How prior and p-value heuristics are used when interpreting data
bioRxiv (Cold Spring Harbor Laboratory)(2023)
摘要
Scientific conclusions are based on the ways that researchers interpret data, a process that is shaped by psychological and cultural factors. When researchers use shortcuts known as heuristics to interpret data, it can sometimes lead to errors. To test the use of heuristics, we surveyed 623 researchers in biology and asked them to interpret scatterplots that showed ambiguous relationships, altering only the labels on the graphs. Our manipulations tested the use of two heuristics based on major statistical frameworks: (1) the strong prior heuristic, where a relationship is viewed as stronger if it is expected a priori, following Bayesian statistics, and (2) the p-value heuristic, where a relationship is viewed as stronger if it is associated with a small p-value, following null hypothesis statistical testing. Our results show that both the strong prior and p-value heuristics are common. Surprisingly, the strong prior heuristic was more prevalent among inexperienced researchers, whereas its effect was diminished among the most experienced biologists in our survey. By contrast, we find that p-values cause researchers at all levels to report that an ambiguous graph shows a strong result. Together, these results suggest that experience in the sciences may diminish a researcher's Bayesian intuitions, while reinforcing the use of p-values as a shortcut for effect size. Reform to data science training in STEM could help reduce researchers' reliance on error-prone heuristics.
### Competing Interest Statement
The authors have declared no competing interest.
更多查看译文
关键词
heuristics,data,p-value
AI 理解论文
溯源树
样例
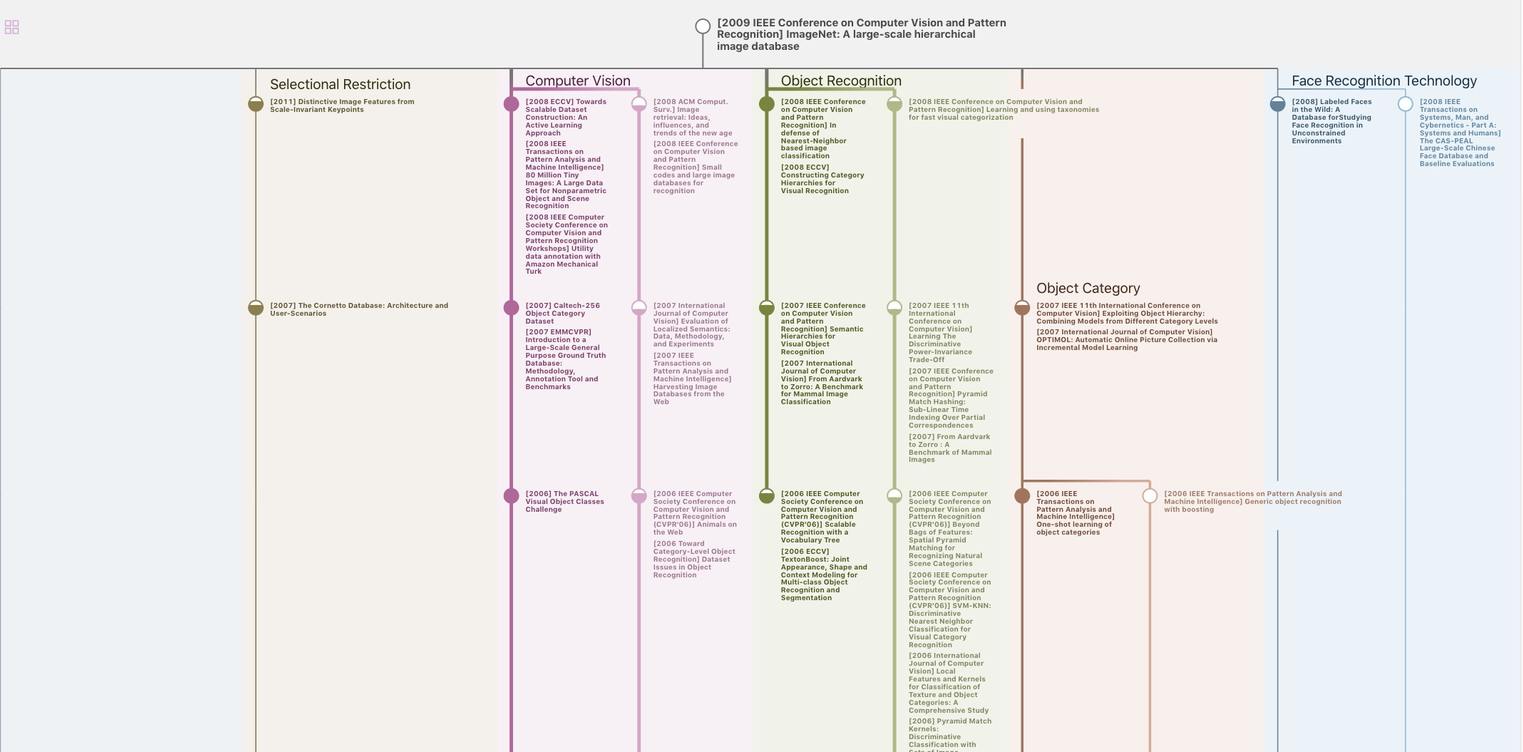
生成溯源树,研究论文发展脉络
Chat Paper
正在生成论文摘要