Deconvoluting experimental decay energy spectra: The 26O case
PHYSICAL REVIEW C(2023)
摘要
In nuclear reaction experiments, the measured decay energy spectra can give insights into the shell structure of decaying systems. However, extracting the underlying physics from the measurements is challenging due to detector resolution and acceptance effects. The Richardson-Lucy (RL) algorithm, a deblurring method that is commonly used in optics and has proven to be a successful technique for restoring images, was applied to our experimental nuclear physics data. The only inputs to the method are the observed energy spectrum and the detector's response matrix also known as the transfer matrix. We demonstrate that the technique can help access information about the shell structure of particle-unbound systems from the measured decay energy spectrum that is not immediately accessible via traditional approaches such as & chi;-square fitting. For a similar purpose, we developed a machine learning model that uses a deep neural network (DNN) classifier to identify resonance states from the measured decay energy spectrum. We tested the performance of both methods on simulated data and experimental measurements. Then, we applied both algorithms to the decay energy spectrum of 26O & RARR; 24O +n + n measured via invariant mass spectroscopy. The resonance states restored using the RL algorithm to deblur the measured decay energy spectrum agree with those found by the DNN classifier. Both deblurring and DNN approaches suggest that the raw decay energy spectrum of 26O exhibits three peaks at approximately 0.15 MeV, 1.50 MeV, and 5.00 MeV, with half-widths of 0.29 MeV, 0.80 MeV, and 1.85 MeV, respectively.
更多查看译文
AI 理解论文
溯源树
样例
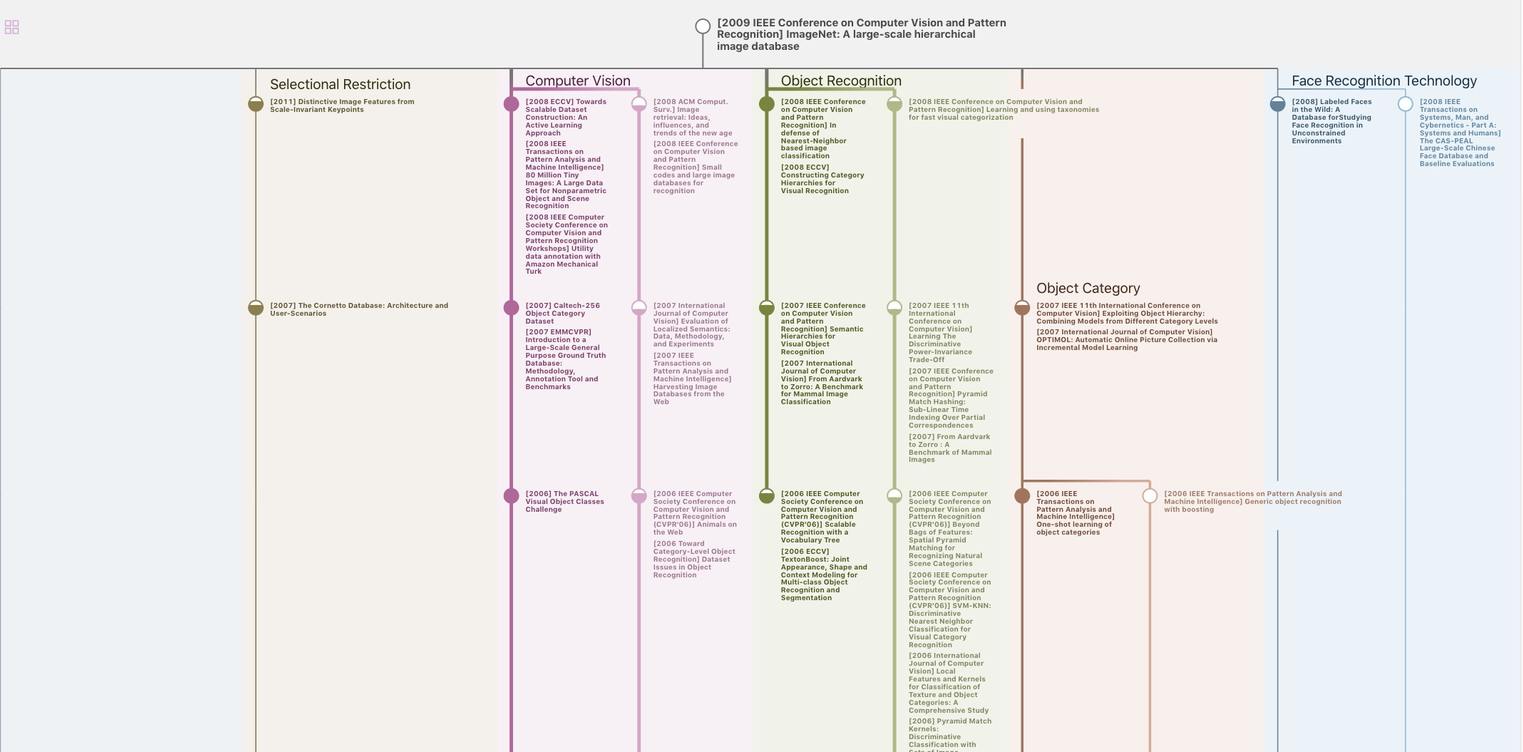
生成溯源树,研究论文发展脉络
Chat Paper
正在生成论文摘要