximizing its utilization and ensuring the stability of power systems. However, existing methods for short-term wind power prediction face challenges in effectively capturing hidden features in historical data characterized by local random fluc-tuations. Furthermore, these methods lack appropriate compensation mechanisms to account for deviations between predicted and actual wind farm results. This paper proposes a novel wind power prediction model that addresses these limitations through feature enhancement and autoregressive error compensation. The model emphasizes the relationship between six wind power variables collected in the field and utilizes bidirectional long short-term memory to capture bidirectional time series characteristics. The attention mechanism is employed to assign different weights to these features, enhancing their representation and reducing computa-tional complexity. To overcome frequent local fluctuations in wind power, an autoregressive error compensation model is integrated to further improve prediction accuracy. The proposed method is validated through practical application in a wind power plant, and experimental results demonstrate significant reduction in root mean square error compared to other models. This approach effectively extracts change trends in non-stationary wind power data, enhancing short-term power prediction performance. The proposed method has potential for application in other large-scale wind farms within the hybrid prediction framework.
MoreTranslated text
Key words
Wind power prediction,Attention mechanism,Bidirectional long short-term memory,Autoregressive integrated moving average model,Error compensation
AI Read Science
Must-Reading Tree
Example
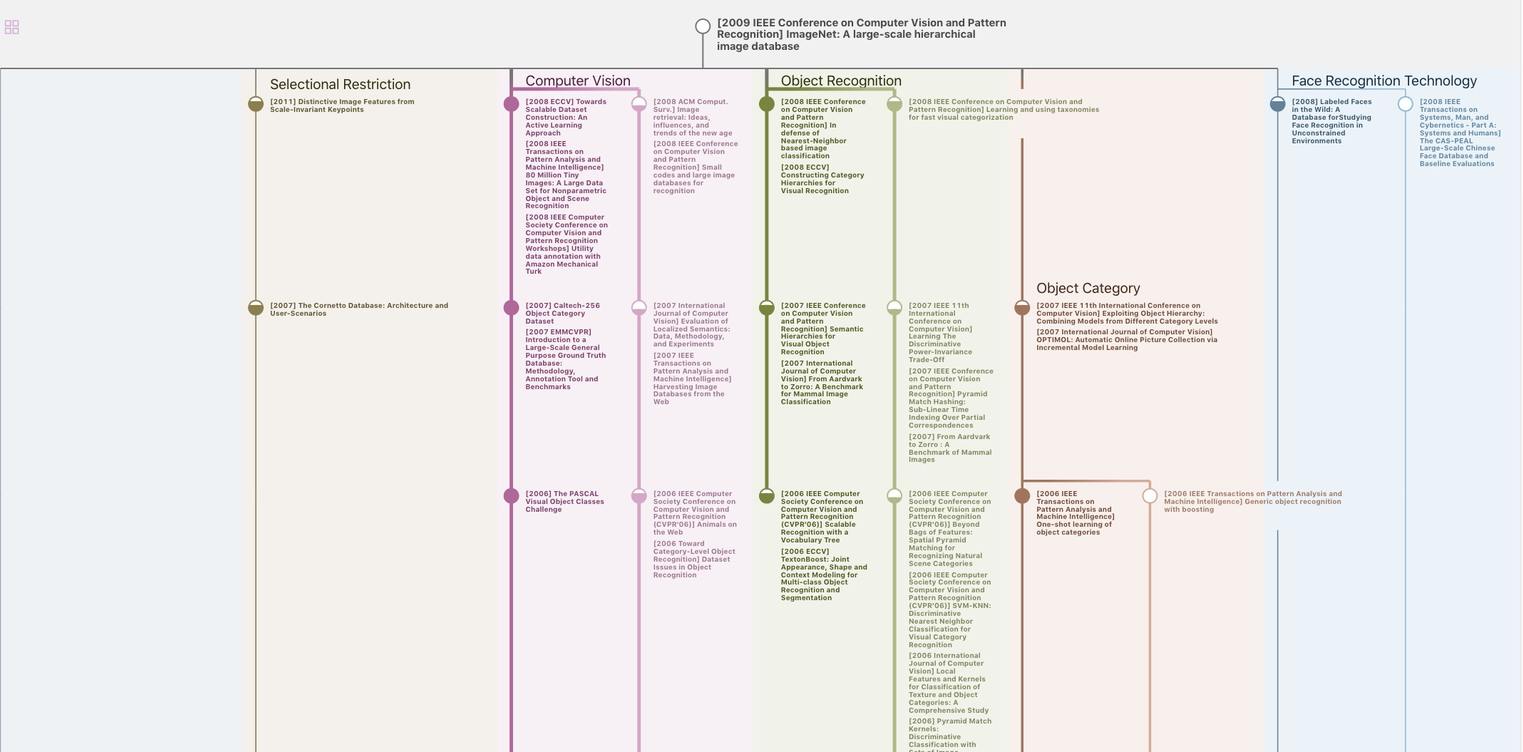
Generate MRT to find the research sequence of this paper
Chat Paper
Summary is being generated by the instructions you defined