Autoregressive Omni-Aware Outpainting for Open-Vocabulary 360-Degree Image Generation
AAAI 2024(2024)
摘要
A 360-degree (omni-directional) image provides an all-encompassing spherical view of a scene. Recently, there has been an increasing interest in synthesising 360-degree images from conventional narrow field of view (NFoV) images captured by digital cameras and smartphones, for providing immersive experiences in various scenarios such as virtual reality. Yet, existing methods typically fall short in synthesizing intricate visual details or ensure the generated images align consistently with user-provided prompts. In this study, autoregressive omni-aware generative network (AOG-Net) is proposed for 360-degree image generation by outpainting an incomplete 360-degree image progressively with NFoV and text guidances joinly or individually. This autoregressive scheme not only allows for deriving finer-grained and text-consistent patterns by dynamically generating and adjusting the process but also offers users greater flexibility to edit their conditions throughout the generation process. A global-local conditioning mechanism is devised to comprehensively formulate the outpainting guidance in each autoregressive step. Text guidances, omni-visual cues, NFoV inputs and omni-geometry are encoded and further formulated with cross-attention based transformers into a global stream and a local stream into a conditioned generative backbone model. As AOG-Net is compatible to leverage large-scale models for the conditional encoder and the generative prior, it enables the generation to use extensive open-vocabulary text guidances. Comprehensive experiments on two commonly used 360-degree image datasets for both indoor and outdoor settings demonstrate the state-of-the-art performance of our proposed method. Our code is available at https://github.com/zhuqiangLu/AOG-NET-360.
更多查看译文
关键词
ML: Deep Generative Models & Autoencoders,CV: Applications
AI 理解论文
溯源树
样例
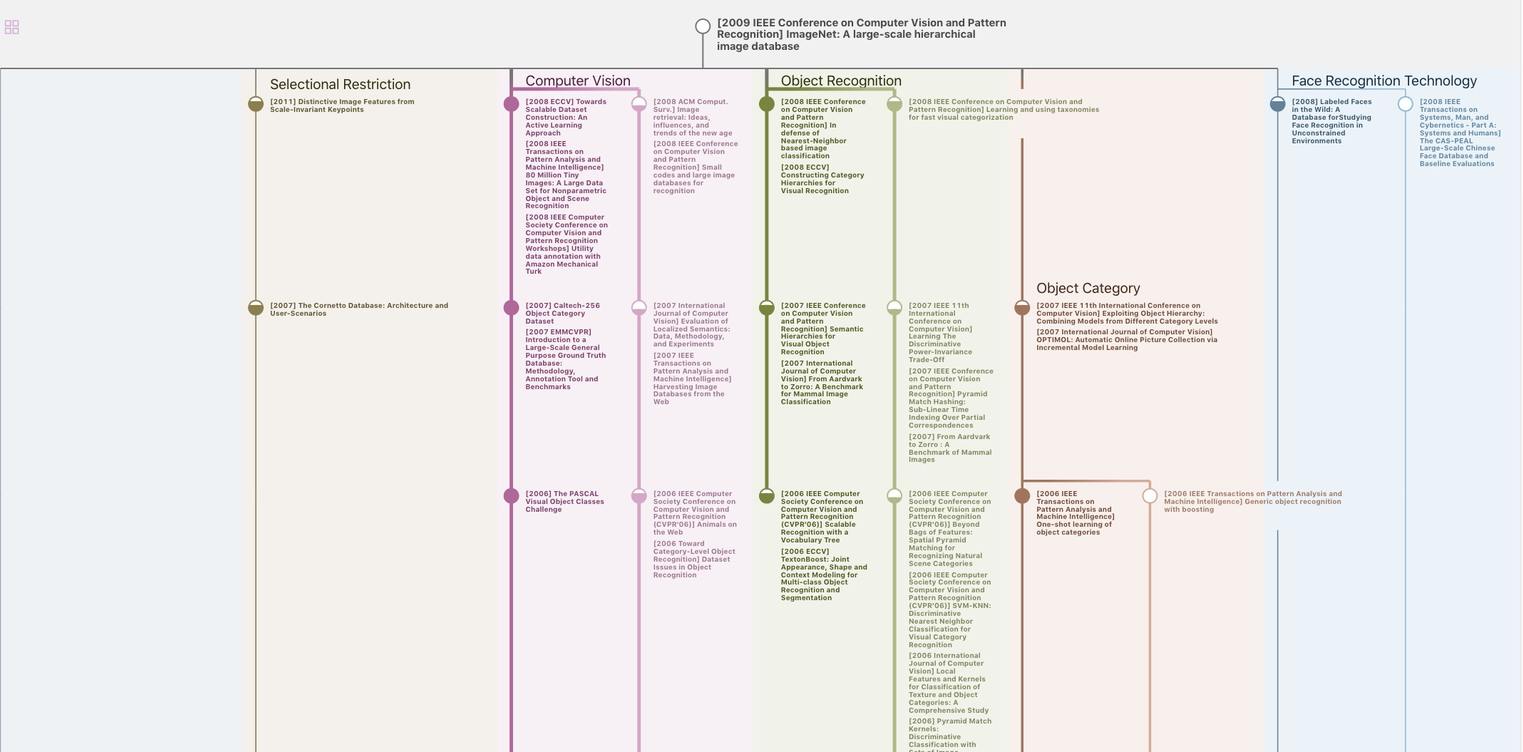
生成溯源树,研究论文发展脉络
Chat Paper
正在生成论文摘要