Large stochastic volatility in mean VARs
JOURNAL OF ECONOMETRICS(2023)
摘要
Bayesian vector autoregressions with stochastic volatility in both the conditional mean and variance (SVMVARs) are widely used for studying the macroeconomic effects of uncertainty. Despite their popularity, intensive computational demands when estimating such models has constrained researchers to specifying a small number of latent volatilities, and made out-of-sample forecasting exercises impractical. In this paper, we propose an efficient Markov chain Monte Carlo (MCMC) algorithm that facilitates timely posterior and predictive inference with large SVMVARs. In a simulation exercise, we show that the new algorithm is significantly faster than the state-of-the-art particle Gibbs with ancestor sampling algorithm, and exhibits superior mixing properties. In two applications, we show that large SVMVARs are generally useful for structural analysis and out-of-sample forecasting, and are especially useful in periods of high uncertainty such as the Great Recession and the COVID-19 pandemic. & COPY; 2023 Elsevier B.V. All rights reserved.
更多查看译文
关键词
Bayesian VARs,Macroeconomic forecasting,Stochastic volatility in mean,State space models,Uncertainty
AI 理解论文
溯源树
样例
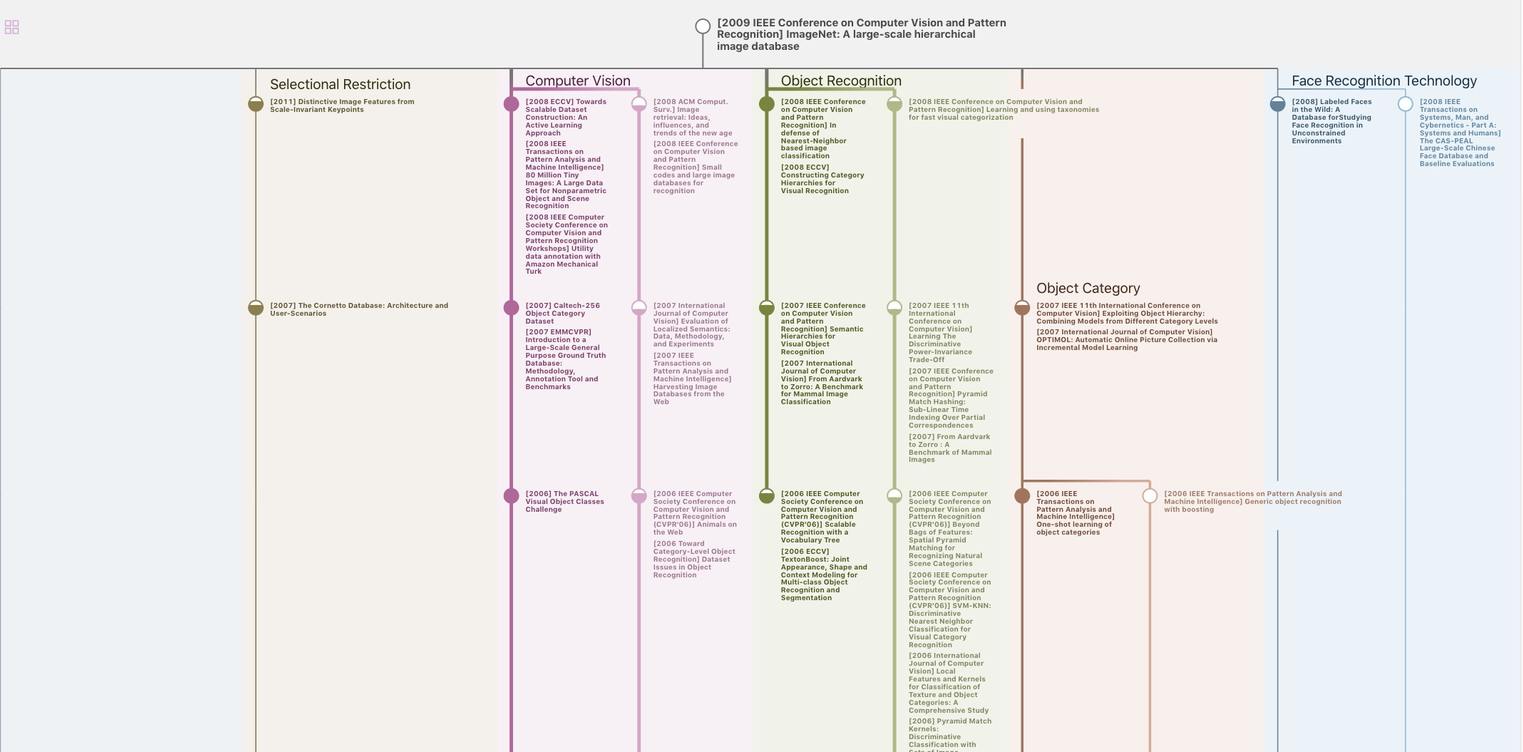
生成溯源树,研究论文发展脉络
Chat Paper
正在生成论文摘要