Spatio-temporal correlation for simultaneous ultra-short-term wind speed prediction at multiple locations
ENERGY(2023)
摘要
Wind, as a fluid, has continuity in both space and time. Coupling spatial and temporal information to build prediction models can improve wind speed prediction accuracy. This paper proposes a method that predicts wind speed at multiple locations using both spatial and temporal data. Three deep learning models are introduced: Convolutional Residual Spatial-Temporal Long Short-Term Memory neural network (CoReSTL), Convolutional Spatial-Temporal-3D neural network (CoST-3), and Convolutional Spatial-Temporal Long Short-Term Memory neural network (CoST-L). These models combine Convolutional Long Short-Term Memory (ConvLSTM), Residual Network (ResNet), and 1 x 1 3D convolution to extract spatial and temporal correlations between multi-site wind speeds. The spatio-temporal prediction of wind fields under two terrains was carried out to screen out neural network models with higher accuracy. The results show that CoReSTL, CoST-3, and CoST-L all achieved better prediction results. However, the performance of the CoReSTL model was better than that of CoST-3 and CoST-L, with stronger applicability in complex real terrain.
更多查看译文
关键词
wind,prediction,correlation,spatio-temporal,ultra-short-term
AI 理解论文
溯源树
样例
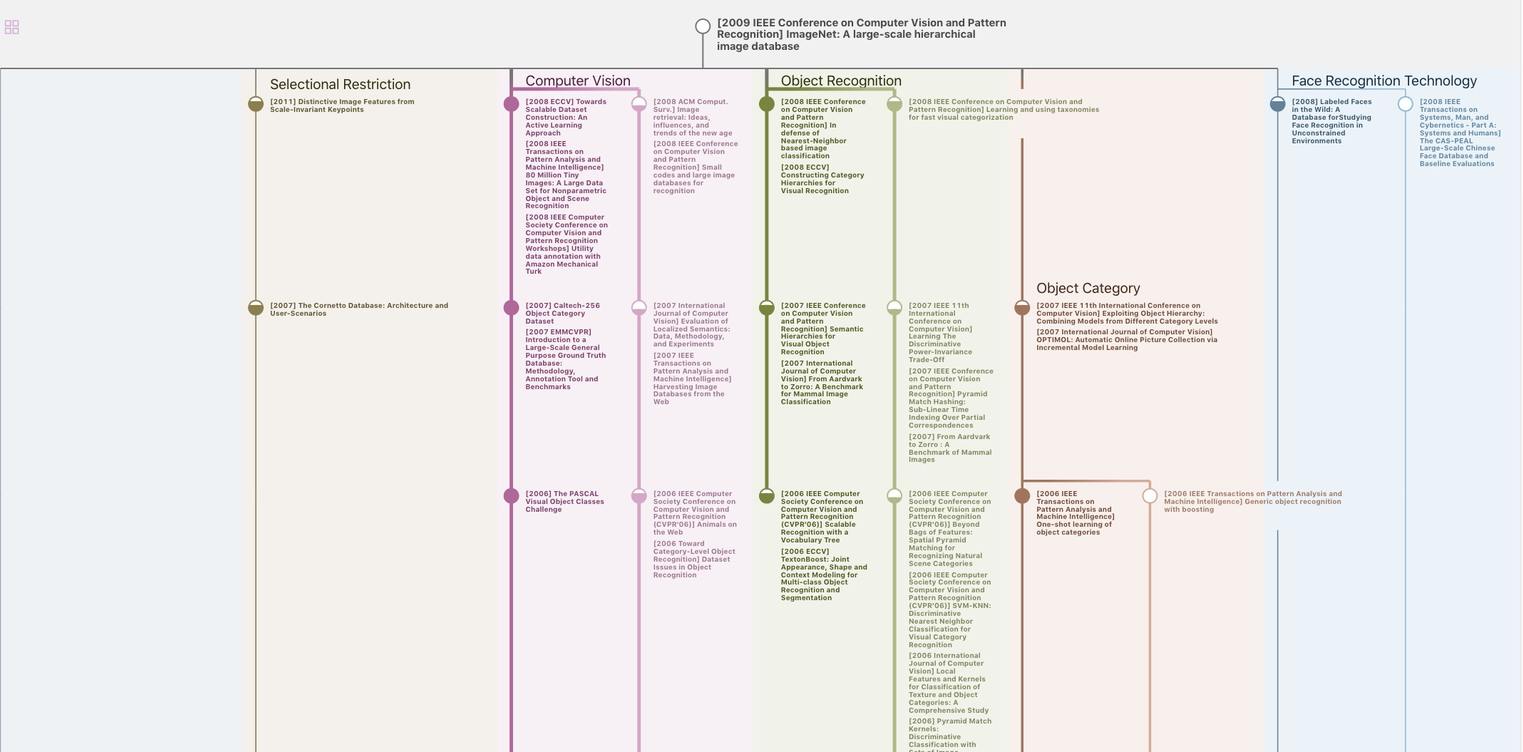
生成溯源树,研究论文发展脉络
Chat Paper
正在生成论文摘要