Manipulation of Neuronal Network Firing Patterns using Temporal Deep Unfolding-based MPC
2023 ASIA PACIFIC SIGNAL AND INFORMATION PROCESSING ASSOCIATION ANNUAL SUMMIT AND CONFERENCE, APSIPA ASC(2023)
摘要
Because neuronal networks are intricate systems composed of interconnected neurons, their control poses challenges owing to their nonlinearity and complexity. In this paper, we propose a method to design control input to a neuronal network to manipulate the firing patterns of modules within the network. We propose a methodology for designing a control input based on temporal deep unfolding-based model predictive control (TDU-MPC), a control methodology based on the deep unfolding technique actively investigated in the context of wireless signal processing. During the method development, we address the unique characteristics of neuron dynamics, such as zero gradients in firing times, by approximating input currents using a sigmoid function. The effectiveness of the proposed method is confirmed via numerical simulations. In networks with 15 and 30 neurons, the control was achieved to switch the firing frequencies of two modules without directly applying control inputs. This study includes a tailored methodology for networked neurons, the extension of TDU-MPC to nonlinear systems with reset dynamics, and the achievement of desired firing patterns in neuronal networks.
更多查看译文
AI 理解论文
溯源树
样例
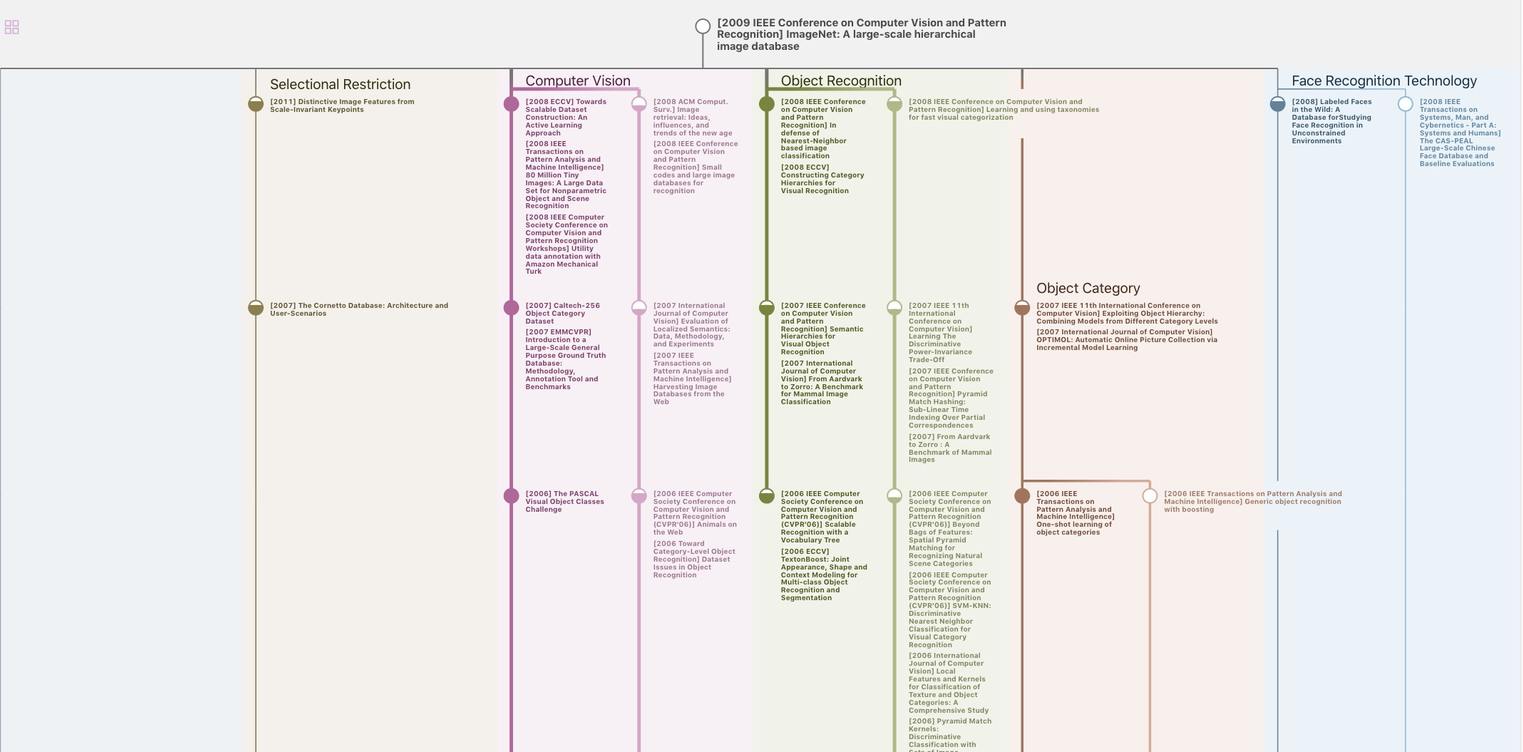
生成溯源树,研究论文发展脉络
Chat Paper
正在生成论文摘要