Evaluating the Efficacy of Supervised Learning vs Large Language Models for Identifying Cognitive Distortions and Suicidal Risks in Chinese Social Media
CoRR(2023)
摘要
Large language models, particularly those akin to the rapidly progressing GPT series, are gaining traction for their expansive influence. While there is keen interest in their applicability within medical domains such as psychology, tangible explorations on real-world data remain scant. Concurrently, users on social media platforms are increasingly vocalizing personal sentiments; under specific thematic umbrellas, these sentiments often manifest as negative emotions, sometimes escalating to suicidal inclinations. Timely discernment of such cognitive distortions and suicidal risks is crucial to effectively intervene and potentially avert dire circumstances. Our study ventured into this realm by experimenting on two pivotal tasks: suicidal risk and cognitive distortion identification on Chinese social media platforms. Using supervised learning as a baseline, we examined and contrasted the efficacy of large language models via three distinct strategies: zero-shot, few-shot, and fine-tuning. Our findings revealed a discernible performance gap between the large language models and traditional supervised learning approaches, primarily attributed to the models' inability to fully grasp subtle categories. Notably, while GPT-4 outperforms its counterparts in multiple scenarios, GPT-3.5 shows significant enhancement in suicide risk classification after fine-tuning. To our knowledge, this investigation stands as the maiden attempt at gauging large language models on Chinese social media tasks. This study underscores the forward-looking and transformative implications of using large language models in the field of psychology. It lays the groundwork for future applications in psychological research and practice.
更多查看译文
关键词
suicidal risks,chinese social media,large language models,cognitive distortions,social media
AI 理解论文
溯源树
样例
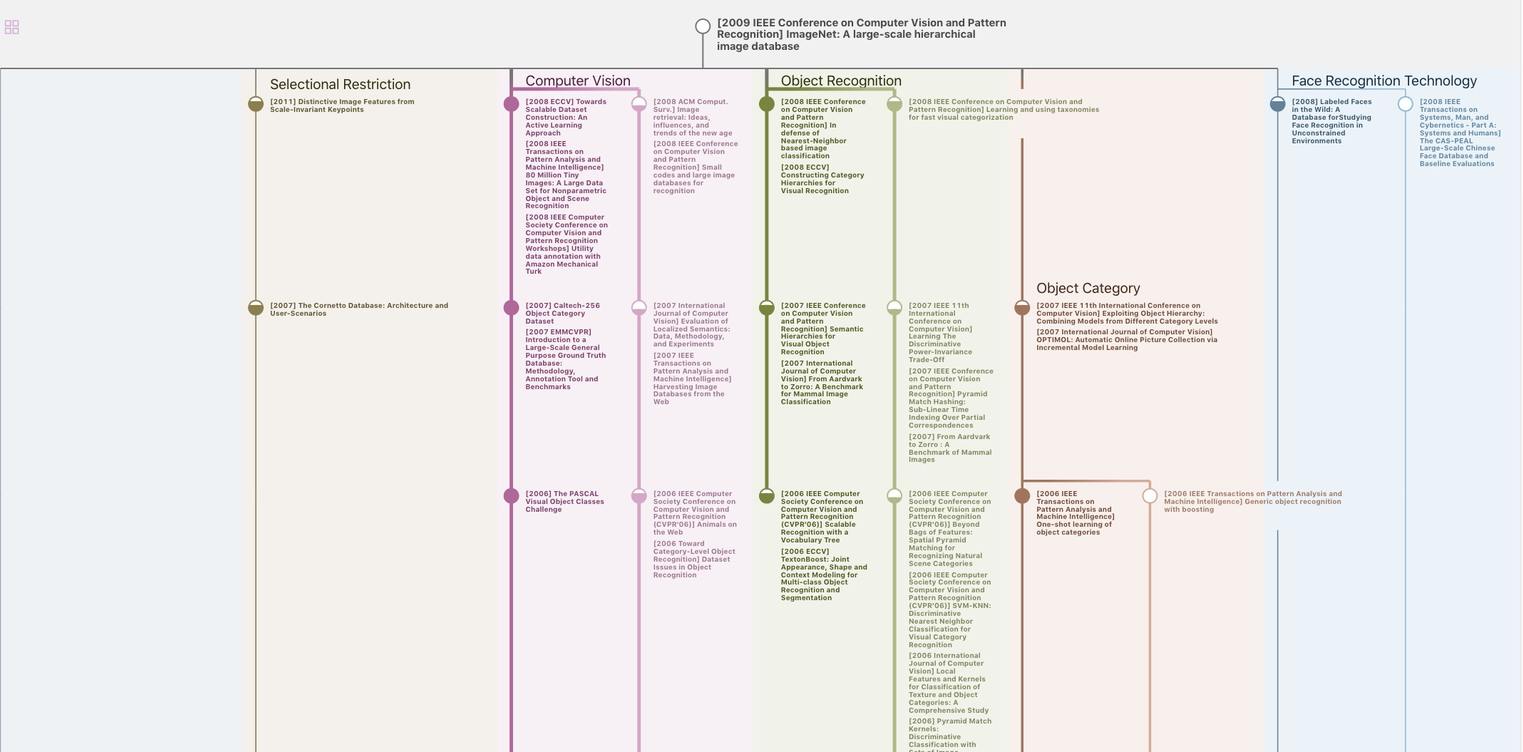
生成溯源树,研究论文发展脉络
Chat Paper
正在生成论文摘要