A support vector regression-based method for modeling geometric errors in CNC machine tools
The International Journal of Advanced Manufacturing Technology(2024)
摘要
For the problem of geometric error prediction of CNC machine tools, an improved hybrid grey wolf optimization (IHGWO) algorithm is proposed to optimize the geometric error modeling scheme of the support vector regression machine (SVR). The predicted and measured values of the geometric error are combined to construct the fitness function. In IHGWO, principles of particle swarm optimization (PSO) algorithm and dimension learning-based hunting (DLH) search strategies are introduced while retaining the excellent grey wolf position of the basic grey wolf optimization (GWO) algorithm. IHGWO algorithm uses Euclidean distance to construct the neighborhood of individual grey wolves, which enhances the ability to communicate between individual grey wolves and improves the convergence speed and accuracy of the algorithm. Predictive performance of SVR models using sum squared residual to quantify geometric error. Based on the screw theory, space models of geometric errors of CNC machine tools are established and combined with SVR models of geometric errors for compensation. Empirical evidence proves that the proposed method surpasses current error modeling methods in terms of precision and efficiency, as evidenced by a minimum reduction of 9% in circular trajectory error and a reduction to two overruns in S-shaped test pieces after error compensation. This research contributes to the field of CNC machine tool error modeling and has practical implications for manufacturing industries.
更多查看译文
关键词
Geometric errors,Support vector machine regression,Improved hybrid grey wolf optimization algorithm,CNC machine tools,Error compensation
AI 理解论文
溯源树
样例
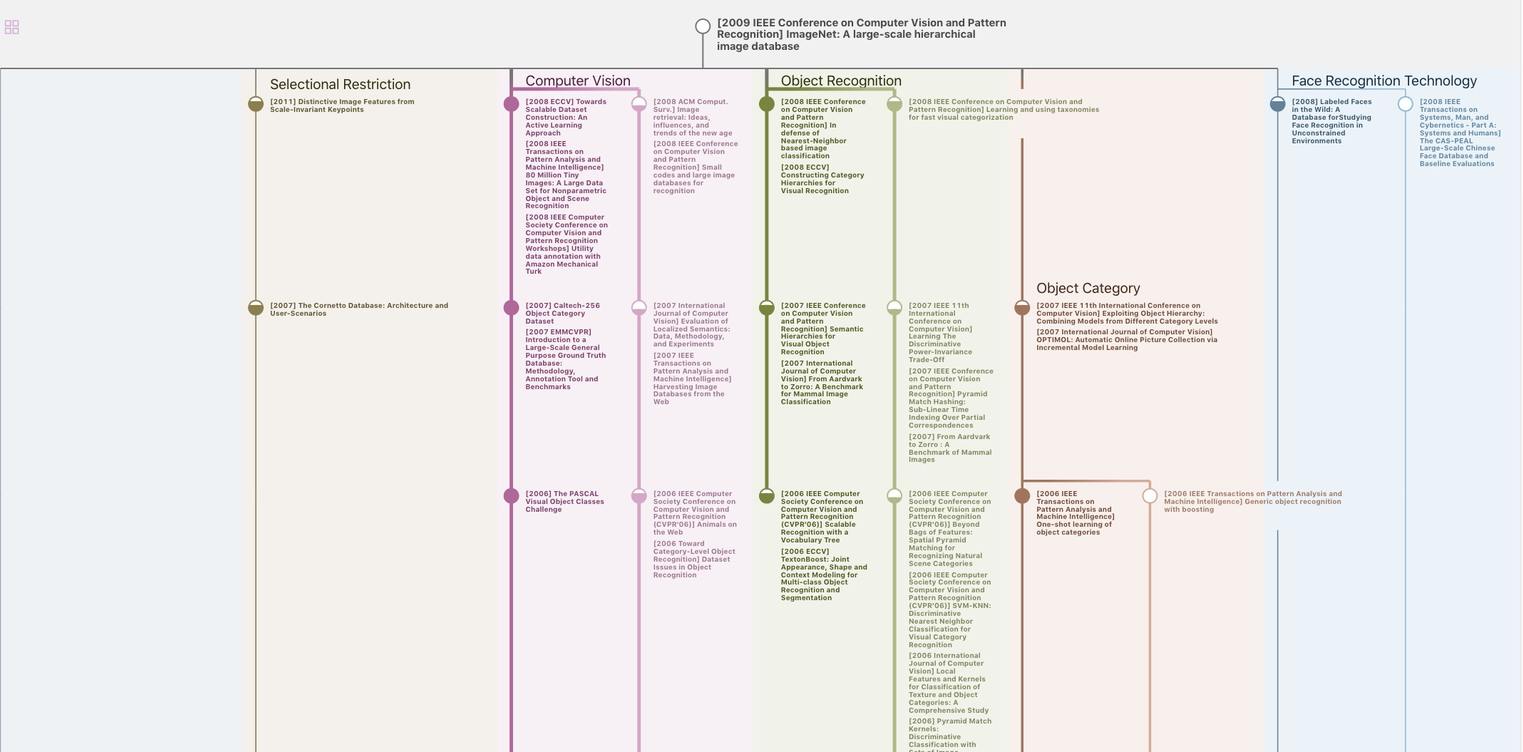
生成溯源树,研究论文发展脉络
Chat Paper
正在生成论文摘要