Addressing gaps in data on drinking water quality through data integration and machine learning: evidence from Ethiopia
npj Clean Water(2023)
摘要
Monitoring access to safely managed drinking water services requires information on water quality. An increasing number of countries have integrated water quality testing in household surveys however it is not anticipated that such tests will be included in all future surveys. Using water testing data from the 2016 Ethiopia Socio-Economic Survey (ESS) we developed predictive models to identify households using contaminated (≥1 E. coli per 100 mL) drinking water sources based on common machine learning classification algorithms. These models were then applied to the 2013–2014 and 2018–2019 waves of the ESS that did not include water testing. The highest performing model achieved good accuracy (88.5%; 95% CI 86.3%, 90.6%) and discrimination (AUC 0.91; 95% CI 0.89, 0.94). The use of demographic, socioeconomic, and geospatial variables provided comparable results to that of the full features model whereas a model based exclusively on water source type performed poorly. Drinking water quality at the point of collection can be predicted from demographic, socioeconomic, and geospatial variables that are often available in household surveys.
更多查看译文
关键词
water quality,data integration,machine learning,ethiopia
AI 理解论文
溯源树
样例
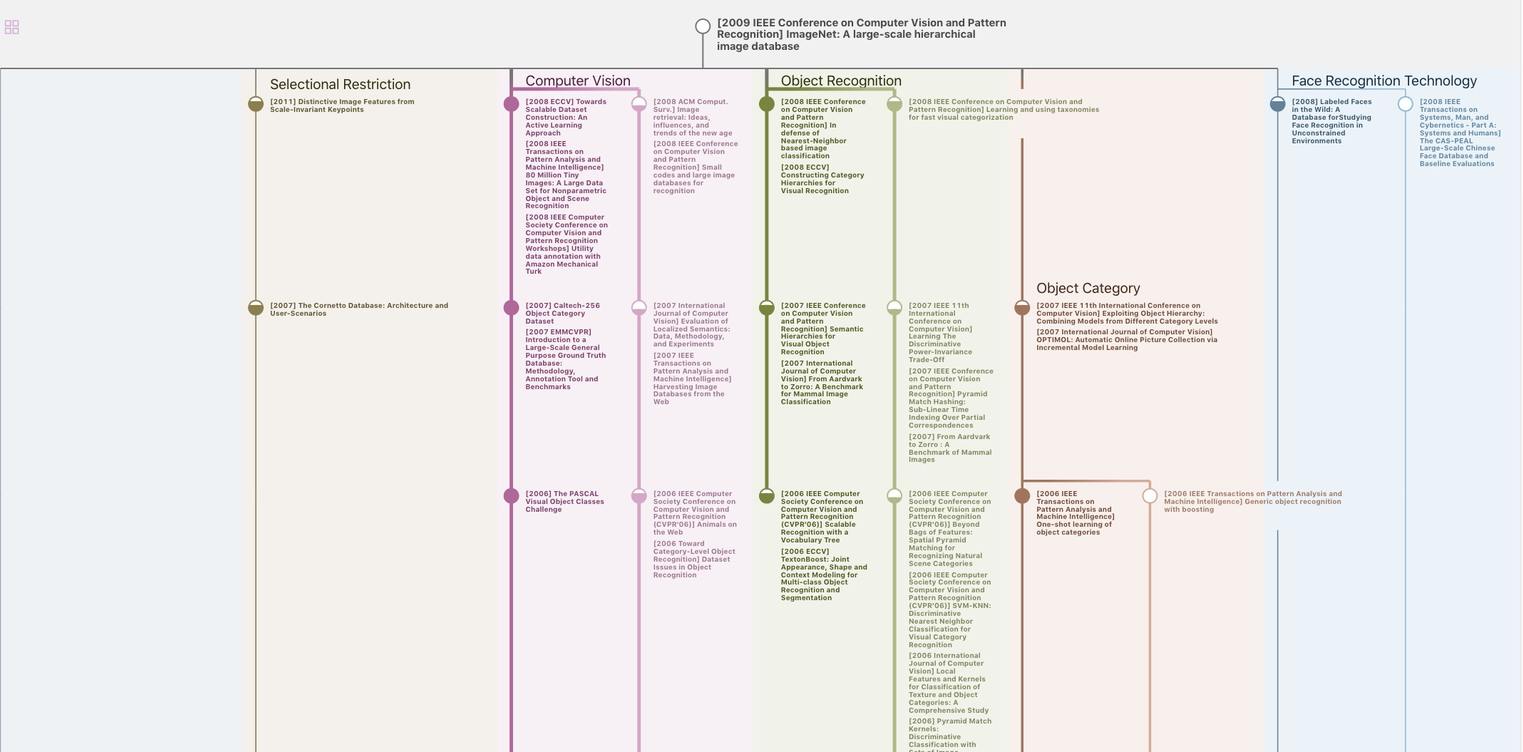
生成溯源树,研究论文发展脉络
Chat Paper
正在生成论文摘要