A novel method based on knowledge adoption model and non-kernel SVM for predicting the helpfulness of online reviews
JOURNAL OF THE OPERATIONAL RESEARCH SOCIETY(2023)
摘要
In this paper, a novel method is proposed to predict online review helpfulness based on the knowledge adoption model (KAM) theory and non-kernel weighted quadratic surface support vector machine (WQSSVM). The KAM theory develops helpfulness-predicting features from two perspectives: the quality of online review content and the credibility of online review sources. Content quality is measured by calculating the percentage of domain words in a review, as well as the percentage of stop words. This method effectively addresses the issue of knowledge barriers existing in the online reviews in specific domains. Source credibility is measured by looking at the number of fans an author has, as well as the number of likes he has received. The WQSSVM significantly reduces the computational time in selecting a suitable kernel and related parameters. To investigate the performance of the proposed method, computational experiments were conducted on three crawled online review datasets of traditional Chinese medicine, takeouts, and mobile phone products. The numerical results not only indicate the superior capability of the proposed method in identifying the optimal combination of features and forecasting accuracy but also indicate the greater importance of source credibility over quality of content, for predicting the online review helpfulness.
更多查看译文
关键词
Online reviews, forecasting method, knowledge adoption model, support vector machine, helpful information
AI 理解论文
溯源树
样例
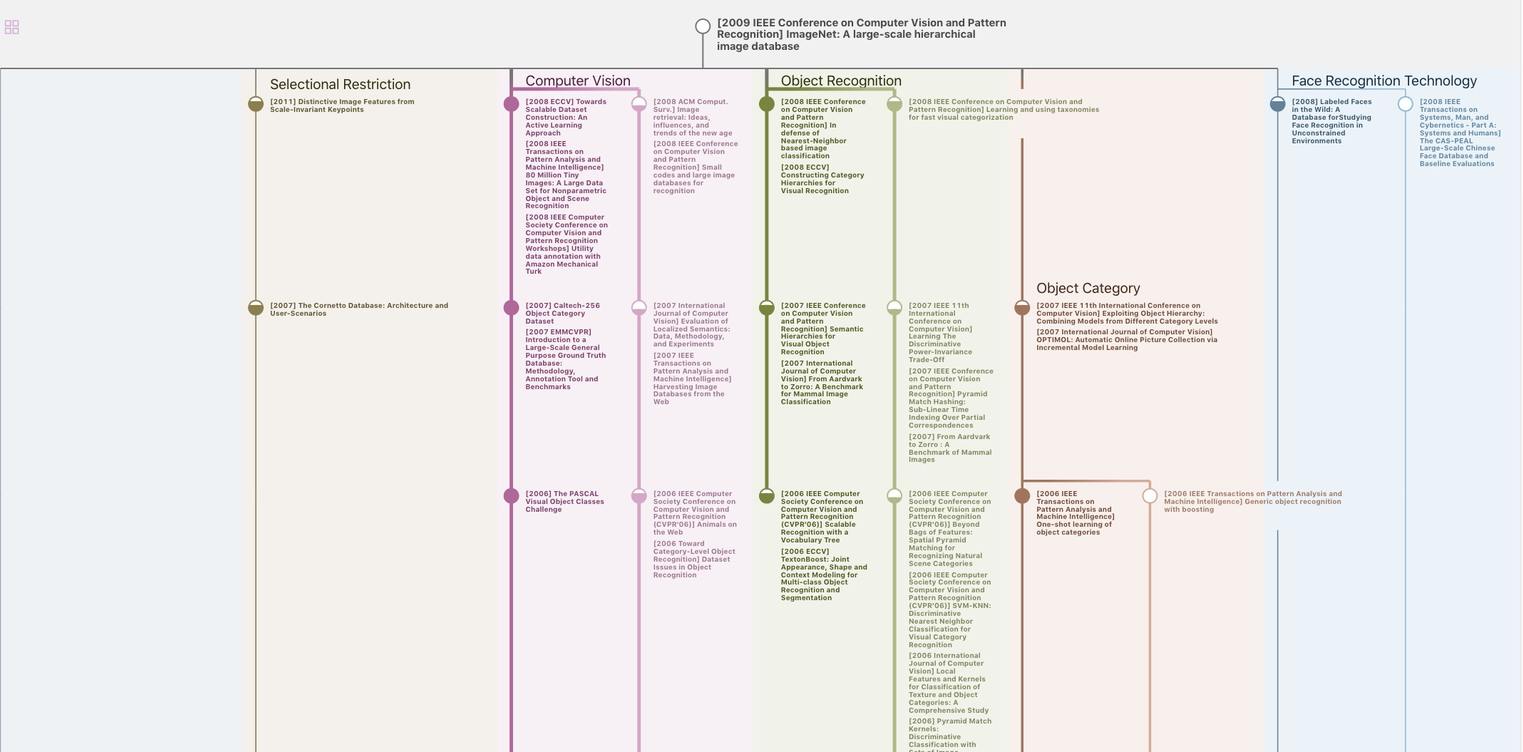
生成溯源树,研究论文发展脉络
Chat Paper
正在生成论文摘要