Adversarial Attacks for Neural Network Based Industrial Soft Sensors: Mirror Output Attack and Translation Mirror Output Attack
IEEE TRANSACTIONS ON INDUSTRIAL INFORMATICS(2024)
Abstract
Soft sensing using the neural network technique has been increasingly applied to industrial processes. Recently, the security and robustness of neural network based soft sensors have become primary concerns. Additionally, current studies indicated that neural networks are vulnerable to adversarial attacks. In other words, small perturbations imposed on the input can lead to significant deviations in the output. If a soft sensor for key process variables is attacked, considerable damage may be brought to industrial processes. This paper focuses on the attack methods for neural network based industrial soft sensors. Considering the characteristics of industrial soft sensors, this paper proposes two new adversarial attack methods. The first method, called the Mirror Output Attack (MOA), is a subtle attack method that flips the output curve to change the direction of outputs. The second method, called the Translation Mirror Output Attack (TMOA), is easy to make operators misoperate. TMOA translates the output curve while flipping the output curve to achieve the purpose of changing the output conditions. The effectiveness of MOA and TMOA is demonstrated in an industrial case study of the sulfur recovery unit (SRU) process. Simulation results show that the neural network based industrial soft sensors can be attacked by both of the two proposed adversarial attack methods. The study of adversarial attack methods can provide a basis for defending against attacks, thereby enhancing the security and robustness of soft sensors.
MoreTranslated text
Key words
Soft sensing,Adversarial attack,Mirror output attack(MOA),Translation mirror output attack(TMOA)
AI Read Science
Must-Reading Tree
Example
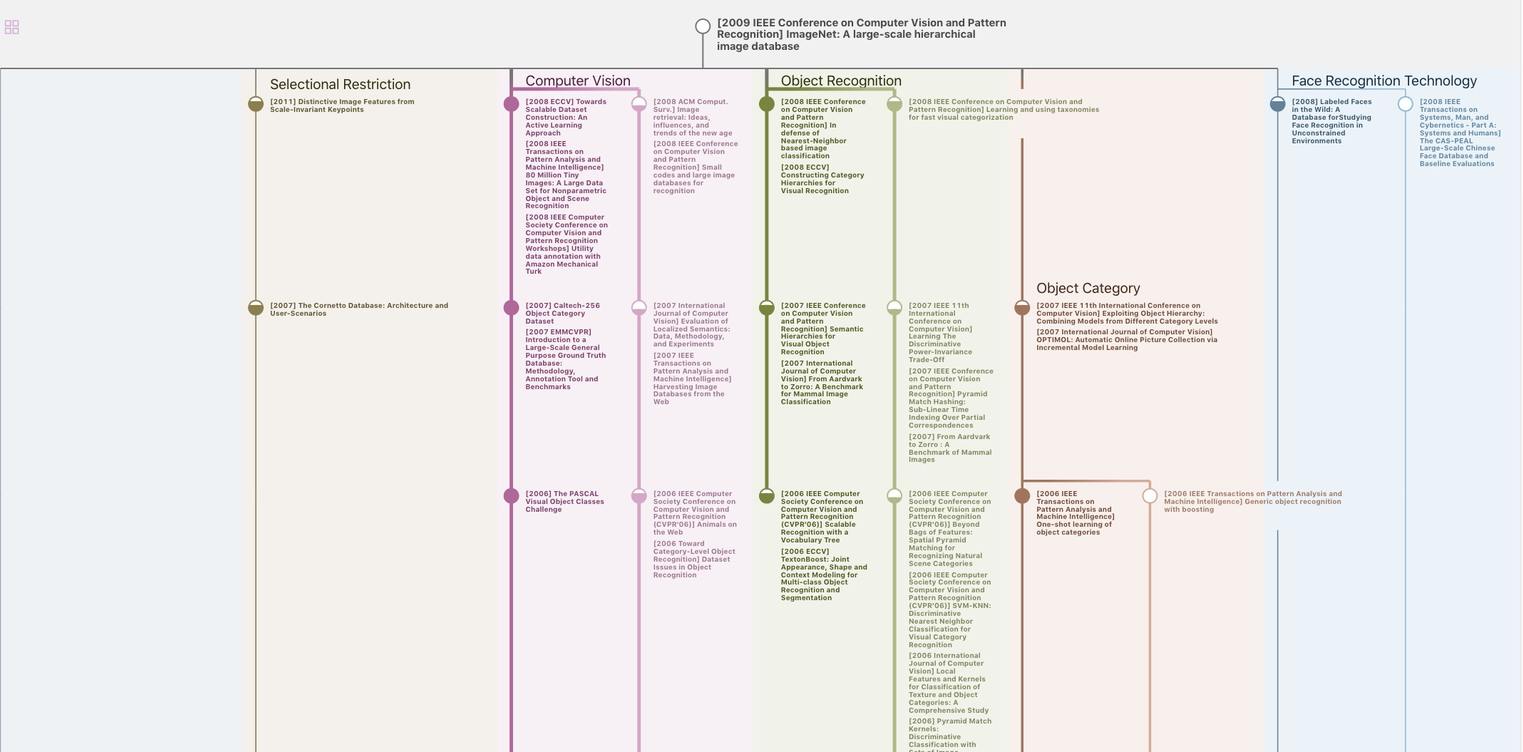
Generate MRT to find the research sequence of this paper
Chat Paper
Summary is being generated by the instructions you defined