Hyperspectral Anomaly Detection Using Spatial-Spectral-Based Union Dictionary and Improved Saliency Weight
REMOTE SENSING(2023)
摘要
Hyperspectral anomaly detection (HAD), which is widely used in military and civilian fields, aims to detect the pixels with large spectral deviation from the background. Recently, collaborative representation using union dictionary (CRUD) was proved to be effective for achieving HAD. However, the existing CRUD detectors generally only use the spatial or spectral information to construct the union dictionary (UD), which possibly causes a suboptimal performance and may be hard to use in actual scenarios. Additionally, the anomalies are treated as salient relative to the background in a hyperspectral image (HSI). In this article, a HAD method using spatial-spectral-based UD and improved saliency weight (SSUD-ISW) is proposed. To construct robust UD for each testing pixel, a spatial-based detector, a spectral-based detector and superpixel segmentation are jointly considered to yield the background set and anomaly set, which provides pure and representative pixels to form a robust UD. Differently from the conventional operation that uses the dual windows to construct the background dictionary in the local region and employs the RX detector to construct the anomaly dictionary in a global scope, we developed a robust UD construction strategy in a nonglobal range by sifting the pixels closest to the testing pixel from the background set and anomaly set to form the UD. With a preconstructed UD, a CRUD is performed, and the product of the anomaly dictionary and corresponding representation coefficient is explored to yield the response map. Moreover, an improved saliency weight is proposed to fully mine the saliency characteristic of the anomalies. To further improve the performance, the response map and saliency weight are combined with a nonlinear fusion strategy. Extensive experiments performed on five datasets (i.e., Salinas, Texas Coast, Gainesville, San Diego and SpecTIR datasets) demonstrate that the proposed SSUD-ISW detector achieves the satisfactory AUC(df) values (i.e., 0.9988, 0.9986, 0.9939, 0.9945 and 0.9997), as compared to the comparative detectors whose best AUC(df) values are 0.9938, 0.9956, 0.9833, 0.9919 and 0.9991.
更多查看译文
关键词
anomaly detection,collaborative representation,union dictionary,saliency weight
AI 理解论文
溯源树
样例
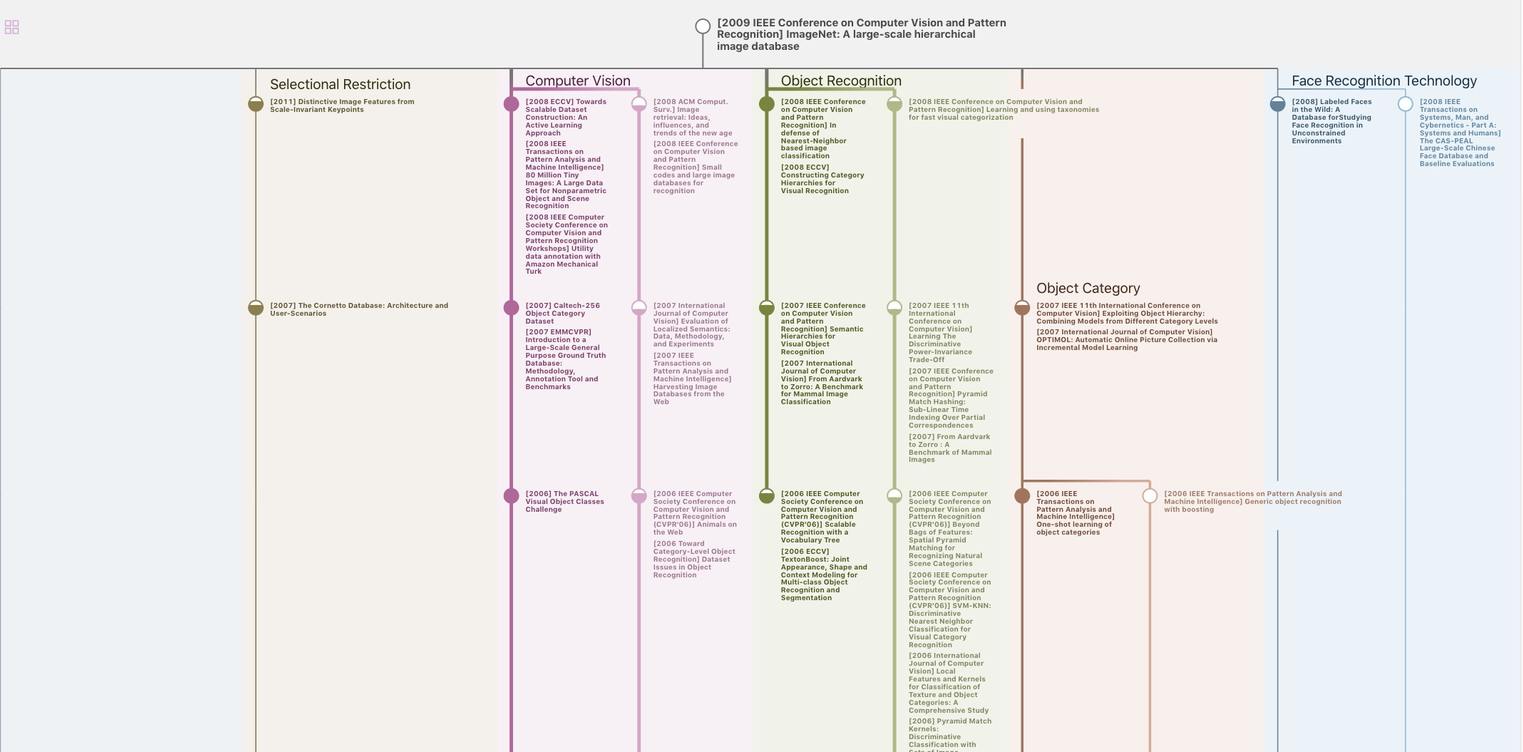
生成溯源树,研究论文发展脉络
Chat Paper
正在生成论文摘要