Hyperparameter Optimization in Bagging-Based ELM Algorithm for Lithium-Ion Battery State of Health Estimation
2023 IEEE APPLIED POWER ELECTRONICS CONFERENCE AND EXPOSITION, APEC(2023)
摘要
Artificial neural networks are widely studied for the state of health (SOH) estimation of Lithium-ion batteries because they can recognize global features from the raw data and are able to cope with multi-dimensional data. But the performance of the model depends to some extent on the selection of the hyperparameters, which remain constant during model training. To improve the generalization performance as well as accuracy, an ensemble learning framework is proposed for battery SOH estimation, where multiple extreme learning machines are trained combined with bagging technology. The numbers of bags and neurons of the base model are then tuned by five commonly used hyperparameter optimization methods. Moreover, the SOH value with maximum probability density is selected as the output estimate to further improve the estimation accuracy. Finally, experimental results on both NMC and LPF batteries demonstrate that the proposed method with hyperparameter optimization can achieve stable and accurate battery SOH estimation. Regardless of which optimization method is used, the average percentage error for SOH estimation of NMC and LFP batteries can keep below 1% and 1.2%, respectively.
更多查看译文
关键词
Lithium-ion battery,state of health,robust estimation,ensemble learning
AI 理解论文
溯源树
样例
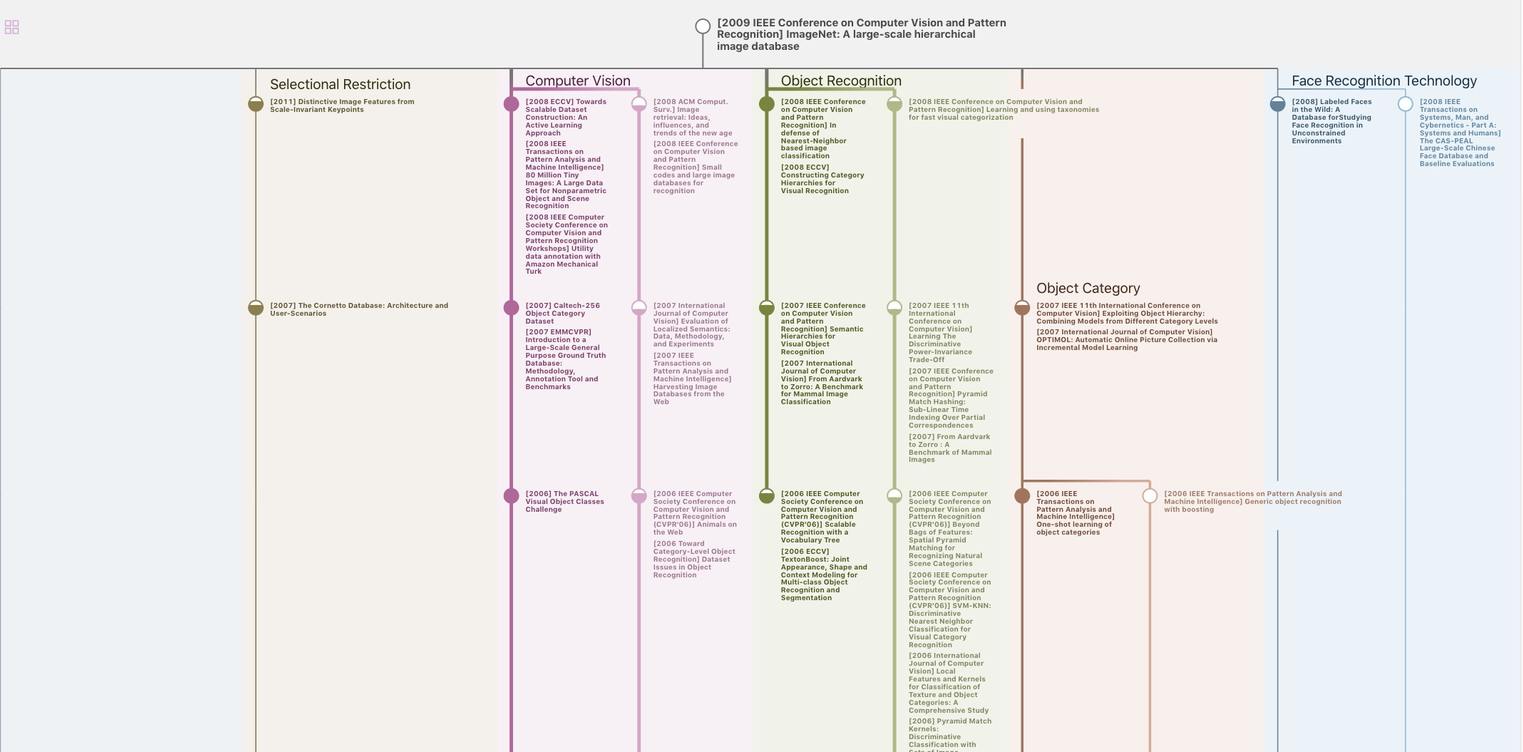
生成溯源树,研究论文发展脉络
Chat Paper
正在生成论文摘要