Uncertainty quantification by gaussian random fields for point-like emissions from satellite observations
INTERNATIONAL JOURNAL FOR UNCERTAINTY QUANTIFICATION(2023)
摘要
We propose a statistical approach to estimate emissions of isolated pointlike sources by NO2 tropospheric column concentrations satellite observations. The approach is data driven; in addition to the satellite measurements it only uses available wind data and a rudimentary model for the NOx chemistry. We construct interpolated fields of the satellite observations using Gaussian random fields, which allows for a more flexible fitting of data than the more standard Gaussian plume regressions. They enable producing uncertainty quantification, even with partly obscured or missing observations. The Gaussian random field surfaces provide continuous surfaces of the satellite observations along which flux integrals are computed to simplify the problem from two-dimensional satellite observations to one-dimensional fluxes. The emission estimates are then obtained by a simple model that combines the flux and chemistry. Extensive uncertainty quantification is implemented at every step of the estimation procedure by using Markov chain Monte Carlo sampling methods. The method is verified by simulated observations and applied to a Copernicus Sentinel-5 Precursor TROPOspheric Monitoring Instrument (TROPOMI) data to estimate industrial nitrogen oxide emissions from the power plants of Belchatow, Poland and Yangluo, Wuhan, China. With Belchatow, we compare the obtained emission rates against reported emissions using annually reported total emissions and available power generation data.
更多查看译文
关键词
gaussian random fields,uncertainty quantification,satellite observations,point-like
AI 理解论文
溯源树
样例
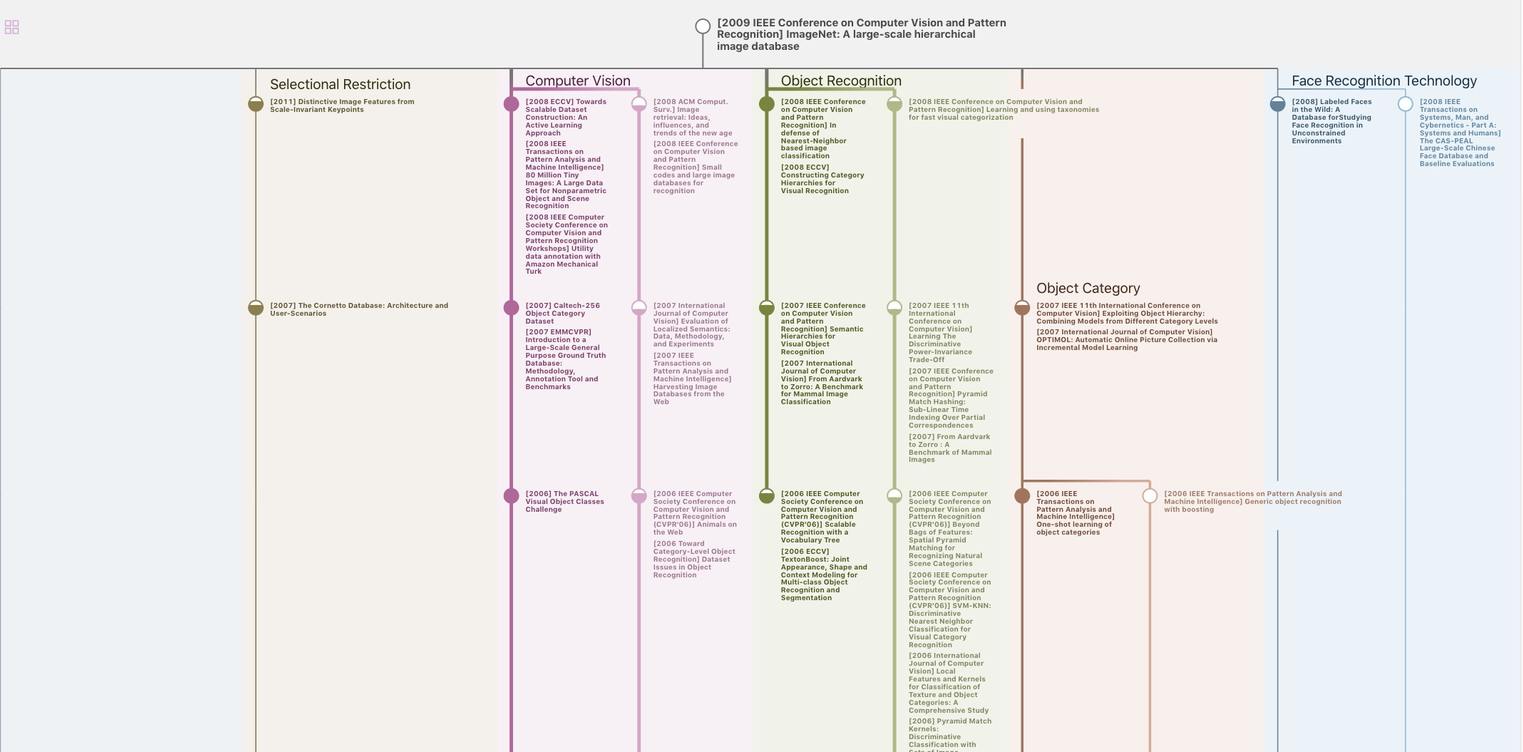
生成溯源树,研究论文发展脉络
Chat Paper
正在生成论文摘要