Measuring the Emission Changes and Meteorological Dependence of Source-Specific BC Aerosol Using Factor Analysis Coupled With Machine Learning
JOURNAL OF GEOPHYSICAL RESEARCH-ATMOSPHERES(2023)
摘要
Reducing ambient black carbon (BC) relies on the targeted control of anthropogenic emissions. Measuring emission changes in source-specific BC aerosol is essential to assess the effectiveness of regulatory policies but is difficult due to the presence of meteorology and multiple co-existing emissions. Herein, we propose a data-driven approach, combining dispersion-normalized factor analysis (DN-PMF) with a machine learning weather adjustment (deweathering) technique, to decompose ambient BC into source emissions and meteorological drivers. Six refined BC sources were extracted from the factor analysis of aethalometer multi-wavelength BC and concurrent observational datasets. In addition to the widely reported dominant sources, such as vehicular emissions (VE) and coal/biomass burning (BB), a discernible port and shipping emission source were identified with potential impacts on coastal air quality. The source-specific BC showed abrupt changes in response to interventions (e.g., holidays) after separating weather-related confounders. Significant reductions in deweathered coal and BB, VE, and local dust verified the effectiveness of policies, such as clean winter-heating and support for the Clean Air Actions. As revealed by a post-hoc model explanation technique, the evolution of the boundary layer was the predominant meteorological driver exerting the opposite impact on local sources with respect to distant regional-wide sources, that is, the port and shipping emissions.
更多查看译文
关键词
black carbon,source apportionment,machine learning,emission control,meteorology
AI 理解论文
溯源树
样例
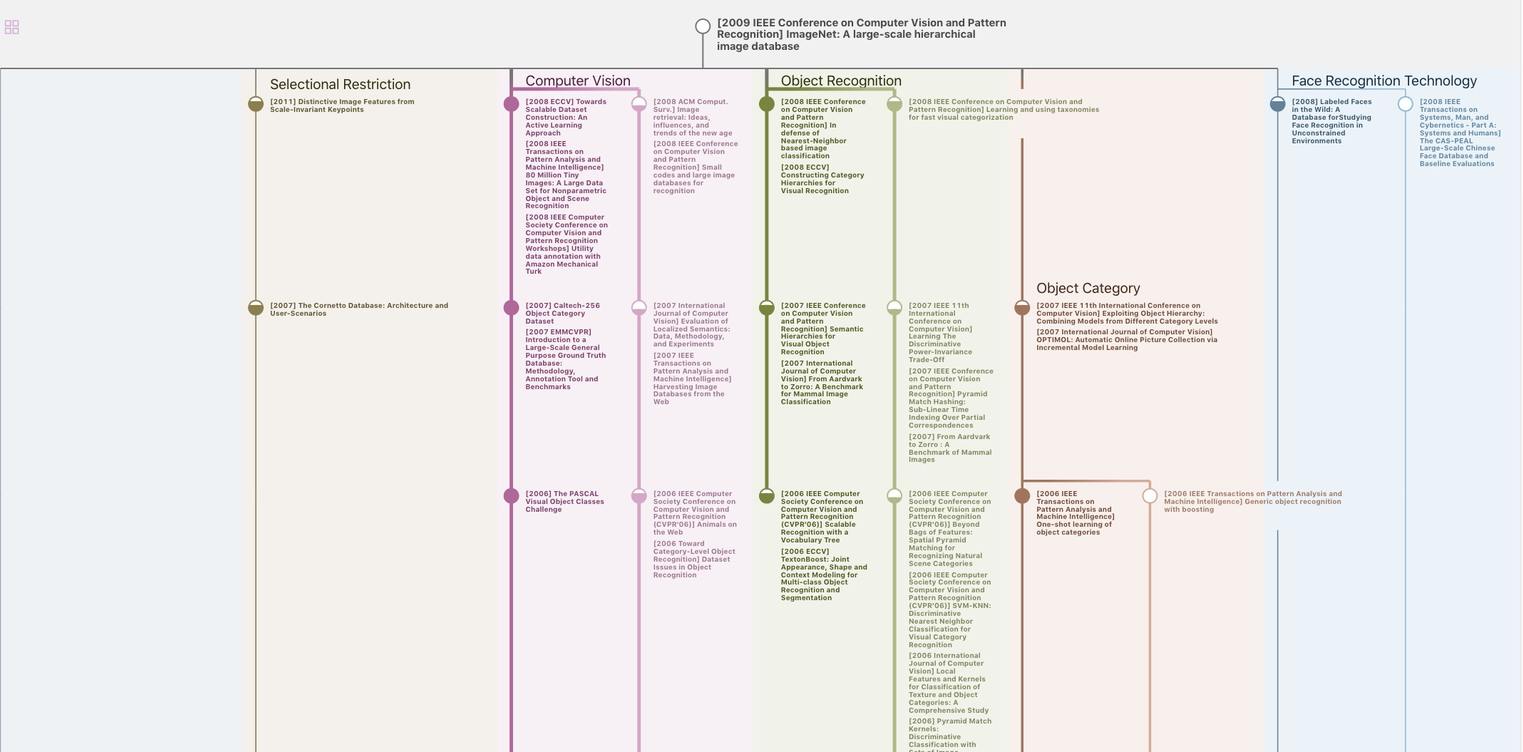
生成溯源树,研究论文发展脉络
Chat Paper
正在生成论文摘要