Graph convolutional networks with learnable spatial weightings for traffic forecasting applications
TRANSPORTMETRICA A-TRANSPORT SCIENCE(2023)
摘要
How to select a suitable spatial weighting scheme for convolutional graph neural networks (ConvGNNs) is challenging. In this study, we propose a ConvGNN, termed learnable graph convolutional (LGC) network, which learns spatial weightings between a road and its k-hop neighbours as learnable parameters in the spatial convolutional operator. A dynamic LGC (DLGC) network is further proposed to learn the dynamics of spatial weightings by explicitly considering the temporal correlations of spatial weightings at different times of the day. A multi-temporal DLGC (MTDLGC) network is developed for forecasting traffic variables in road networks. Results of case study suggest that the MT-DLGC network can achieve higher prediction accuracy than other state-of-the-art baselines. Both LGC and DLGC networks can be used as general spatial weighting schemes for baselines with better forecasting performance than existing spatial weighting schemes, e.g., graph attention. The source code of this study is available publicly at https://github.com/Mayaohong/MTDLGC.
更多查看译文
关键词
Convolutional graph neural networks,spatial weighting scheme,dynamic graph convolutional network,traffic forecasting,transport informatics
AI 理解论文
溯源树
样例
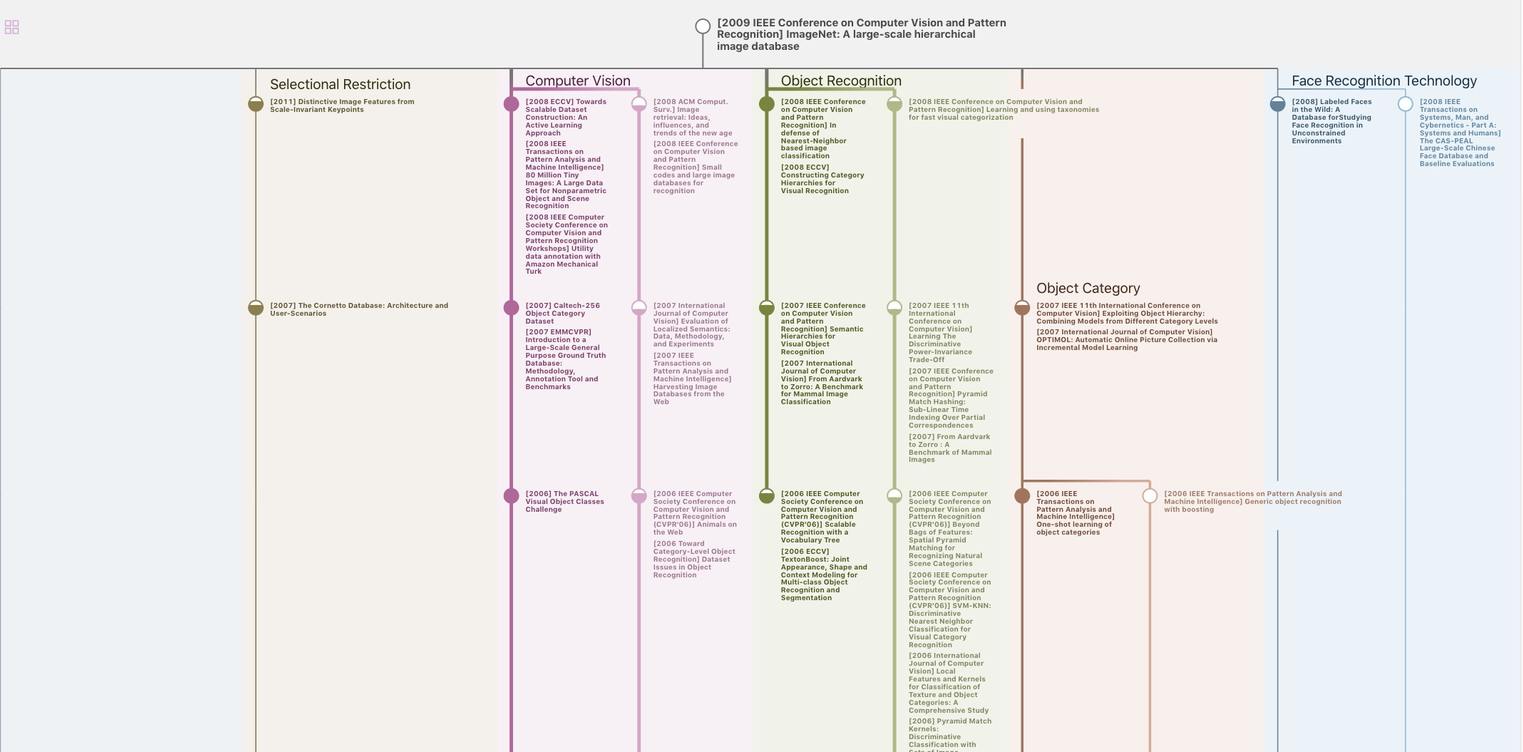
生成溯源树,研究论文发展脉络
Chat Paper
正在生成论文摘要