A stochastic programming approach to the antibiotics time machine problem
Mathematical Biosciences(2024)
摘要
Antibiotics Time Machine is an important problem to understand antibiotic resistance and how it can be reversed. Mathematically, it can be modelled as follows: Consider a set of genotypes, each of which contain a set of mutated and unmutated genes. Suppose that a set of growth rate measurements of each genotype under a set of antibiotics are given. The transition probabilities of a ‘realization’ of a Markov chain associated with each arc under each antibiotic are computable via a predefined function given the growth rate realizations. The aim is to maximize the expected probability of reaching to the genotype with all unmutated genes given the initial genotype in a predetermined number of transitions, considering the following two sources of uncertainties: (i) the randomness in growth rates, (ii) the randomness in transition probabilities, which are functions of growth rates. We develop stochastic mixed-integer linear programming and dynamic programming approaches to solve static and dynamic versions of the Antibiotics Time Machine Problem under the aforementioned uncertainties. We adapt a Sample Average Approximation approach that exploits the special structure of the problem and provide accurate solutions that perform very well in an out-of-sample analysis.
更多查看译文
关键词
Fitness landscape,Bacterial growth rate,Drug delivery,Beta-lactamase,Mixed-integer linear programming,Dynamic programming
AI 理解论文
溯源树
样例
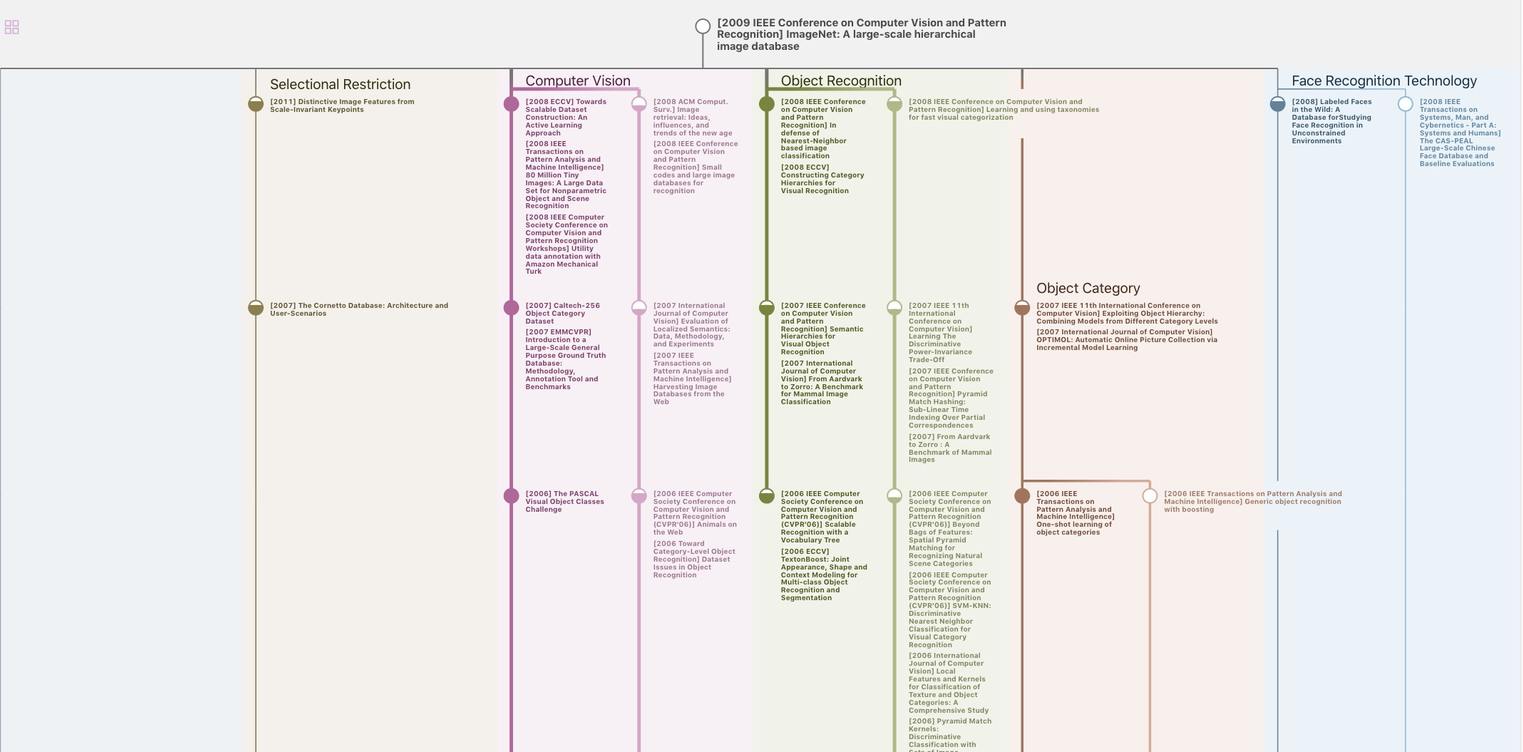
生成溯源树,研究论文发展脉络
Chat Paper
正在生成论文摘要