Screening the stones of Venice: Mapping social perceptions of cultural significance through graph-based semi-supervised classification
ISPRS JOURNAL OF PHOTOGRAMMETRY AND REMOTE SENSING(2023)
摘要
Mapping cultural significance of heritage properties in urban environment from the perspective of the public has become an increasingly relevant process, as highlighted by the 2011 UNESCO Recommendation on the Historic Urban Landscape (HUL). With the ubiquitous use of social media and the prosperous developments in machine and deep learning, it has become feasible to collect and process massive amounts of information produced by online communities about their perceptions of heritage as social constructs. Moreover, such information is usually inter-connected and embedded within specific socioeconomic and spatiotemporal contexts. This paper presents a methodological workflow for using semi-supervised learning with graph neural networks (GNN) to classify, summarize, and map cultural significance categories based on user-generated content on social media. Several GNN models were trained as an ensemble to incorporate the multi-modal (visual and textual) features and the contextual (temporal, spatial, and social) connections of social media data in an attributed multi-graph structure. The classification results with different models were aligned and evaluated with the prediction confidence and agreement. Furthermore, message diffusion methods on graphs were proposed to aggregate the post labels onto their adjacent spatial nodes, which helps to map the cultural significance categories in their geographical contexts. The workflow is tested on data gathered from Venice as a case study, demonstrating the generation of social perception maps for this UNESCO World Heritage property. This research framework could also be applied in other cities worldwide, contributing to more socially inclusive heritage management processes. Furthermore, the proposed methodology holds the potential of diffusing any human-generated location-based information onto spatial networks and temporal timelines, which could be beneficial for measuring the safety, vitality, and/or popularity of urban spaces.
更多查看译文
关键词
Social media data,Multi-modal machine learning,Graph Neural Networks,Spectral centrality,Heritage values and attributes,Label diffusion
AI 理解论文
溯源树
样例
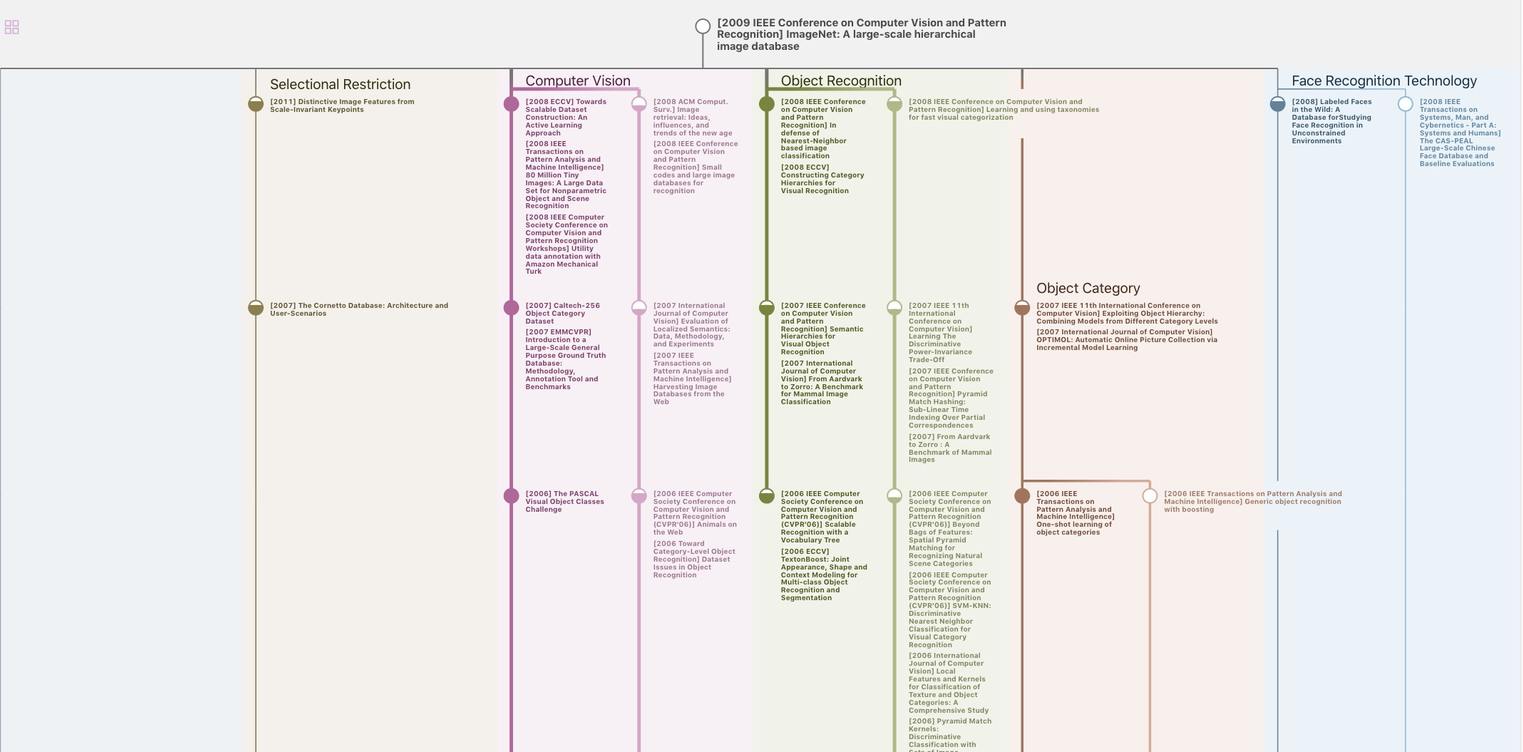
生成溯源树,研究论文发展脉络
Chat Paper
正在生成论文摘要