A novel fusion maximum correntropy Kalman/UFIR filter for state estimation with uncertain non-Gaussian noise statistics
MEASUREMENT(2023)
摘要
Despite the higher estimation accuracy of the maximum correntropy Kalman filter (MCKF) algorithm in system with non-Gaussian noise, its estimation performance will decrease when the noise source changes due to disturbances from external uncertainties. Fortunately, the unbiased finite impulse response predictor (UFIR) can overcome the MCKF problems by automatically ignoring the noise related to both the process and measurements. Meanwhile, the MCKF could resolve the UFIR problems, such as non-optimal estimation performance and risk of dead zones. Therefore, a novel fusion maximum correntropy Kalman/UFIR filter is proposed to achieve a more advantageous estimation effect, in which specific probability weights are assigned to the two filters to implement the fusion of two filters. The simulation results demonstrate the superiority of the presented fusion filter algorithm when compared to the fusion Kalman/UFIR filter regarding the estimation performance under the non-Gaussian noise system.
更多查看译文
关键词
maximum correntropy kalman/ufir,state estimation,kalman/ufir filter,novel fusion,non-gaussian
AI 理解论文
溯源树
样例
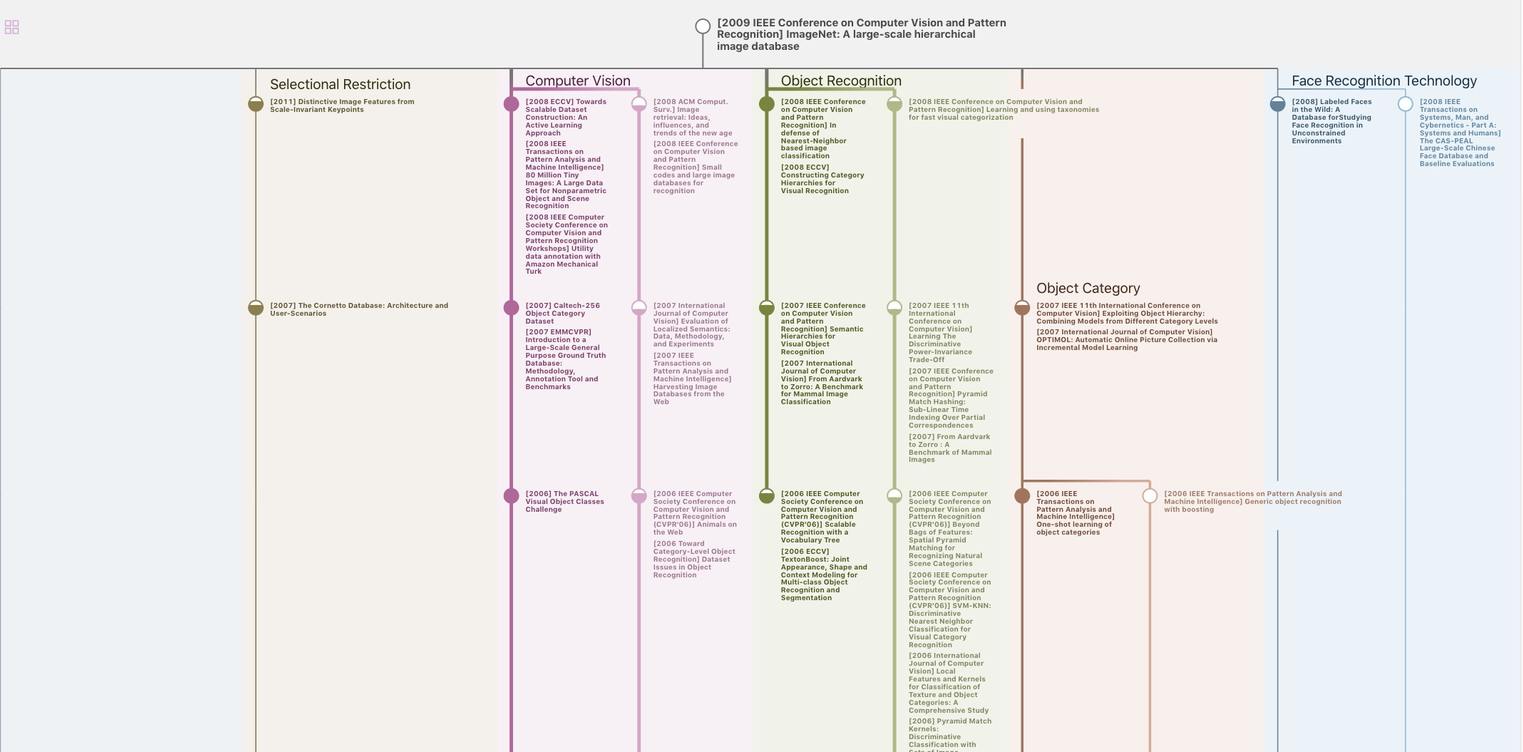
生成溯源树,研究论文发展脉络
Chat Paper
正在生成论文摘要