Companion Animal Disease Diagnostics Based on Literal-Aware Medical Knowledge Graph Representation Learning
CoRR(2023)
摘要
Knowledge graph (KG) embedding has been used to benefit the diagnosis of animal diseases by analyzing electronic medical records (EMRs), such as notes and veterinary records. However, learning representations to capture entities and relations with literal information in KGs is challenging as the KGs show heterogeneous properties and various types of literal information. Meanwhile, the existing methods mostly aim to preserve graph structures surrounding target nodes without considering different types of literals, which could also carry significant information. In this paper, we propose a knowledge graph embedding model for the efficient diagnosis of animal diseases, which could learn various types of literal information and graph structure and fuse them into unified representations, namely LiteralKG. Specifically, we construct a knowledge graph that is built from EMRs along with literal information collected from various animal hospitals. We then fuse different types of entities and node feature information into unified vector representations through gate networks. Finally, we propose a self-supervised learning task to learn graph structure in pretext tasks and then towards various downstream tasks. Experimental results on link prediction tasks demonstrate that our model outperforms the baselines that consist of state-of-the-art models.
更多查看译文
关键词
Medical knowledge graph embedding,disease diagnosis,companion animal disease
AI 理解论文
溯源树
样例
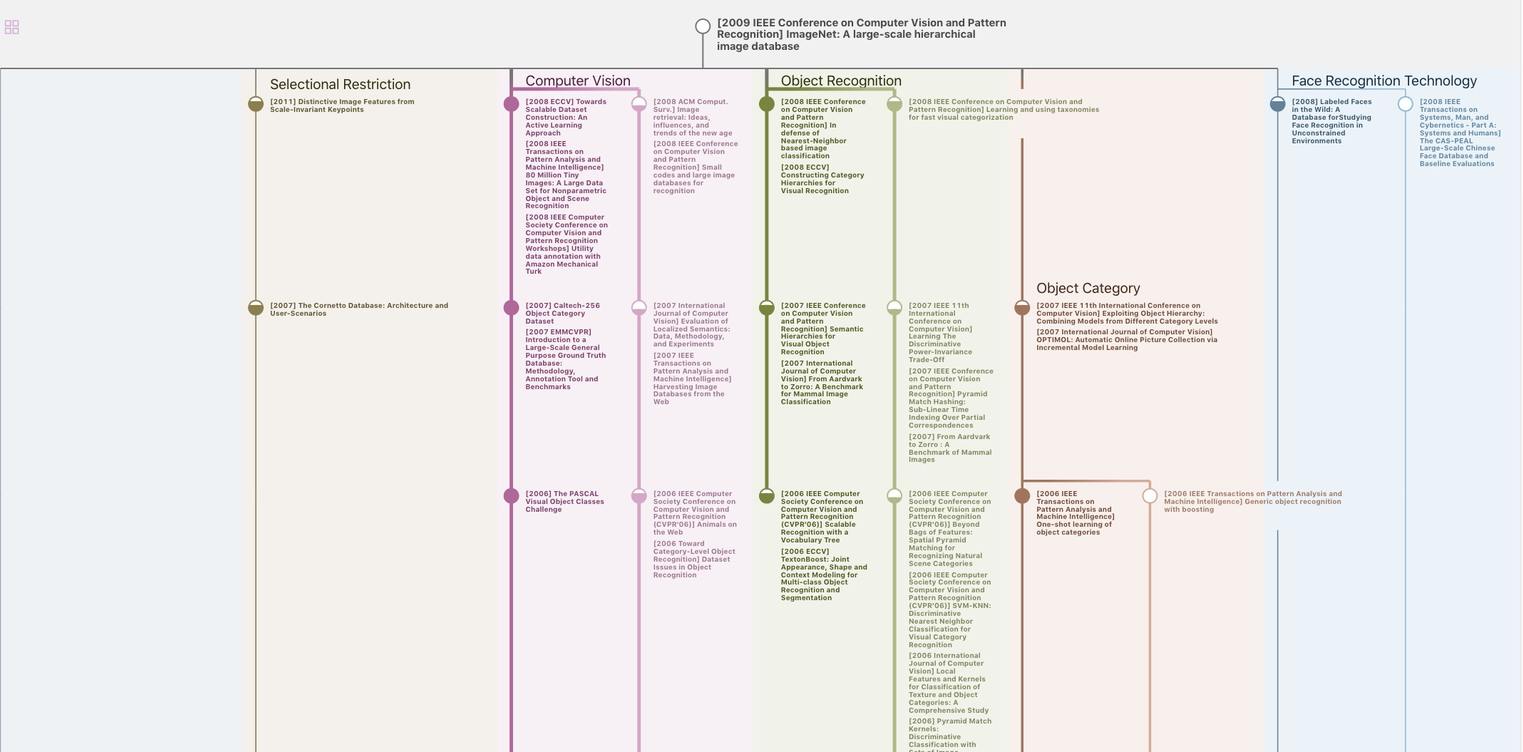
生成溯源树,研究论文发展脉络
Chat Paper
正在生成论文摘要