Data-Driven Approaches for Bead Geometry Prediction Via Melt Pool Monitoring
JOURNAL OF MANUFACTURING SCIENCE AND ENGINEERING-TRANSACTIONS OF THE ASME(2023)
摘要
In the realm of additive manufacturing, the selection of process parameters to avoid over and under deposition entails a time-consuming and resource-intensive trial-and-error approach. Given the distinct characteristics of each part geometry, there is a pressing need for advancing real-time process monitoring and control to ensure consistent and reliable part dimensional accuracy. This research shows that support vector regression (SVR) and convolutional neural network (CNN) models offer a promising solution for real-time process control due to the models' abilities to recognize complex, non-linear patterns with high accuracy. A novel experiment was designed to compare the performance of SVR and CNN models to indirectly detect bead height from a coaxial image of a melt pool from a single-layer, single bead build. The study showed that both SVR and CNN models trained on melt pool data collected from a coaxial optical camera can accurately predict the bead height with a mean absolute percentage error of 3.67% and 3.68%, respectively.
更多查看译文
关键词
additive manufacturing,advanced materials and processing,computer-integrated manufacturing,sensing,monitoring,and diagnostics
AI 理解论文
溯源树
样例
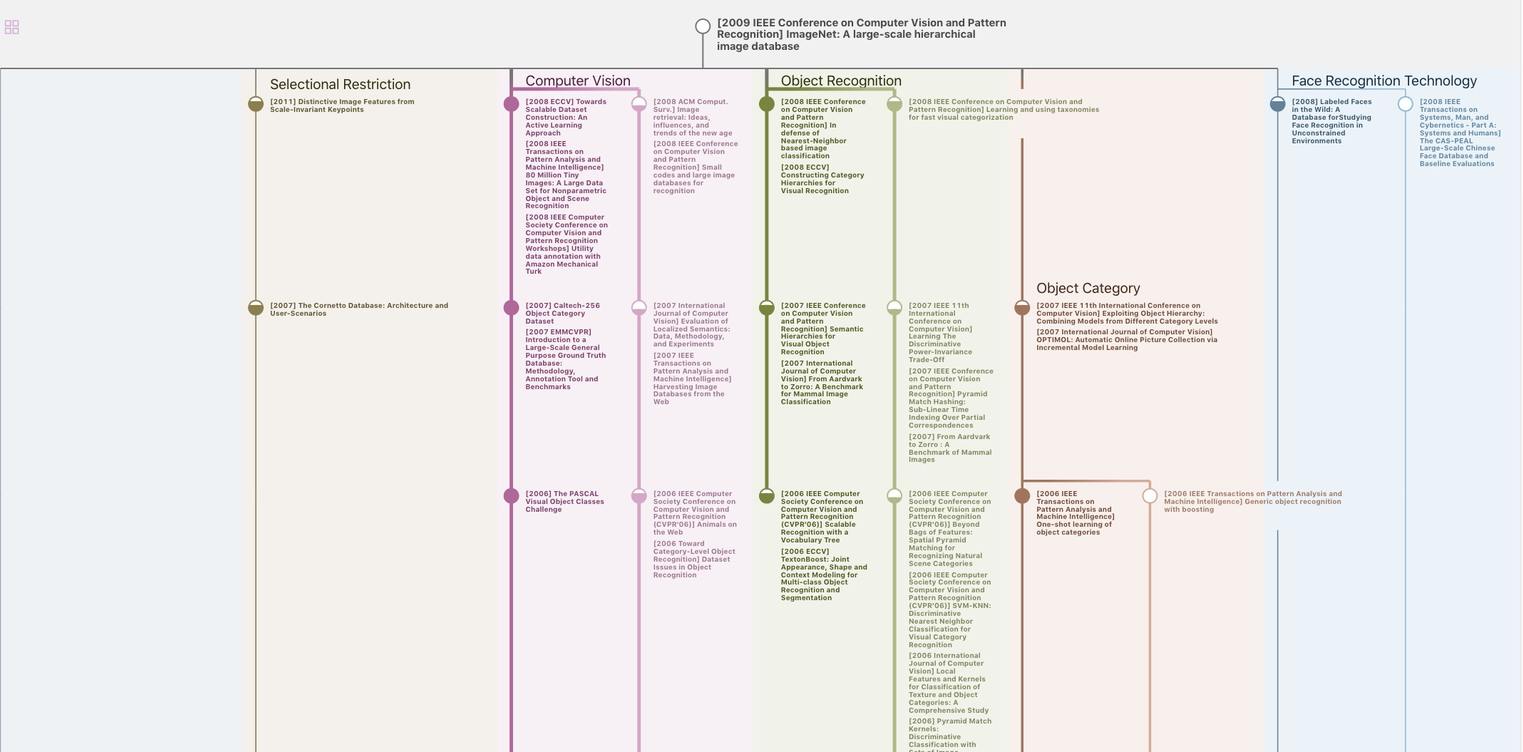
生成溯源树,研究论文发展脉络
Chat Paper
正在生成论文摘要