Building element recognition with MTL-AINet considering view perspectives
OPEN GEOSCIENCES(2023)
摘要
The reconstruction and analysis of building models are crucial for the construction of smart cities. A refined building model can provide a reliable data support for data analysis and intelligent management of smart cities. The colors, textures, and geometric forms of building elements, such as building outlines, doors, windows, roof skylights, roof ridges, and advertisements, are diverse; therefore, it is challenging to accurately identify the various details of buildings. This article proposes the Multi-Task Learning AINet method that considers features such as color, texture, direction, and roll angle for building element recognition. The AINet is used as the basis function; the semantic projection map of color and texture, and direction and roll angle is used for multi-task learning, and the complex building facade is divided into similar semantic patches. Thereafter, the multi-semantic features are combined using hierarchical clustering with a region adjacency graph and the nearest neighbor graph to achieve an accurate recognition of building elements. The experimental results show that the proposed method has a higher accuracy for building detailed edges and can accurately extract detailed elements.
更多查看译文
关键词
multi-view stereo images,building elements,multi-task learning,similar semantic patches,segmentation,clustering
AI 理解论文
溯源树
样例
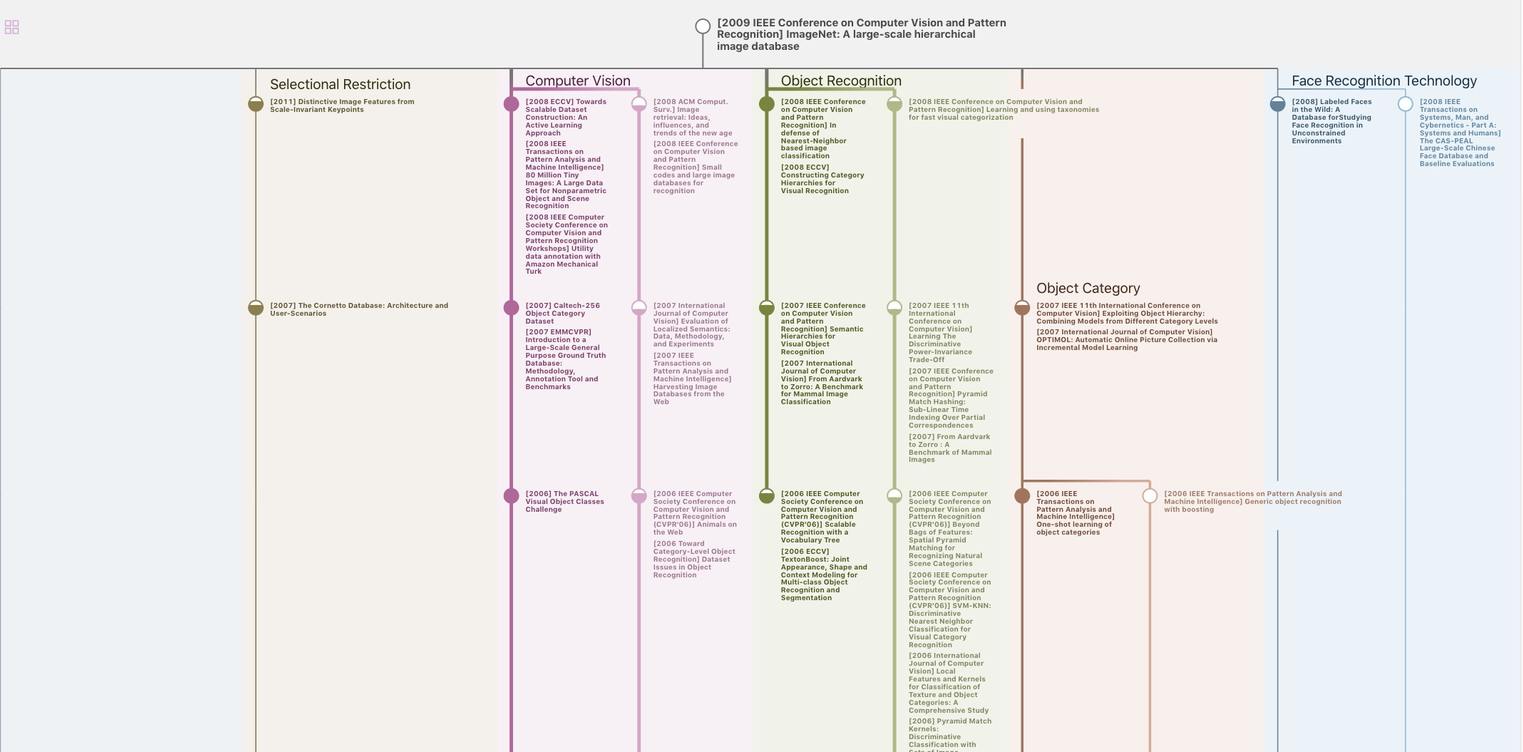
生成溯源树,研究论文发展脉络
Chat Paper
正在生成论文摘要