Independent Design and Optimization of Control-Learning Performance for a Nonlinear Repetitive-Control System
IEEE-ASME TRANSACTIONS ON MECHATRONICS(2023)
摘要
Repetitive control involves two different behaviors: continuous control in a period and discrete learning between two periods, which together determine the fast and precise tracking control. Using a 2-D method, previous methods could only preferentially design the two behaviors rather than independently, because of the coupling of control and learning in the conventional modified repetitive control, which limits further improvement of tracking performance. This article presents a generalized modified repetitive control structure that separates control and learning and allows them to be designed independently using a 2-D method. Using a Takagi Sugeno (T-S) fuzzy model and a parallel distribution compensation scheme, a linear-matrix-inequality (LMI)-based condition is derived to ensure the stability of a nonlinear repetitive-control system. Three adjustable parameters in the LMIs are used to tune the weights of control and learning. After that, dual indexes are devised to evaluate the control-learning performance, respectively. Thus, the regulation of the control-learning behavior is transformed into a standard multiobjective optimization problem. The nondominated sorting genetic algorithm-II is used to solve the problem. Finally, numerical simulations are performed to illustrate the process of independent regulation of control and learning, and experimental comparisons with related approaches are performed to show the validity and superiority.
更多查看译文
关键词
2-D model,control and learning,dual indexes,generalized modified repetitive control (GMRC),independent design and optimization,repetitive control
AI 理解论文
溯源树
样例
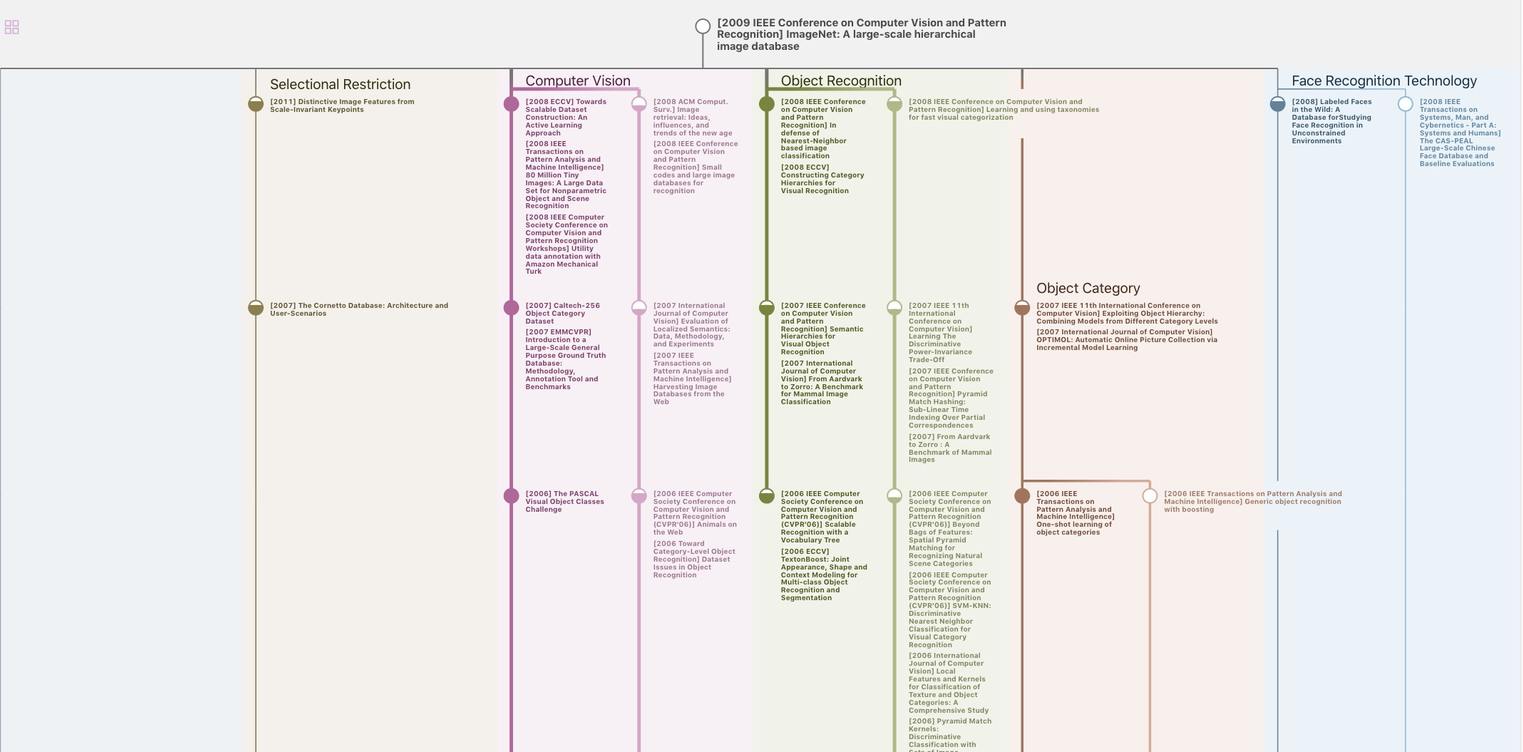
生成溯源树,研究论文发展脉络
Chat Paper
正在生成论文摘要