Multi-pass Bayesian estimation: a robust Bayesian method
COMPUTATIONAL STATISTICS(2023)
Abstract
The prior plays a central role in Bayesian inference but specifying a prior is often difficult and a prior considered appropriate by a modeler may be significantly biased. We propose multi-pass Bayesian estimation (MBE), a robust Bayesian method capable of adjusting the prior's influence on the inference result based on the prior's quality. MBE adjusts the relative importance of the prior and the data by iteratively performing approximate Bayesian updates on the given data, with the number of updates determined using a cross-validation method. The repeated use of the data resembles the data cloning method, but data cloning performs maximum likelihood estimation (MLE), while MBE interpolates between standard Bayesian inference and MLE; there are also algorithmic differences in how MBE and data cloning make repeated use of the data. Alternatively, MBE can be considered a method for constructing a new prior from the given initial prior and the data. We additionally provide a new non-asymptotic bound on the convergence of data cloning, and provide an MBE-like iterative heuristic approach which achieves faster convergence speed by boosting posterior variance. In numerical simulations on several simulated and real-world datasets, MBE provides robust inference results as compared to standard Bayesian inference and MLE.
MoreTranslated text
Key words
Bayesian method,Multi-pass Bayesian estimation,Maximum likelihood estimation,Prior
AI Read Science
Must-Reading Tree
Example
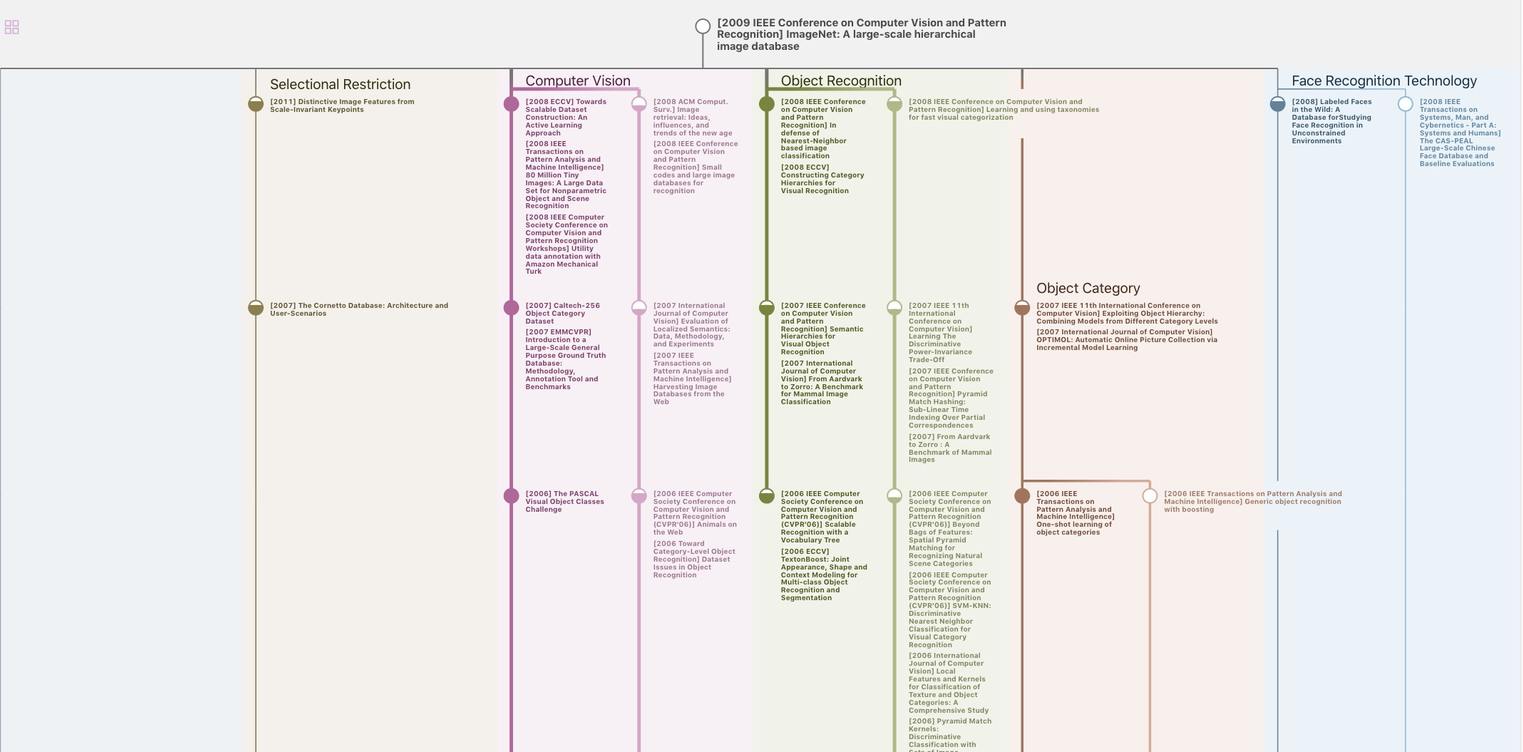
Generate MRT to find the research sequence of this paper
Chat Paper
Summary is being generated by the instructions you defined