Predicting the lateral displacement of tall buildings using an LSTM-based deep learning approach
WIND AND STRUCTURES(2023)
摘要
Structural health monitoring is used to ensure the well-being of civil structures by detecting damage and estimating deterioration. Wind flow applies external loads to high-rise buildings, with the horizontal force component of the wind causing structural displacements in high-rise buildings. This study proposes a deep learning-based predictive model for measuring lateral displacement response in high-rise buildings. The proposed long short-term memory model functions as a sequence generator to generate displacements on building floors depending on the displacement statistics collected on the top floor. The model was trained with wind-induced displacement data for the top floor of a high-rise building as input. The outcomes demonstrate that the model can forecast wind-induced displacement on the remaining floors of a building. Further, displacement was predicted for each floor of the high-rise buildings at wind flow angles of 0 & DEG; and 45 & DEG;. The proposed model accurately predicted a high-rise building model's story drift and lateral displacement. The outcomes of this proposed work are anticipated to serve as a guide for assessing the overall lateral displacement of high-rise buildings.
更多查看译文
关键词
high-rise buildings, long short-term memory, recurrent neural network, structural health monitoring, wind-induced displacement
AI 理解论文
溯源树
样例
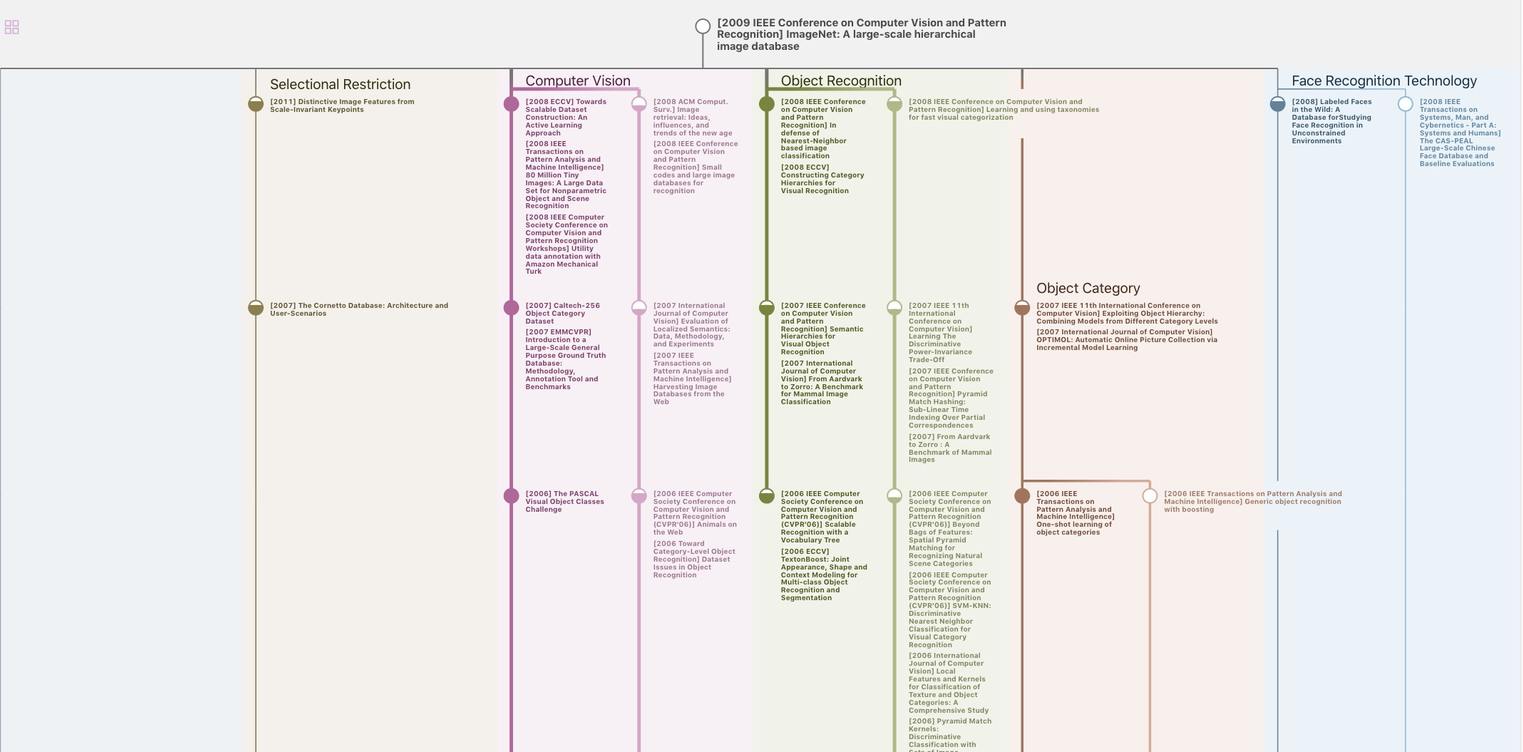
生成溯源树,研究论文发展脉络
Chat Paper
正在生成论文摘要