MS-UNet-v2: Adaptive Denoising Method and Training Strategy for Medical Image Segmentation with Small Training Data
CoRR(2023)
摘要
Models based on U-like structures have improved the performance of medical image segmentation. However, the single-layer decoder structure of U-Net is too "thin" to exploit enough information, resulting in large semantic differences between the encoder and decoder parts. Things get worse if the number of training sets of data is not sufficiently large, which is common in medical image processing tasks where annotated data are more difficult to obtain than other tasks. Based on this observation, we propose a novel U-Net model named MS-UNet for the medical image segmentation task in this study. Instead of the single-layer U-Net decoder structure used in Swin-UNet and TransUnet, we specifically design a multi-scale nested decoder based on the Swin Transformer for U-Net. The proposed multi-scale nested decoder structure allows the feature mapping between the decoder and encoder to be semantically closer, thus enabling the network to learn more detailed features. In addition, we propose a novel edge loss and a plug-and-play fine-tuning Denoising module, which not only effectively improves the segmentation performance of MS-UNet, but could also be applied to other models individually. Experimental results show that MS-UNet could effectively improve the network performance with more efficient feature learning capability and exhibit more advanced performance, especially in the extreme case with a small amount of training data, and the proposed Edge loss and Denoising module could significantly enhance the segmentation performance of MS-UNet.
更多查看译文
关键词
adaptive denoising method,image segmentation,small training data,ms-unet-v
AI 理解论文
溯源树
样例
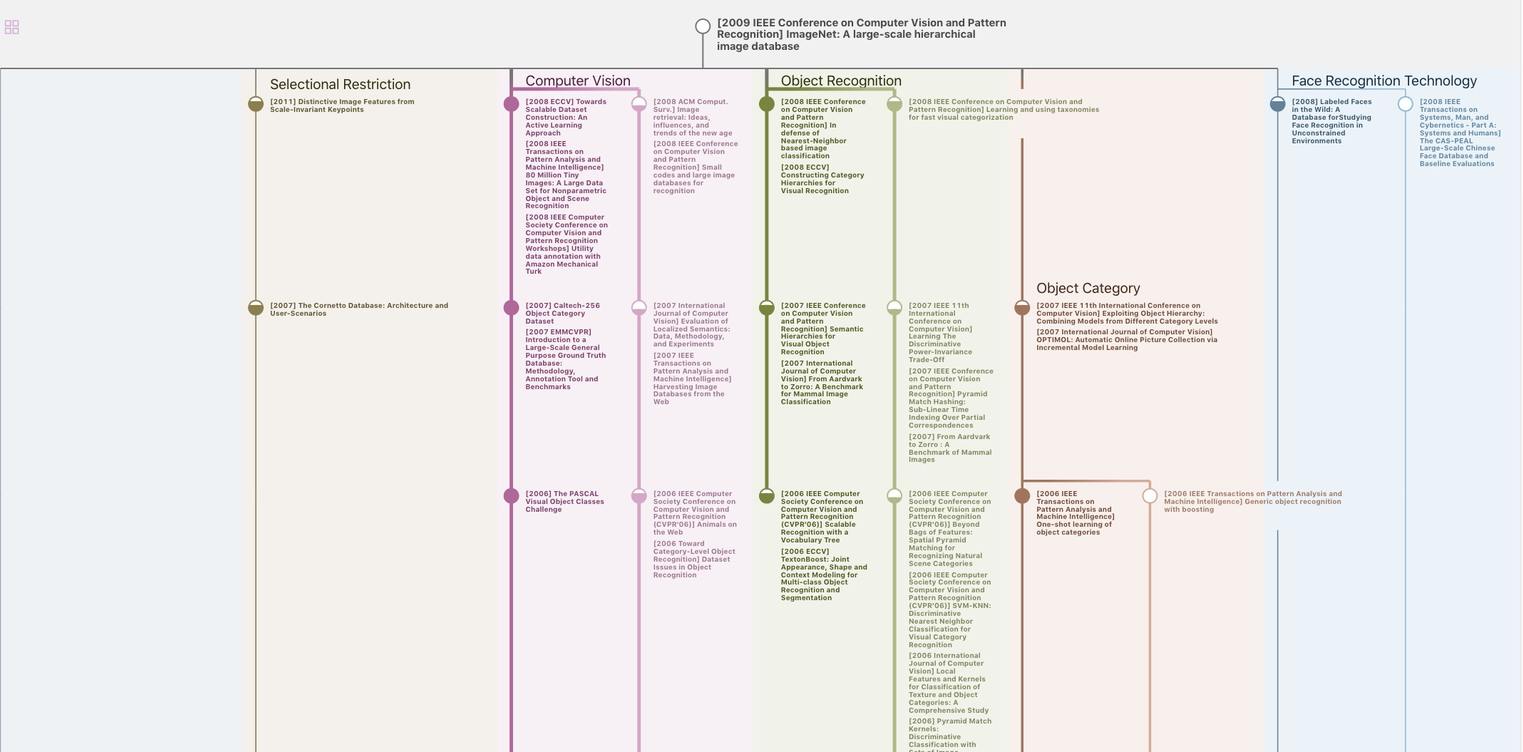
生成溯源树,研究论文发展脉络
Chat Paper
正在生成论文摘要