Explaining any black box model using real data
FRONTIERS IN COMPUTER SCIENCE(2023)
摘要
In recent years the use of complex machine learning has increased drastically. These complex black box models trade interpretability for accuracy. The lack of interpretability is troubling for, e.g., socially sensitive, safety-critical, or knowledge extraction applications. In this paper, we propose a new explanation method, SLISE, for interpreting predictions from black box models. SLISE can be used with any black box model (model-agnostic), does not require any modifications to the black box model (post-hoc), and explains individual predictions (local). We evaluate our method using real-world datasets and compare it against other model-agnostic, local explanation methods. Our approach solves shortcomings in other related explanation methods by only using existing data instead of sampling new, artificial data. The method also generates more generalizable explanations and is usable without modification across various data domains.
更多查看译文
关键词
XAI (explainable artificial intelligence), model-agnostic explanation, interpretable machine learning, local explanation, explanations, interpretability, HCI (human-computer interaction)
AI 理解论文
溯源树
样例
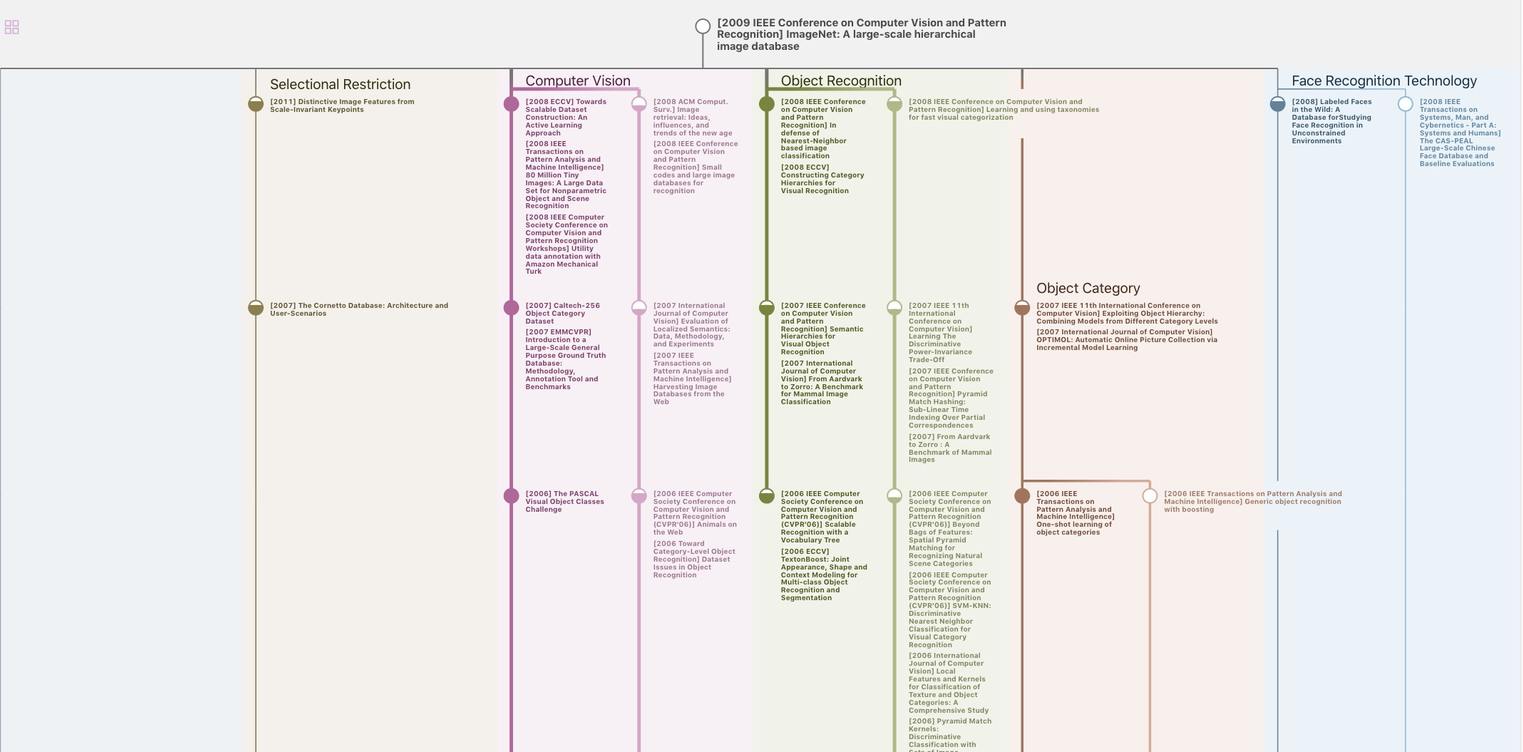
生成溯源树,研究论文发展脉络
Chat Paper
正在生成论文摘要