Multilevel Feature Extraction Method for Adaptive Fault Diagnosis of Railway Axle Box Bearing
SHOCK AND VIBRATION(2023)
摘要
Railway axle box bearing fault signal contains high Q-factor resonance and low Q-factor transient shock components with periodic transient shock features that can characterize bearing faults. However, extracting fault features is usually difficult due to noise, transmission paths, and high-amplitude accidental shocks. Therefore, to address the abovementioned problems, the multilevel feature extraction method for adaptive fault diagnosis of railway axle box bearing was proposed. This paper used the maximum second-order cyclostationary blind convolution (CYCBD) to weaken the influence of disturbances, enhancing weak transient shock components. Resonant sparse decomposition(RSSD) is used for the selection of quality factor Q due to its excellent fault feature separation property. Considering the strict fault frequency component periodicity in the envelope spectrum, the envelope spectrum multipoint kurtosis (ESMK) is proposed as a metric. The Q factor is optimized using the gray wolf optimization algorithm (GWO) to obtain an adaptive sparse decomposition method (GWO-RSSD) for extracting bearing transient fault shocks to eliminate the signal effects of high-amplitude disturbance shocks and background noise. The simulation results and measured signal analysis of railway axle box bearing show that ESMK can effectively measure periodic transient shocks under strong shock interference compared with the MED-RSSD method. Thus, GWO-RSSD can adaptively separate the optimal low-Q resonance components, verifying the methods' effectiveness and superiority.
更多查看译文
AI 理解论文
溯源树
样例
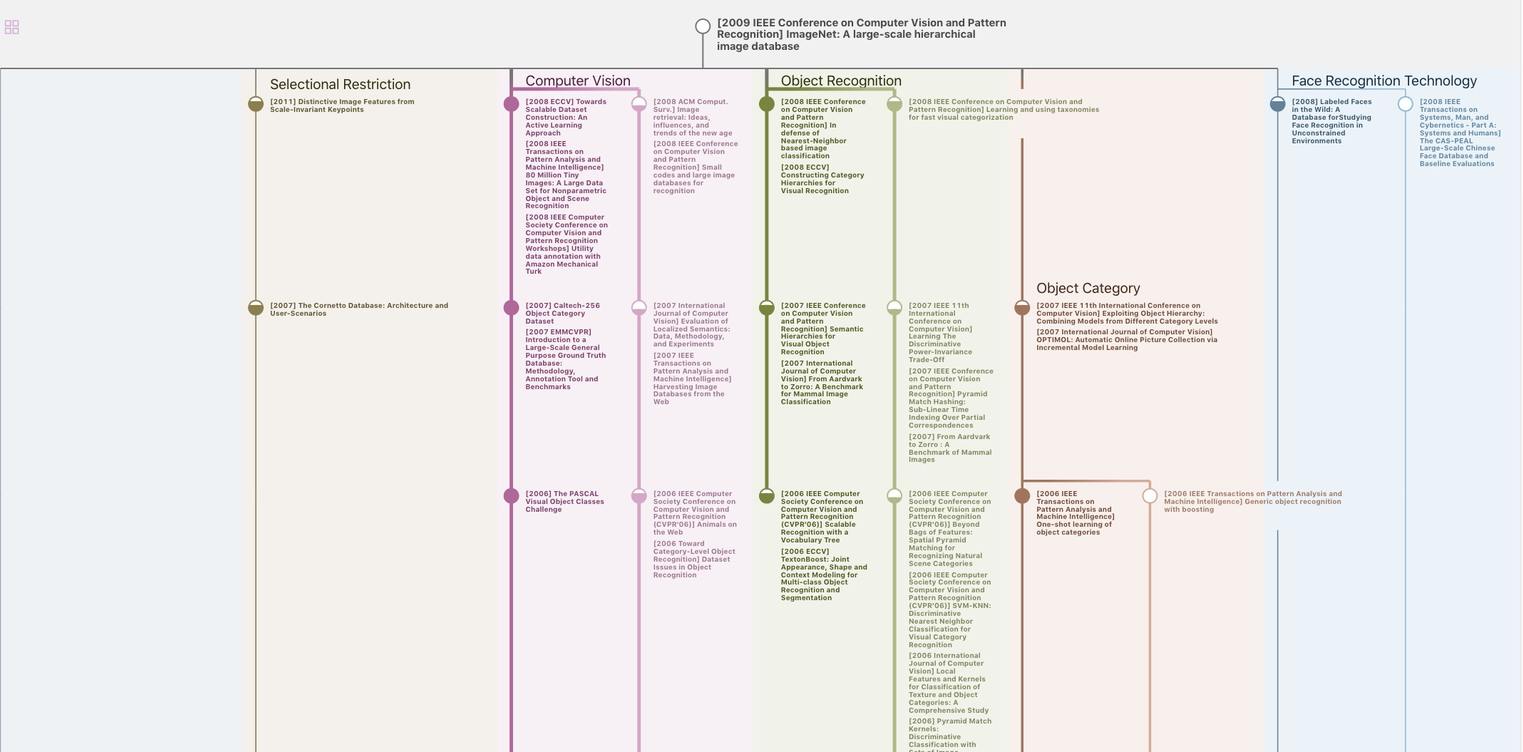
生成溯源树,研究论文发展脉络
Chat Paper
正在生成论文摘要