Optimization of machine learning classification models for tumor cells based on cell elements heterogeneity with laser-induced breakdown spectroscopy
JOURNAL OF BIOPHOTONICS(2023)
摘要
The rapid and accurate diagnosis of cancer is an important topic in clinical medicine. In the present work, an innovative method based on laser-induced breakdown spectroscopy (LIBS) combined with machine learning was developed to distinguish and classify different tumor cell lines. The LIBS spectra of cells were first acquired. Then the spectral pre-processing was performed as well as detailed optimization to improve the classification accuracy. After that, the convolutional neural network (CNN), support vector machine (SVM), and K-nearest neighbors were further compared for the optimized classification ability of tumor cells. Both the CNN algorithm and SVM algorithm have achieved impressive discrimination performances for tumor cells distinguishing, with an accuracy of 97.72%. The results show that the heterogeneity of elements in tumor cells plays an important role in distinguishing the cells. It also means that the LIBS technique can be used as a fast classification method for classifying tumor cells.
更多查看译文
关键词
cancer cell recognition,chemical elements,laser-induced breakdown spectroscopy (LIBS),machine learning,tumor cells
AI 理解论文
溯源树
样例
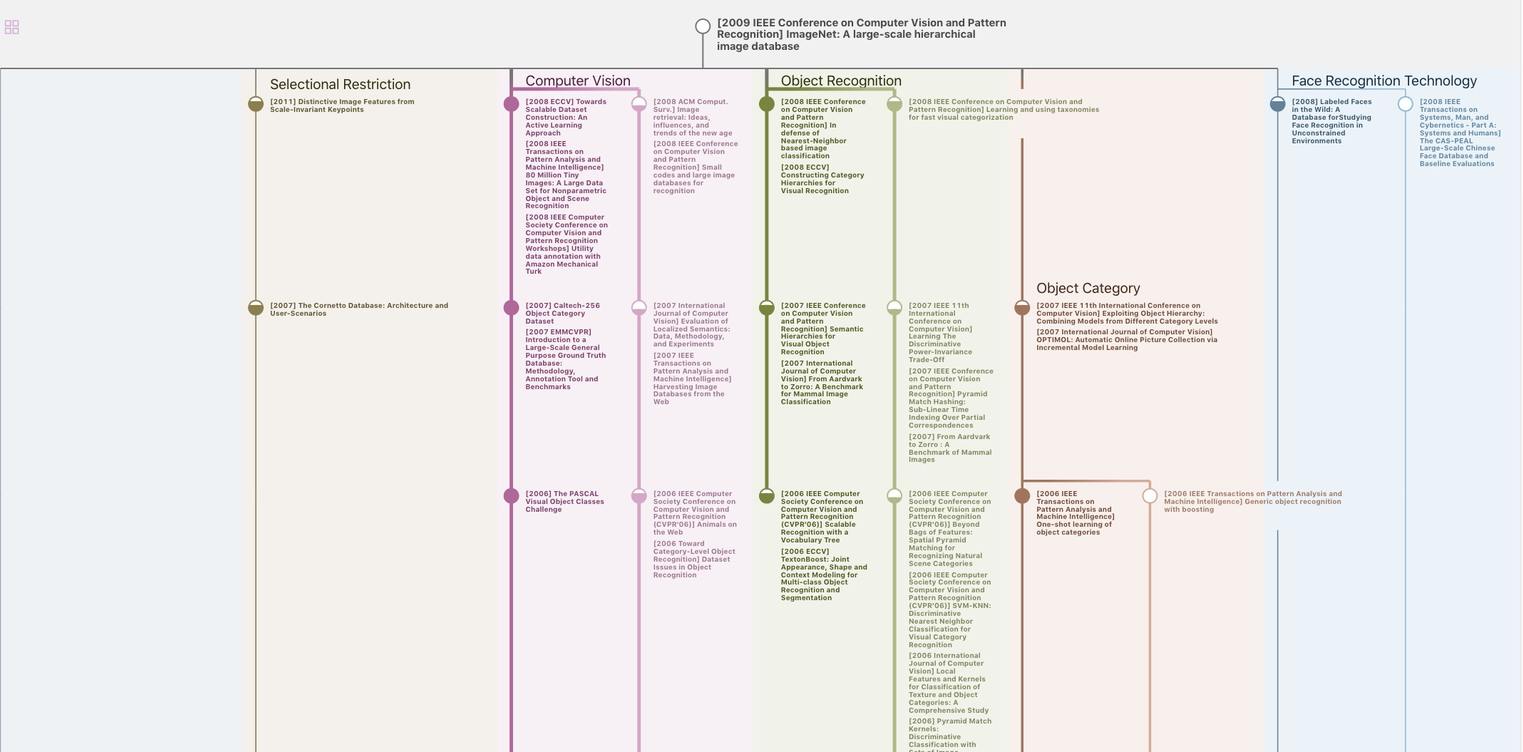
生成溯源树,研究论文发展脉络
Chat Paper
正在生成论文摘要