Harnessing Nature-Inspired Soft Computing for Reinforced Soil Bearing Capacity Prediction: A Neuro-nomograph Approach for Efficient Design
INTERNATIONAL JOURNAL OF GEOSYNTHETICS AND GROUND ENGINEERING(2023)
Abstract
The bearing capacity of soil in general is considered a key parameter in the field of geotechnical engineering, requiring field investigations and extensive testing to eliminate design errors. Reinforced sand beds, however, present unique complexities for conventional computational techniques, as these methods may fall short in fully capturing the interaction between parameters that control ultimate bearing capacity. Consequently, nature-inspired soft computing (SC) techniques, inspired by the resilience and adaptability inherent in natural systems, have been utilized and evaluated in simulating and predicting the reinforced soil bearing capacity in geotechnical engineering. These include support vectors machines (SVM), ensemble tree (ET), Gaussian’s process regressions (GPR), regression trees (RT), and artificial neural network (ANN). A thorough evaluation of SC techniques was executed for their computational efficiency and prediction accuracy. The hypermeter values of all SC techniques were optimized using Bayesian optimization with the goal of reducing prediction error. The results showed that ANN-SC technique outperformed the other techniques based on three performance measures: mean absolute errors (MAE), coefficients of determination ( R 2 ), and root mean square errors (RMSE). Furthermore, normalized importance analysis was conducted using the best-performed model (ANN), and the findings demonstrated that the soil average particle size/reinforcement width (D 50 /Aw) had the highest relative importance on the ANN-predicted bearing capacity, followed by friction angle and depth of first reinforcement layer. The python code (using PyNomo library) was employed to develop a nomograph to facilitate the application of the ANN model findings. This neuro-nomograph uses the weights and biases from the optimal ANN model to offer a simplified tool to predict reinforced soil bearing capacity. Furthermore, the performance of this novel neuro-nomograph was validated using physical bearing capacity test results. The comparison showed a coefficient of variation (COV) of 0.13 and a mean ratio of test to predicted bearing capacities (UBC test /UBC pred ) of 1.11, which suggests the minimal scatter and, thus, confirm the high precision of the developed neuro-nomograph predictions of the reinforced soil bearing capacities.
MoreTranslated text
Key words
soft computing,soil,nature-inspired,neuro-nomograph
AI Read Science
Must-Reading Tree
Example
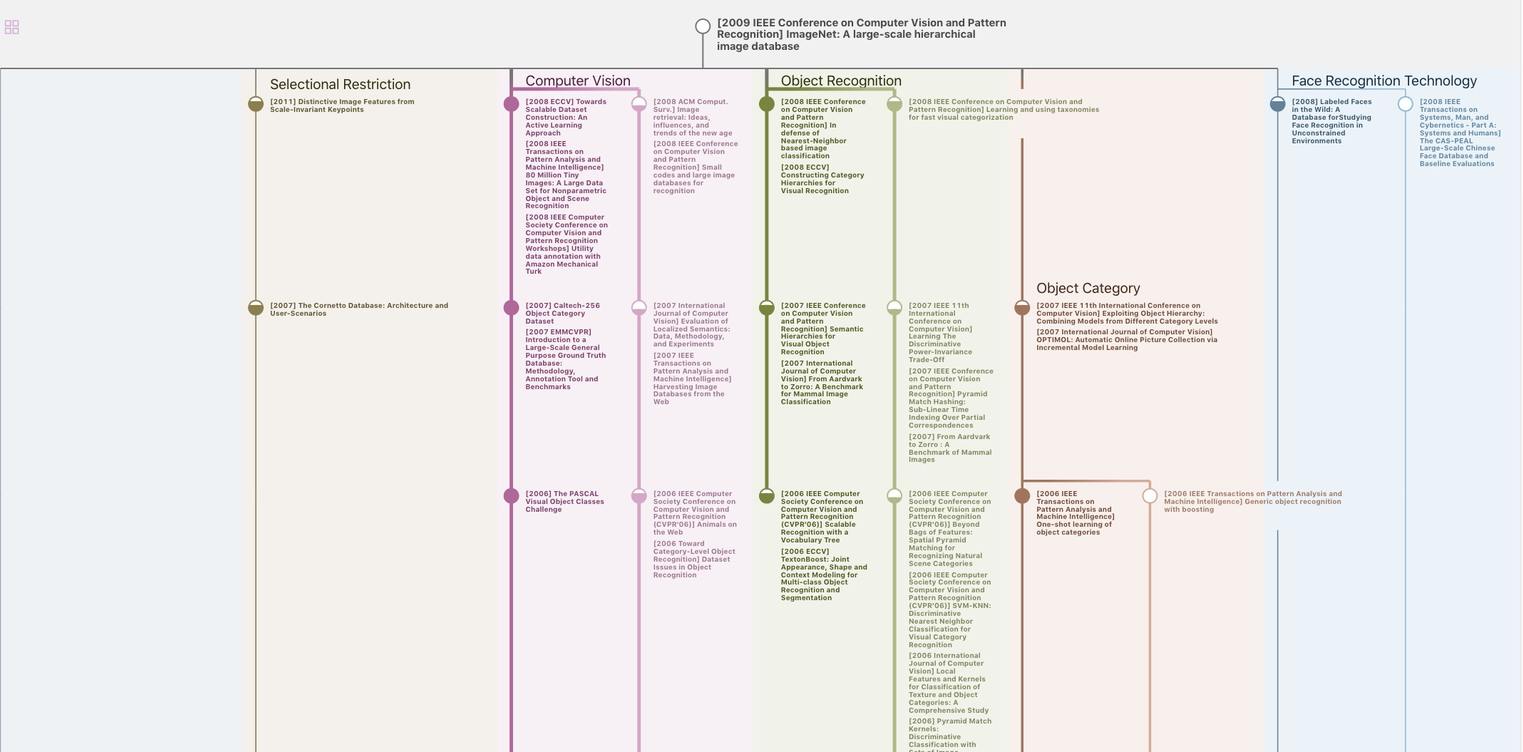
Generate MRT to find the research sequence of this paper
Chat Paper
Summary is being generated by the instructions you defined