Cloud motion vector prediction based on optical flow and multi-scale feature fusion methods
JOURNAL OF NONLINEAR AND CONVEX ANALYSIS(2023)
Abstract
This study aimed to predict cloud motion vectors in satellite cloud mapping and examine edge blurring and feature loss. The study proposed a new Multi-scale Feature Fusion with Attention gate of FlowNet model that connected the image features of different depths using a multi-scale feature fusion design to maintain the integrity of high- and low-level feature information. We also added an attention mechanism and a convolution method in the fusion stage to eliminate the confounding effect that occurred in feature fusion to enhance the feature learning capability. The loss function of the model was also improved to fine-tune the details of the prediction results. The images in Red-Green-Blue space were converted into Hue-Saturation-Value space by dividing the colors, encoding the flow direction and magnitude of the images, and then obtaining the optical flow images to better predict the optical flow. The aforementioned process adaptively de-adjusted the learning rate via the Adam optimizer, which used an exponential shift calculation of [0.5, 0.999], making the gradient feature scaling of each parameter constant. The experimental results showed that the MFFAFlowNet model proposed in this study was more consistent with the true value images in terms of three evaluation metrics endpoint error, mean square error, and mean absolute error. The endpoint error metrics improved the accuracy level by about 4% and 8%, respectively, compared with the experimental results of the FlowNetC and LightFlow models under data set training, which verified the feasibility and effectiveness; the FlowNetC model created independent but identical processing streams for two images and combined them in the subsequent stage to get better results. The loss of information during the process due to the non-robustness of the cloud map led to unsatisfactory prediction results. The LightFlow model was able to get the cloud motion well, but it lost image flow direction and magnitude information. Hence, the prediction effect had a single color, and the MFFA-FlowNet model could achieve a more complete image flow direction and magnitude size during the application process and could obtain a more detailed cloud motion vector of the satellite cloud map.
MoreTranslated text
Key words
Attention gate, cloud motion vector, multi-scale feature fusion, satellite cloud map, typhoon
AI Read Science
Must-Reading Tree
Example
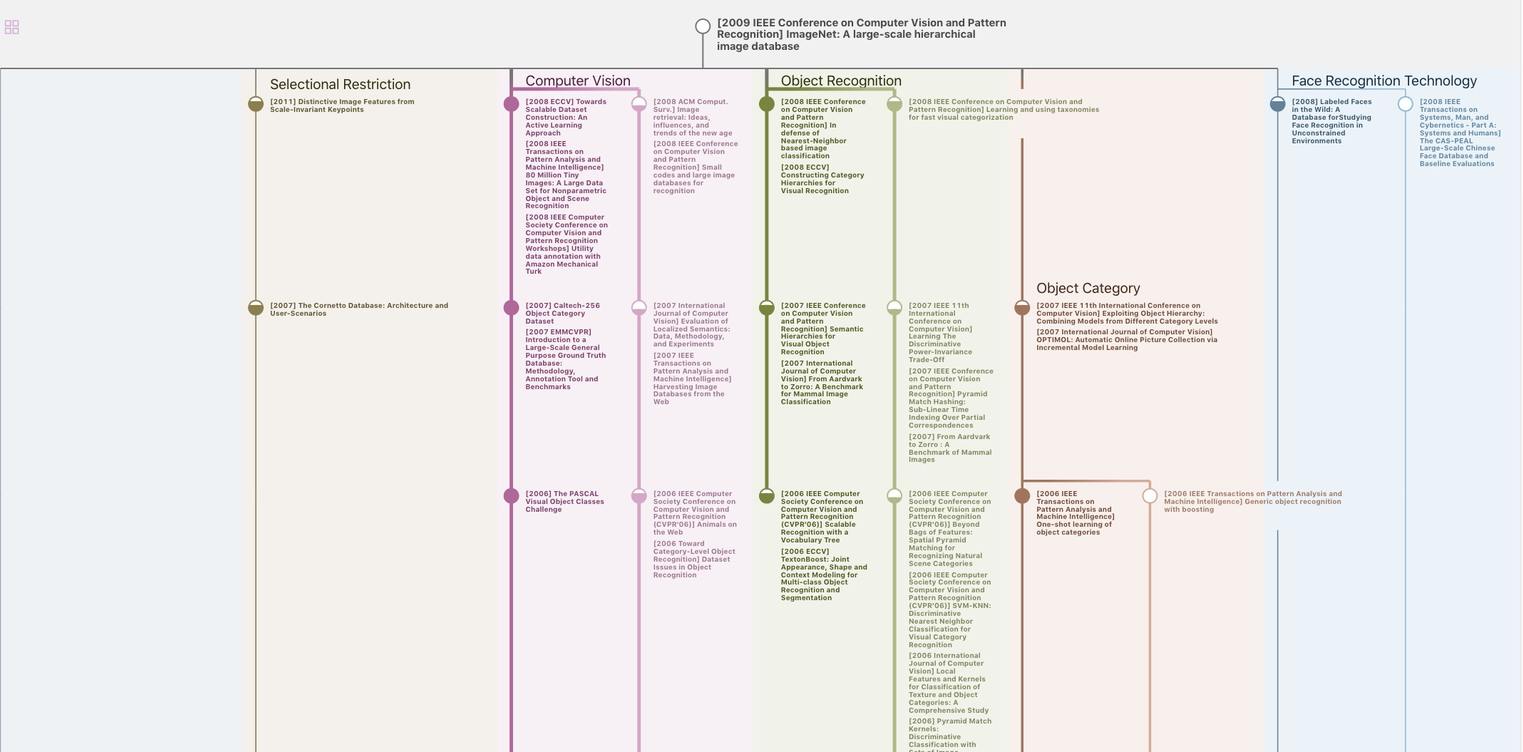
Generate MRT to find the research sequence of this paper
Chat Paper
Summary is being generated by the instructions you defined