Direct image classification using an on-chip photonic deep neural network
INTEGRATED OPTICS: DEVICES, MATERIALS, AND TECHNOLOGIES XXVII(2023)
摘要
Artificial neural networks have been a major driving force in a wide variety of technologies from pattern recognition and natural language reprocessing to medical diagnosis. Neural networks can be trained to perform a certain set of tasks and are typically formed by the interconnection of multiple layers of neurons to perform linear and nonlinear computations. Highly reconfigurable clock-based digital signal processors have been the main hardware platform to implement such networks. However, clock frequency and memory access time are two main contributors in limiting the processing speed. Integrated photonics benefits from the large available bandwidth at optical frequencies and low-loss interconnects, and has proved to be a promising candidate to address the challenges of electronic systems. Most of the demonstrated photonic neural networks have been either using bench-top setups, or the partial integration of some of the linear and/or nonlinear computation blocks. A scalable solution that can integrate all computation blocks has been an open problem. Here, we demonstrate the first end-to-end photonic deep neural network (PDNN) for direct image classification. On-chip image formation of the target object using a 2D array of grating couplers and direct processing of the received optical signals through linear (optical) and nonlinear (opto-electronic) computation, enable an end-to-end classification time of 570 ps. Using a uniformly distributed supply light enables scaling the PDNN to networks with more layers of neurons. The PDNN chip is used to demonstrate classification of 2- and 4-class datasets of handwritten letters and the system achieves accuracies higher than 93.8% and 89.8%, respectively.
更多查看译文
关键词
Photonic neural network, Silicon photonics, Integrated photonics, Image classification
AI 理解论文
溯源树
样例
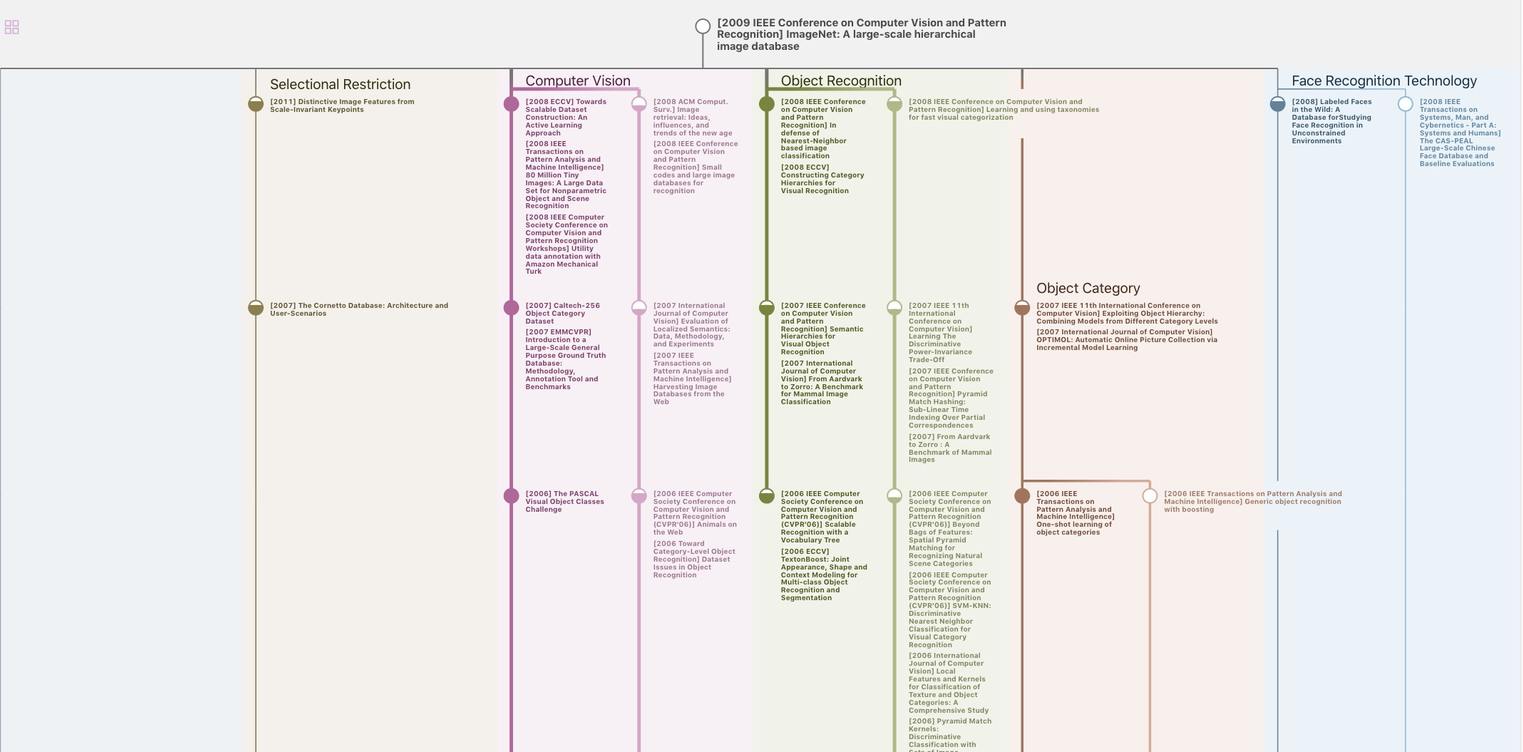
生成溯源树,研究论文发展脉络
Chat Paper
正在生成论文摘要