MarIA and BETO are sexist: evaluating gender bias in large language models for Spanish
LANGUAGE RESOURCES AND EVALUATION(2023)
摘要
The study of bias in language models is a growing area of work, however, both research and resources are focused on English. In this paper, we make a first approach focusing on gender bias in some freely available Spanish language models trained using popular deep neural networks, like BERT or RoBERTa. Some of these models are known for achieving state-of-the-art results on downstream tasks. These promising results have promoted such models’ integration in many real-world applications and production environments, which could be detrimental to people affected for those systems. This work proposes an evaluation framework to identify gender bias in masked language models, with explainability in mind to ease the interpretation of the evaluation results. We have evaluated 20 different models for Spanish, including some of the most popular pretrained ones in the research community. Our findings state that varying levels of gender bias are present across these models.This approach compares the adjectives proposed by the model for a set of templates. We classify the given adjectives into understandable categories and compute two new metrics from model predictions, one based on the internal state (probability) and the other one on the external state (rank). Those metrics are used to reveal biased models according to the given categories and quantify the degree of bias of the models under study.
更多查看译文
关键词
Deep learning, Gender bias, Bias evaluation, Language model, BERT, RoBERTa
AI 理解论文
溯源树
样例
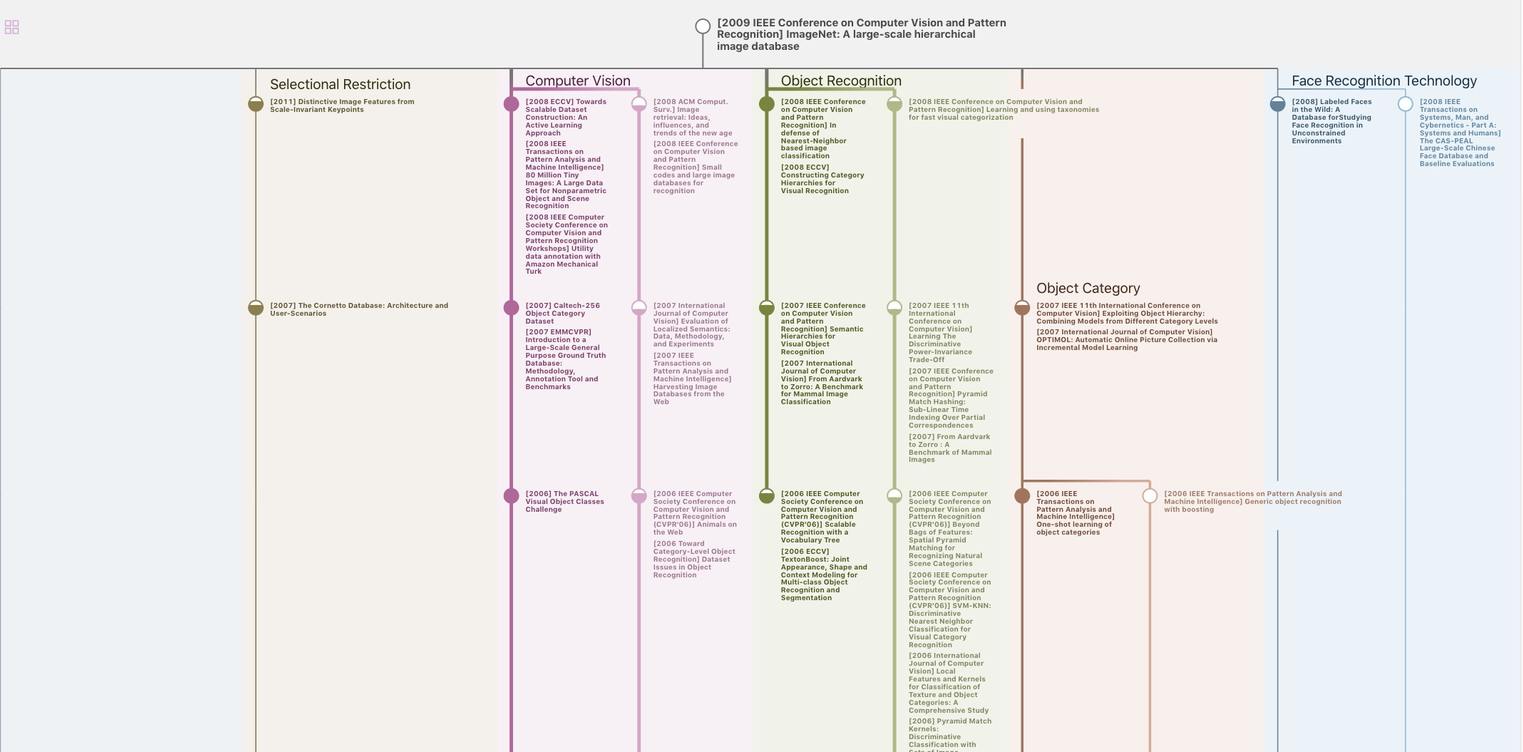
生成溯源树,研究论文发展脉络
Chat Paper
正在生成论文摘要