Plant Disease Detection and Segmentation using End-to-End YOLOv8: A Comprehensive Approach
2023 IEEE 13th International Conference on Control System, Computing and Engineering (ICCSCE)(2023)
摘要
Preventing and managing plant leaf diseases requires a dependable and precise detection method. Detecting leaf diseases in plants is a time-consuming process that has a negative impact on productivity and crop quality. By leveraging the PlantVillage and PlantDoc datasets to train the Ultralytics YOLOv8 model from end to end, this research intends to present a deep learning solution to the detection and segmentation of plant leaf disease. The YOLOv8 model, an advancement of the YOLO series, has been designed to increase detection speed without sacrificing accuracy. Its intricate architecture, composed of multiple convolutional layers, enables complex feature extraction from images, leading to precise identification of plant leaf diseases. As the model is trained end-to-end, it can effectively learn and generalize from the input data, thereby enhancing its predictive performance for unseen or novel instances of leaf diseases. The evaluation results for the YOLOv8 approach are validated by prominent statistical metrics like precision, recall, mAP50 and mAP50-95 value, and F1-score, which resulted in 99.8{%}, 99.3%, 99.5%, 96.5% and 0.999 for the bounding box and 99.1%, 99.3%, 99.3%, 98.5% and 0.992 for the segmentation mask respectively. The results demonstrate the model’s strong performance in accurately detecting and segmenting diseased regions, as indicated by high precision, recall, and mAP values. These findings highlight the effectiveness of the YOLOv8 model in plant disease detection, showcasing its potential for precision agriculture and crop management applications.
更多查看译文
关键词
Deep learning,YOLOv8,Instance segmentation,Precision agriculture,Plant disease detection
AI 理解论文
溯源树
样例
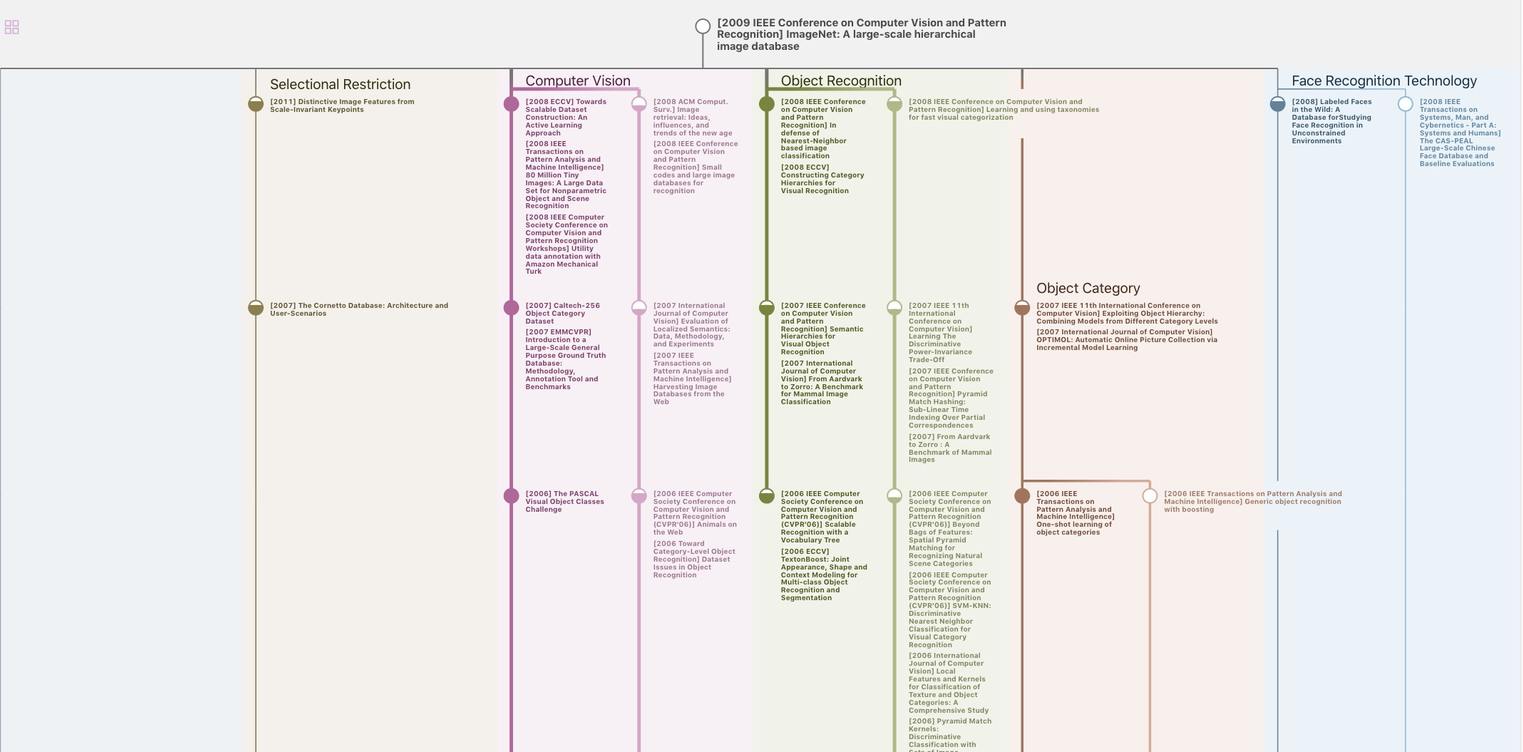
生成溯源树,研究论文发展脉络
Chat Paper
正在生成论文摘要