Unsupervised Feature Extraction for Various Computer-Aided Diagnosis Using Multiple Convolutional Autoencoders and 2.5-Dimensional Local Image Analysis
APPLIED SCIENCES-BASEL(2023)
摘要
Featured Application Providing effective local image features for developing a computer-aided diagnosis system without the burden of collecting disease datasets and annotating its lesion area. There are growing expectations for AI computer-aided diagnosis: computer-aided diagnosis (CAD) systems can be used to improve the accuracy of diagnostic imaging. However, it is not easy to collect large amounts of disease medical image data with lesion area annotations for the supervised learning of CAD systems. This study proposes an unsupervised local image feature extraction method running without such disease medical image datasets. Local image features are one of the key determinants of system performance. The proposed method requires only a normal image dataset that does not include lesions and can be collected easier than a disease dataset. The unsupervised features are extracted by applying multiple convolutional autoencoders to analyze various 2.5-dimensional images. The proposed method is evaluated by two kinds of problems: the detection of cerebral aneurysms in head MRA images and the detection of lung nodules in chest CT images. In both cases, the performance is high, showing an AUC of more than 0.96. These results show that the proposed method can automatically learn features that are useful for lesion recognition from lesion-free normal data, regardless of the type of image or lesion.
更多查看译文
关键词
computer-aided computer-aided diagnosis,feature extraction,multiple convolutional autoencoders
AI 理解论文
溯源树
样例
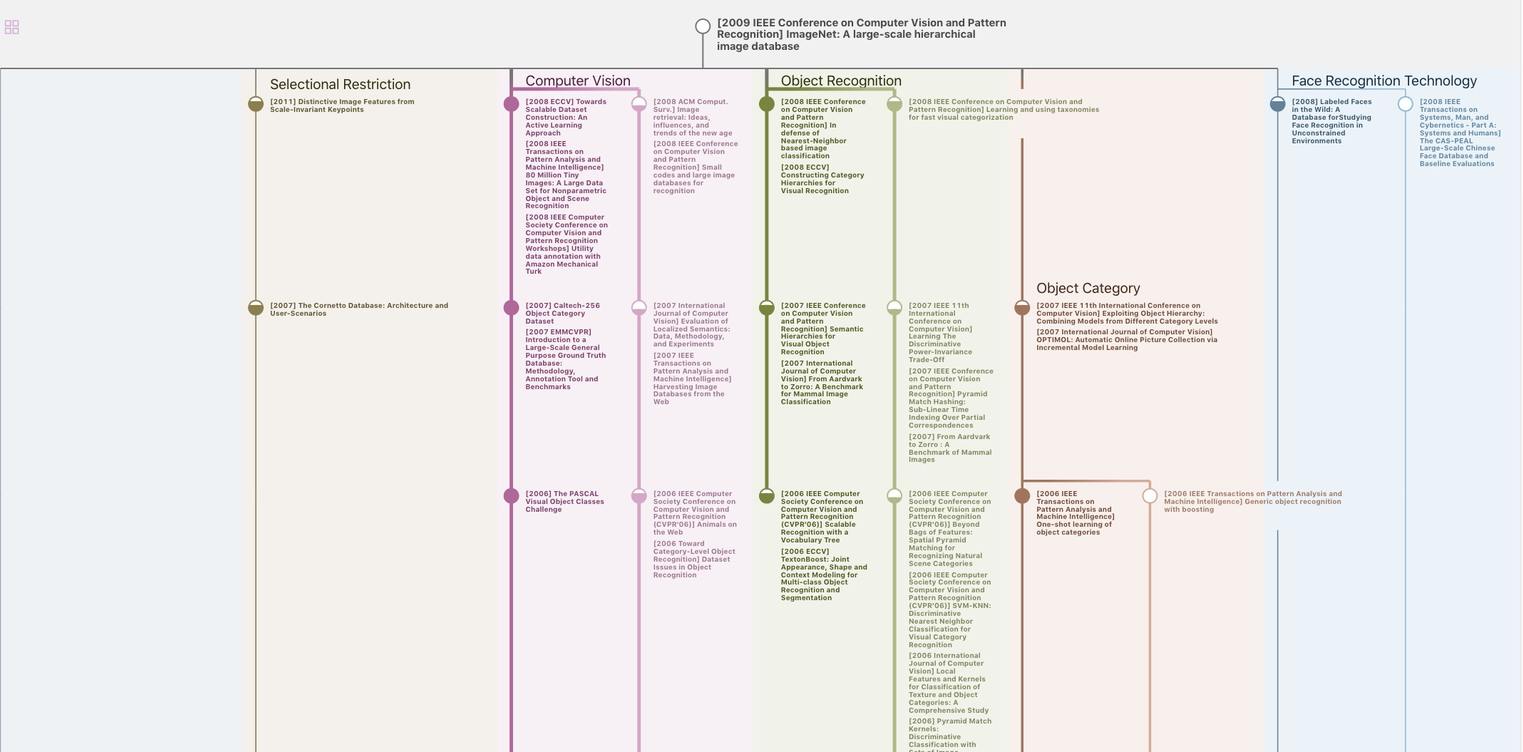
生成溯源树,研究论文发展脉络
Chat Paper
正在生成论文摘要