Subtidal seagrass detector: development of a deep learning seagrass detection and classification model for seagrass presence and density in diverse habitats from underwater photoquadrats
FRONTIERS IN MARINE SCIENCE(2023)
Abstract
This paper presents the development and evaluation of a Subtidal Seagrass Detector (the Detector). Deep learning models were used to detect most forms of seagrass occurring in a diversity of habitats across the northeast Australian seascape from underwater images and classify them based on how much the cover of seagrass was present. Images were collected by scientists and trained contributors undertaking routine monitoring using drop-cameras mounted over a 50 x 50 cm quadrat. The Detector is composed of three separate models able to perform the specific tasks of: detecting the presence of seagrass (Model #1); classify the seagrass present into three broad cover classes (low, medium, high) (Model #2); and classify the substrate or image complexity (simple of complex) (Model #3). We were able to successfully train the three models to achieve high level accuracies with 97%, 80.7% and 97.9%, respectively. With the ability to further refine and train these models with newly acquired images from different locations and from different sources (e.g. Automated Underwater Vehicles), we are confident that our ability to detect seagrass will improve over time. With this Detector we will be able rapidly assess a large number of images collected by a diversity of contributors, and the data will provide invaluable insights about the extent and condition of subtidal seagrass, particularly in data-poor areas.
MoreTranslated text
Key words
deep learning seagrass detection,subtidal seagrass detector,seagrass presence,deep learning,diverse habitats
AI Read Science
Must-Reading Tree
Example
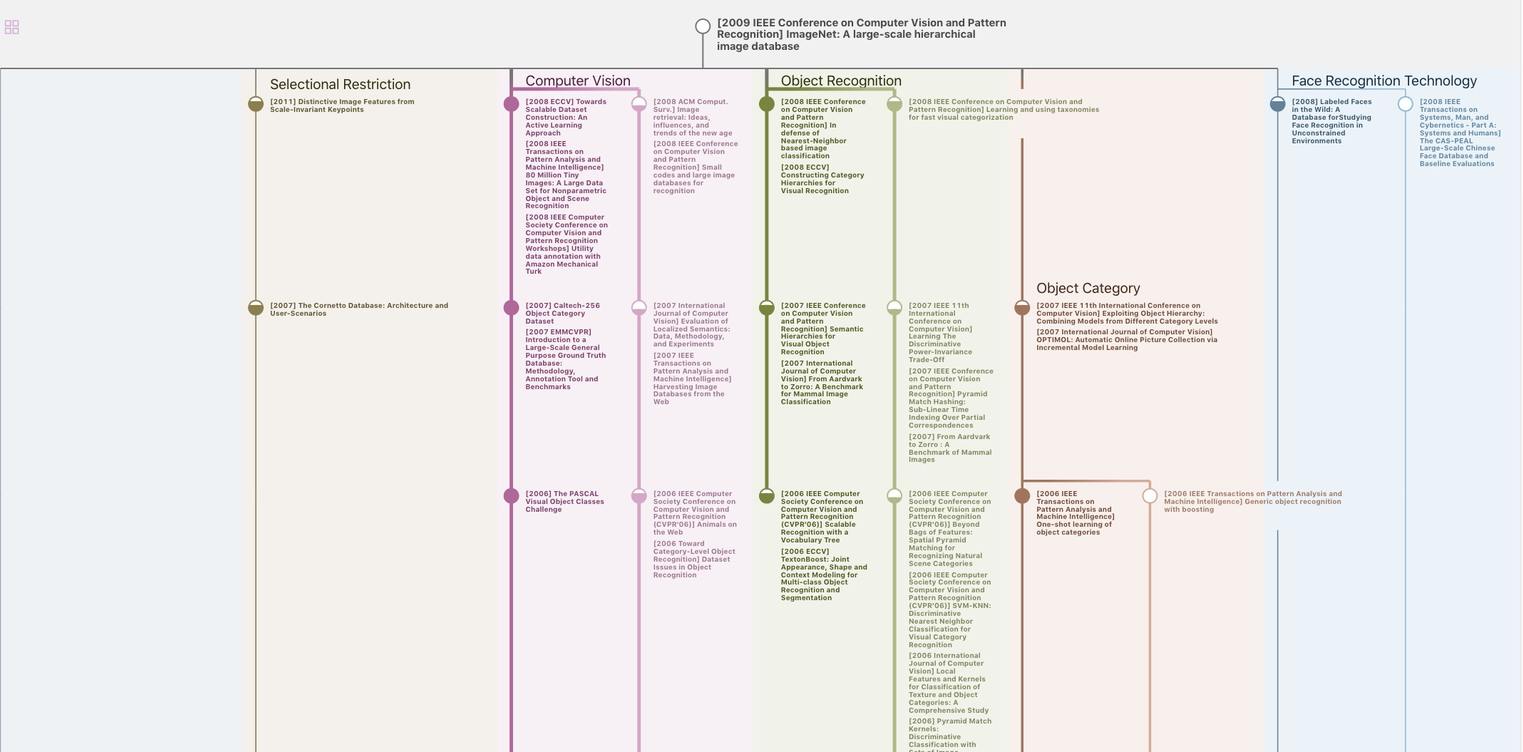
Generate MRT to find the research sequence of this paper
Chat Paper
Summary is being generated by the instructions you defined