A New Spatio-Temporal Selection Method for Estimating Upwelling Medium-Wave Radiation
REMOTE SENSING(2023)
摘要
Accurate estimates of the unperturbed state of upwelling radiation from the earth's surface are vital to the detection and classification of anomalous radiation values. Determining radiative anomalies in the landscape is critical for isolating change, a key application being wildfire detection, which is reliant upon knowledge of a location's radiation budget sans fire. Most techniques for deriving the unperturbed background state of a location use that location's spatial context, that is, the pixels immediately surrounding the target. Spatial contextual estimation can be subject to error due to occlusion of the pixel's spatial context and issues such as land cover heterogeneity. This paper proposes a new method of deriving background radiation levels by decoupling the set of prediction pixels used for estimation from the target location in a Spatio-Temporal Selection (STS) process. The process selects training pixels for predictive purposes from a target-centred search area based on their similarity with the target pixel in terms of brightness temperature over a prescribed time period. The proposed STS process was applied to images from the AHI-8 geostationary sensor centred over the Asia-Pacific, and comparisons were made to both brightness temperature estimates from the spatial context and to sensor measurements. This comparison showed that the STS method provided between 10-40% reduction in estimation error over the commonly utilised contextual estimator; in addition, the STS method increased the availability of estimates in comparison to the spatial context by between 12-31%. Image reconstruction using the method resulted in high-fidelity reproductions of the examined landscape, with standing geographic features and areas experiencing thermal anomalies readily identifiable on the resulting images.
更多查看译文
关键词
radiation,selection,spatio-temporal,medium-wave
AI 理解论文
溯源树
样例
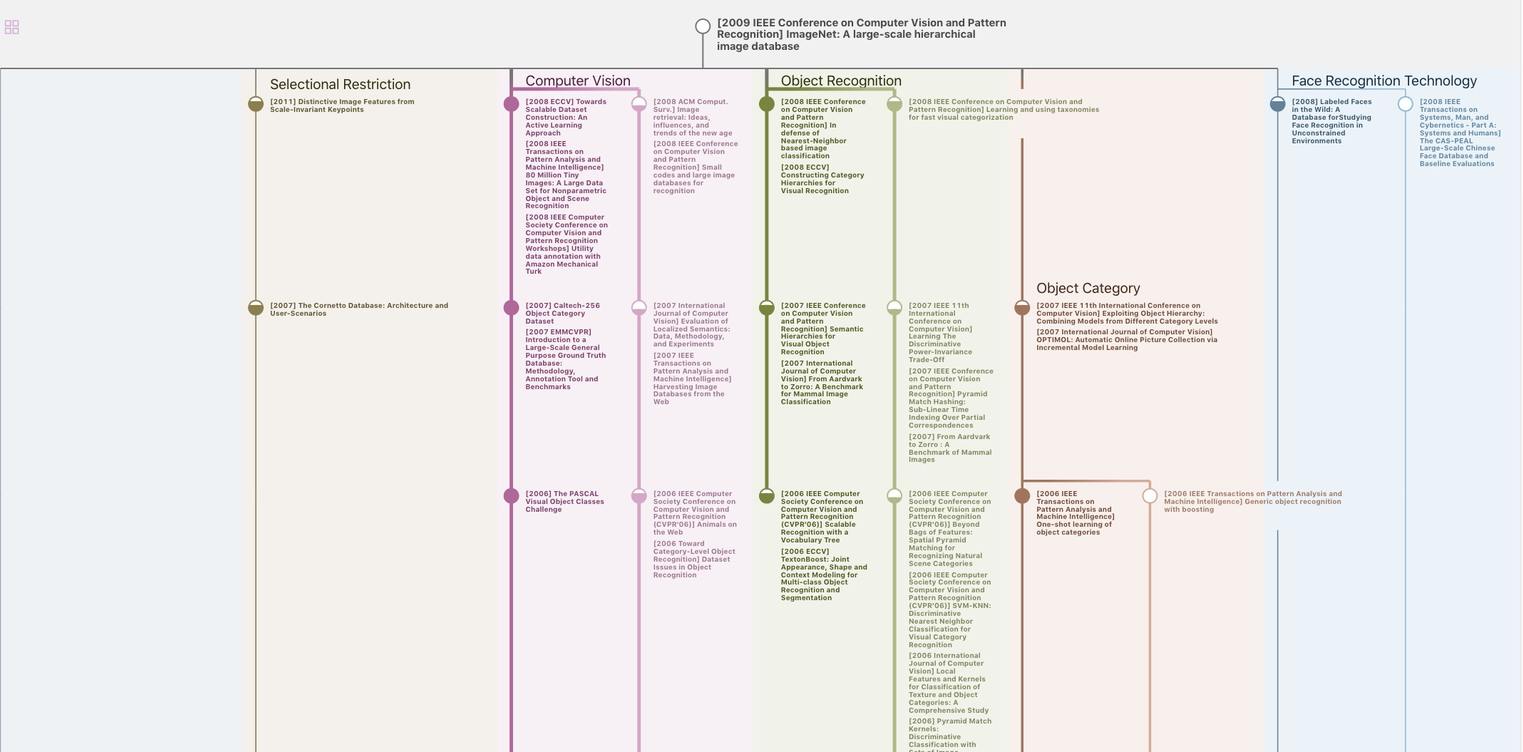
生成溯源树,研究论文发展脉络
Chat Paper
正在生成论文摘要